Socratic Pretraining: Question-Driven Pretraining for Controllable Summarization
arxiv(2022)
摘要
In long document controllable summarization, where labeled data is scarce, pretrained models struggle to adapt to the task and effectively respond to user queries. In this paper, we introduce Socratic pretraining, a question-driven, unsupervised pretraining objective specifically designed to improve controllability in summarization tasks. By training a model to generate and answer relevant questions in a given context, Socratic pretraining enables the model to more effectively adhere to user-provided queries and identify relevant content to be summarized. We demonstrate the effectiveness of this approach through extensive experimentation on two summarization domains, short stories and dialogue, and multiple control strategies: keywords, questions, and factoid QA pairs. Our pretraining method relies only on unlabeled documents and a question generation system and outperforms pre-finetuning approaches that use additional supervised data. Furthermore, our results show that Socratic pretraining cuts task-specific labeled data requirements in half, is more faithful to user-provided queries, and achieves state-of-the-art performance on QMSum and SQuALITY.
更多查看译文
关键词
socratic pretraining,controllable
AI 理解论文
溯源树
样例
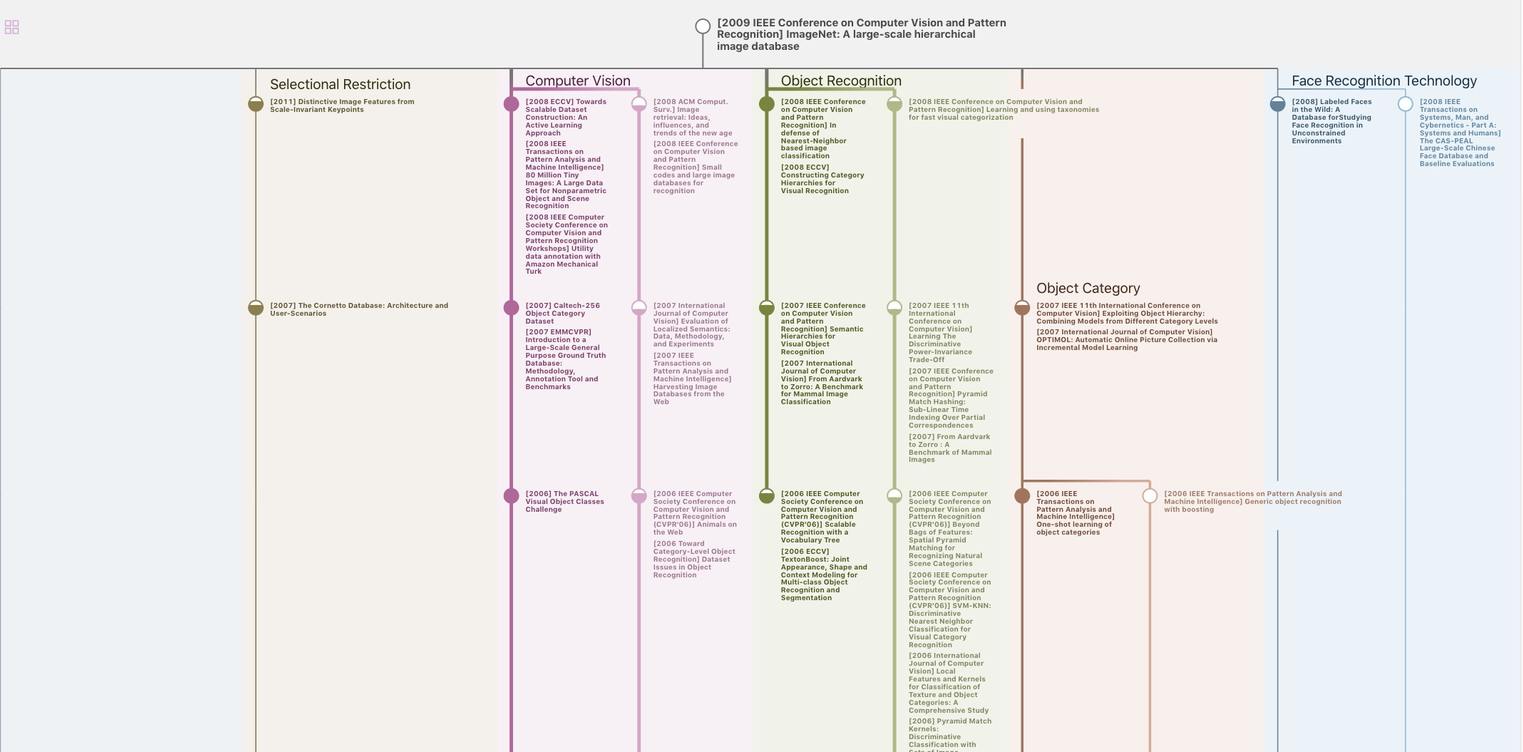
生成溯源树,研究论文发展脉络
Chat Paper
正在生成论文摘要