Detection of Colorectal Adenocarcinoma and Grading Dysplasia on Histopathologic Slides Using Deep Learning
The American Journal of Pathology(2023)
摘要
Colorectal cancer (CRC) is one of the most common types of cancer among men and women. The grading of dysplasia and the detection of adenocarcinoma are important clinical tasks in the diagnosis of CRC and shape the patients' follow-up plans. This study evaluated the feasibility of deep learning models for the classification of colorectal lesions into four classes: benign, low-grade dysplasia, high-grade dysplasia, and adenocarcinoma. To this end, a deep neural network was developed on a training set of 655 whole slide images of digitized colorectal resection slides from a tertiary medical institution; and the network was evaluated on an internal test set of 234 slides, as well as on an external test set of 606 adenocarcinoma slides from The Cancer Genome Atlas database. The model achieved an overall accuracy, sensitivity, and specificity of 95.5%, 91.0%, and 97.1%, respectively, on the internal test set, and an accuracy and sensitivity of 98.5% for adenocarcinoma detection task on the external test set. Results suggest that such deep learning models can potentially assist pathologists in grading colorectal dysplasia, detecting adenocarcinoma, prescreening, and prioritizing the reviewing of suspicious cases to improve the turnaround time for patients with a high risk of CRC. Furthermore, the high sensitivity on the external test set suggests the model's generalizability in detecting colorectal adenocarcinoma on whole slide images across different institutions.
更多查看译文
关键词
colorectal cancer,deep learning,digital pathology,dysplasia,whole-slide imaging
AI 理解论文
溯源树
样例
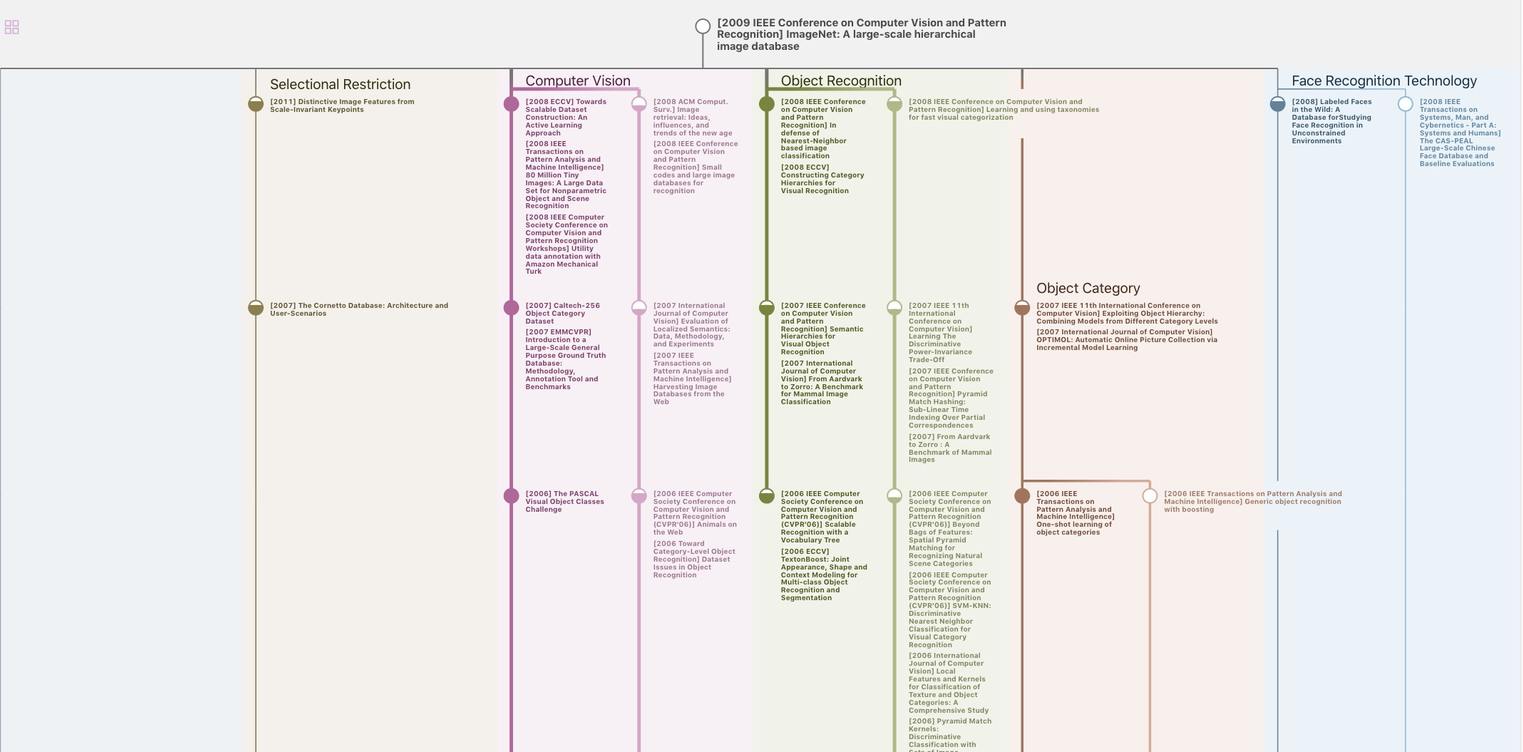
生成溯源树,研究论文发展脉络
Chat Paper
正在生成论文摘要