Automated segmentation of fetal intracranial volume in 3D ultrasound using deep learning: identifying sex differences in prenatal brain development
biorxiv(2022)
摘要
The human brain undergoes major developmental changes during pregnancy. Three-dimensional (3D) ultrasound images allow for the opportunity to investigate typical prenatal brain development on a large scale. Here, we developed a convolutional neural network (CNN) model for automated segmentation of fetal intracranial volume (ICV) from 3D ultrasound, and we applied the trained model in a large independent sample (N = 9795 ultrasounds; N=1763 participants) from the YOUth Baby and Child cohort measured at 20- and 30-week of gestational age to investigate sex differences in fetal ICV and ICV growth. 168 3D ultrasound images with ICV annotations were included to develop a 3D CNN model for automated fetal ICV segmentation. A data augmentation strategy provided physical variation and additional data to train the network. K-fold cross-validation and Bayesian optimization were used for network selection and the ensemble-based system combined multiple networks to form the final ensemble network. The final ensemble network produced consistent and high-quality segmentations of ICV. Our trained model successfully predicted ICV and ICV growth in a large independent longitudinal data set. Boys had significantly larger ICV at 20- (B=2.83; p=1.4e-13) and 30-weeks of pregnancy (B=12.01; p=2.0e-28), and more pronounced ICV growth than girls (t=-4.395; p=1.3e-5). Our approach provides us with the opportunity to investigate fetal brain development on a much larger scale and to answer fundamental questions related to prenatal brain development.
### Competing Interest Statement
The authors have declared no competing interest.
更多查看译文
关键词
fetal intracranial volume,3d ultrasound,prenatal brain development,deep learning
AI 理解论文
溯源树
样例
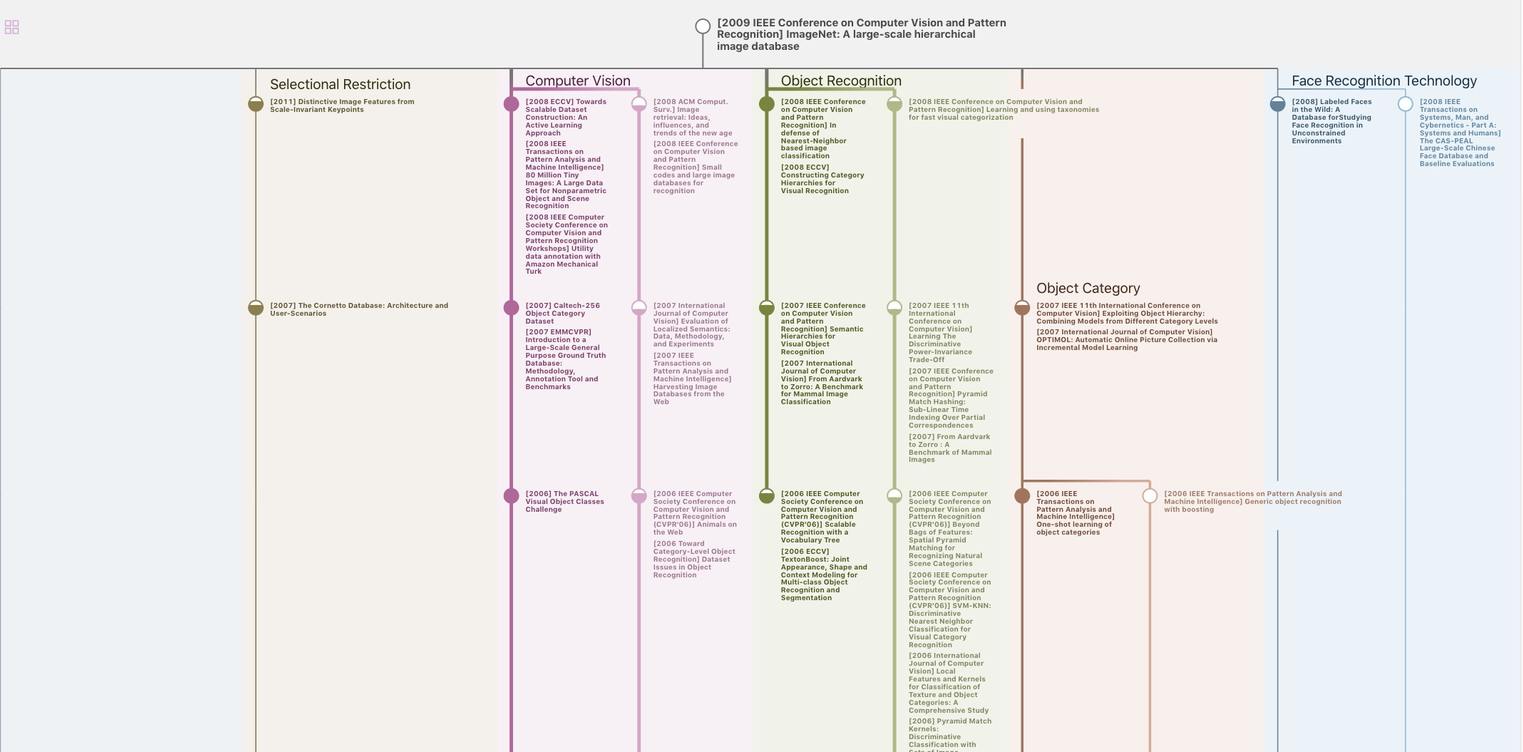
生成溯源树,研究论文发展脉络
Chat Paper
正在生成论文摘要