Improving the Imperceptibility of Adversarial Examples Based on Weakly Perceptual Perturbation in Key Regions
Security and communication networks(2022)
摘要
Deep neural networks have been proved vulnerable to being attacked by adversarial examples, which have attracted extensive attention from researchers. Existing GAN-based object detection adversarial example generation methods are efficient in generating speed but ignore the visual imperceptibility of adversarial examples. In this paper, to improve the visual imperceptibility of adversarial examples, we propose an object detection adversarial example generation method based on weakly perceptual perturbations in key regions. First, a positioning module based on the gradient-weighted activation mapping method is designed to analyze the key region of the object from the perspective of gradient propagation and use the key region in order to limit the range and amplitude of the perturbation. Second, the deep feature of the convolutional network is introduced to constrain the content of adversarial perturbation and improve the similarity between adversarial examples and original images. Finally, a postprocessing method based on median filtering is introduced to further correct the color deviation of adversarial examples and improve imperceptibility. The experimental results for VOC datasets show that the attack success rate increased by 4%, the PSNR increased by 8.6%, and the MSE and LPIPS decreased by 92.3% and 59.5%, respectively. It demonstrates that the proposed method can significantly improve the imperceptibility of the adversarial example with a high attack success rate.
更多查看译文
AI 理解论文
溯源树
样例
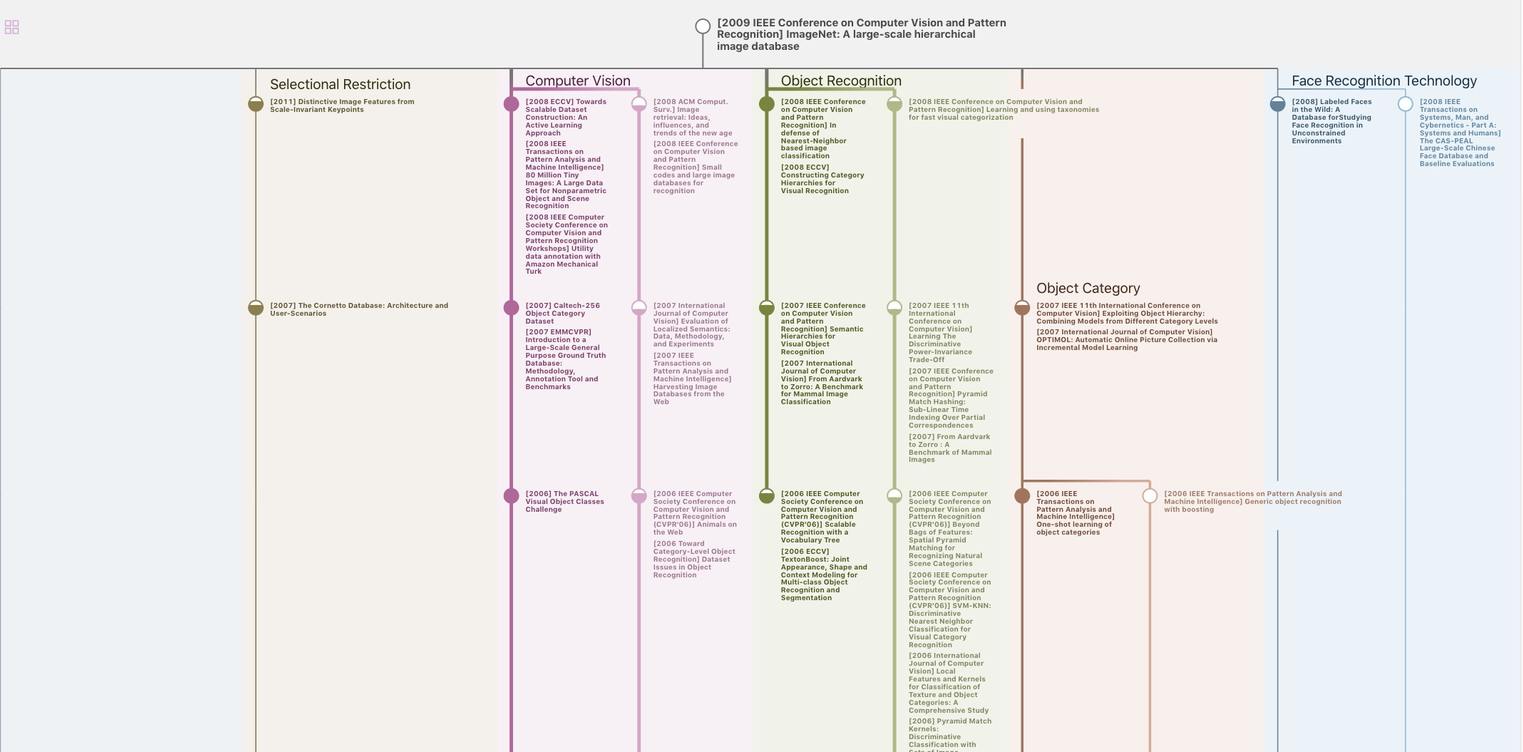
生成溯源树,研究论文发展脉络
Chat Paper
正在生成论文摘要