CoCo: Coherence-Enhanced Machine-Generated Text Detection Under Data Limitation With Contrastive Learning
arxiv(2022)
摘要
Machine-Generated Text (MGT) detection, a task that discriminates MGT from Human-Written Text (HWT), plays a crucial role in preventing misuse of text generative models, which excel in mimicking human writing style recently. Latest proposed detectors usually take coarse text sequence as input and output some good results by fine-tune pretrained models with standard cross-entropy loss. However, these methods fail to consider the linguistic aspect of text (e.g., coherence) and sentence-level structures. Moreover, they lack the ability to handle the low-resource problem which could often happen in practice considering the enormous amount of textual data online. In this paper, we present a coherence-based contrastive learning model named CoCo to detect the possible MGT under low-resource scenario. Inspired by the distinctiveness and permanence properties of linguistic feature, we represent text as a coherence graph to capture its entity consistency, which is further encoded by the pretrained model and graph neural network. To tackle the challenges of data limitations, we employ a contrastive learning framework and propose an improved contrastive loss for making full use of hard negative samples in training stage. The experiment results on two public datasets prove our approach outperforms the state-of-art methods significantly.
更多查看译文
关键词
text detection,contrastive learning,coco,coherence-enhanced,machine-generated
AI 理解论文
溯源树
样例
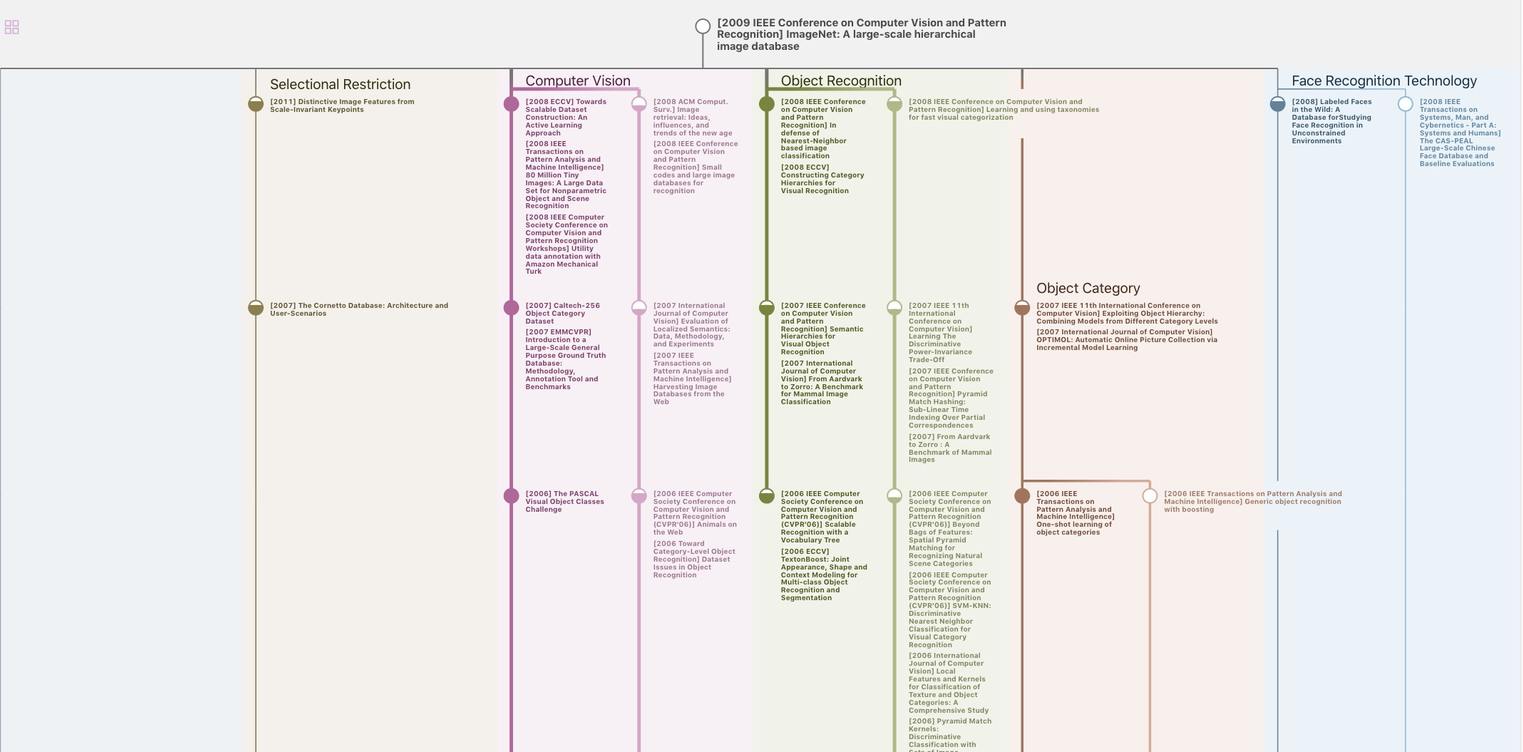
生成溯源树,研究论文发展脉络
Chat Paper
正在生成论文摘要