Self-Supervised Fine-Grained Cycle-Separation Network (FSCN) for Visual-Audio Separation
IEEE TRANSACTIONS ON MULTIMEDIA(2023)
摘要
Audio mixture separation is still challenging due to heavy overlaps and interactions. To correctly separate audio mixtures, we propose a novel self-supervised Fine-grained Cycle-Separation Network (FCSN) for vision-guided audio mixture separation. In the proposed approach, we design a two-stage procedure to perform self-supervised separation on audio mixtures. Using visual information as guidance, a primary-stage separation is realized via a U-net network, then the residual spectrogram is calculated by removing separated spectrograms from the original audio mixture. At the second-stage separation, a cycle-separation module is proposed to refine separation using separated results and the residual spectrogram. Self-supervision learning between vision and audio modalities is presented to push the cycle separation until the residual spectrogram becomes empty. Extensive experiments are evaluated on three large-scale datasets, MUSIC (MUSIC-21), AudioSet, and VGGSound. Experiment results certify that our approach outperforms the state-of-the-art approaches, and demonstrate the effectiveness for separating audio mixtures with overlap and interaction.
更多查看译文
关键词
Audio source separation,Fine-grained Cycle-Separation (FCSN) Network,Self-supervised learning,Visual-guided separation
AI 理解论文
溯源树
样例
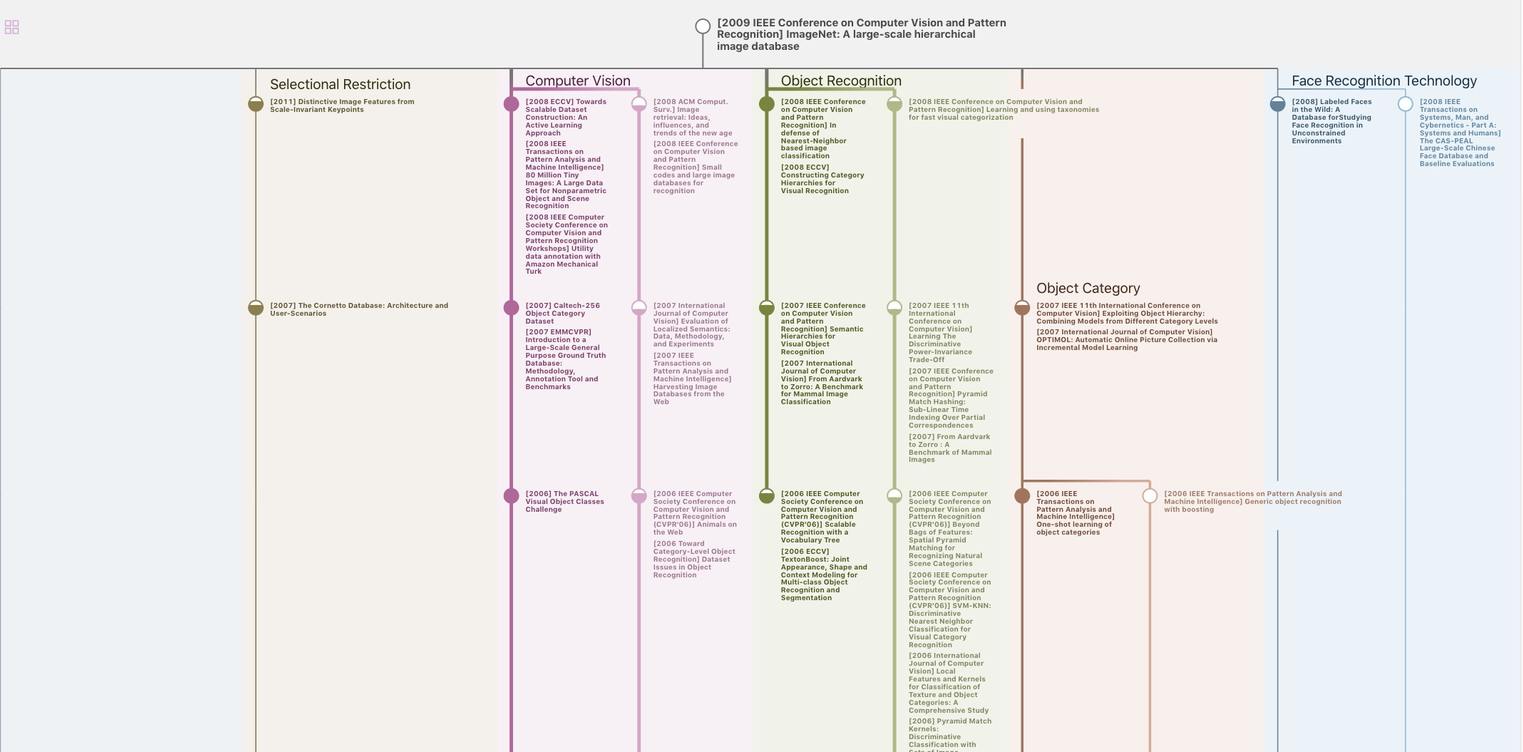
生成溯源树,研究论文发展脉络
Chat Paper
正在生成论文摘要