Multitask Instruction-based Prompting for Fallacy Recognition
emnlp 2022(2022)
摘要
Fallacies are used as seemingly valid arguments to support a position and persuade the audience about its validity. Recognizing fallacies is an intrinsically difficult task both for humans and machines. Moreover, a big challenge for computational models lies in the fact that fallacies are formulated differently across the datasets with differences in the input format (e.g., question-answer pair, sentence with fallacy fragment), genre (e.g., social media, dialogue, news), as well as types and number of fallacies (from 5 to 18 types per dataset). To move towards solving the fallacy recognition task, we approach these differences across datasets as multiple tasks and show how instruction-based prompting in a multitask setup based on the T5 model improves the results against approaches built for a specific dataset such as T5, BERT or GPT-3. We show the ability of this multitask prompting approach to recognize 28 unique fallacies across domains and genres and study the effect of model size and prompt choice by analyzing the per-class (i.e., fallacy type) results. Finally, we analyze the effect of annotation quality on model performance, and the feasibility of complementing this approach with external knowledge.
更多查看译文
关键词
fallacy,prompting,instruction-based
AI 理解论文
溯源树
样例
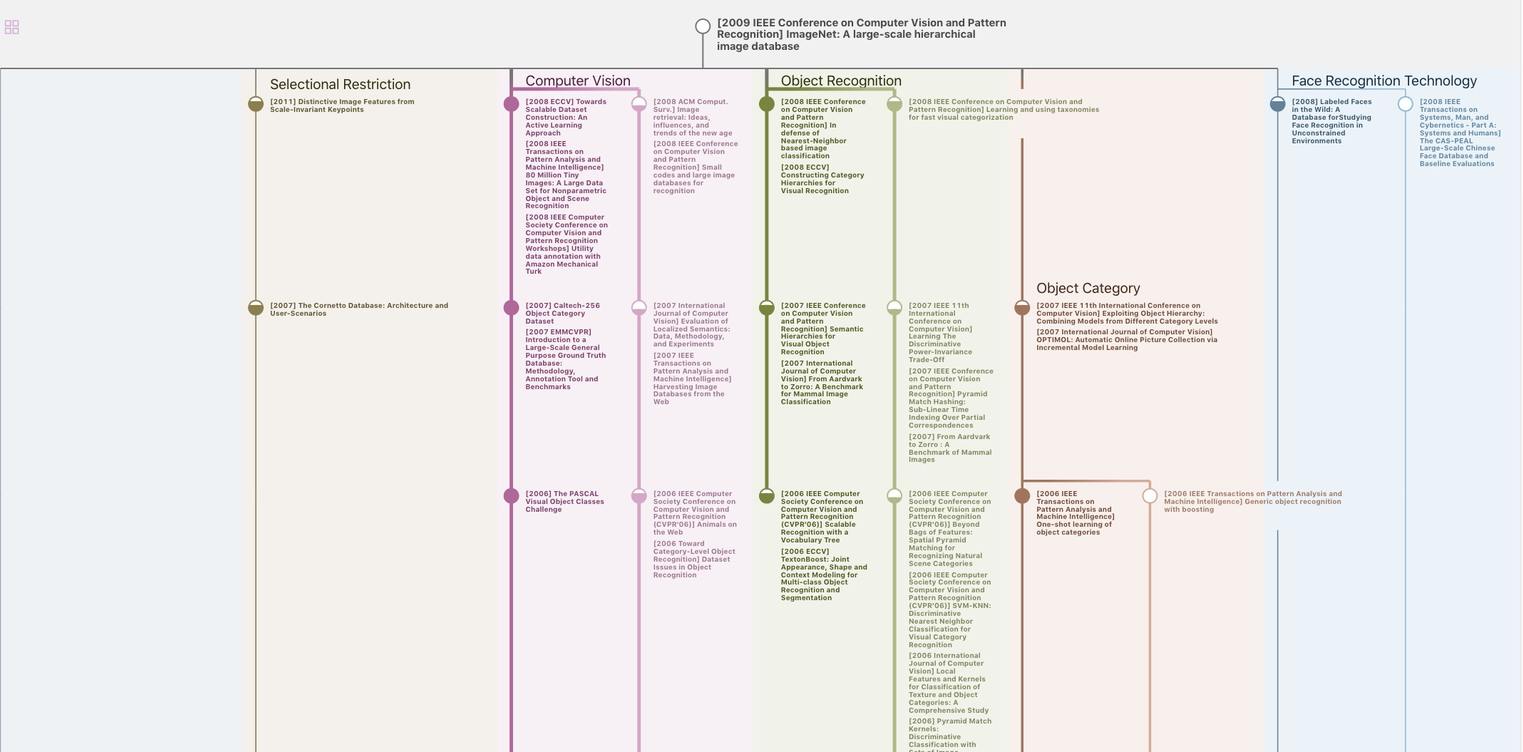
生成溯源树,研究论文发展脉络
Chat Paper
正在生成论文摘要