PIM: Video Coding using Perceptual Importance Maps
arxiv(2023)
摘要
Human perception is at the core of lossy video compression, with numerous approaches developed for perceptual quality assessment and improvement over the past two decades. In the determination of perceptual quality, different spatio-temporal regions of the video differ in their relative importance to the human viewer. However, since it is challenging to infer or even collect such fine-grained information, it is often not used during compression beyond low-level heuristics. We present a framework which facilitates research into fine-grained subjective importance in compressed videos, which we then utilize to improve the rate-distortion performance of an existing video codec (x264). The contributions of this work are threefold: (1) we introduce a web-tool which allows scalable collection of fine-grained perceptual importance, by having users interactively paint spatio-temporal maps over encoded videos; (2) we use this tool to collect a dataset with 178 videos with a total of 14443 frames of human annotated spatio-temporal importance maps over the videos; and (3) we use our curated dataset to train a lightweight machine learning model which can predict these spatio-temporal importance regions. We demonstrate via a subjective study that encoding the videos in our dataset while taking into account the importance maps leads to higher perceptual quality at the same bitrate, with the videos encoded with importance maps preferred $1.8 \times$ over the baseline videos. Similarly, we show that for the 18 videos in test set, the importance maps predicted by our model lead to higher perceptual quality videos, $2 \times$ preferred over the baseline at the same bitrate.
更多查看译文
关键词
perceptual importance maps,video coding
AI 理解论文
溯源树
样例
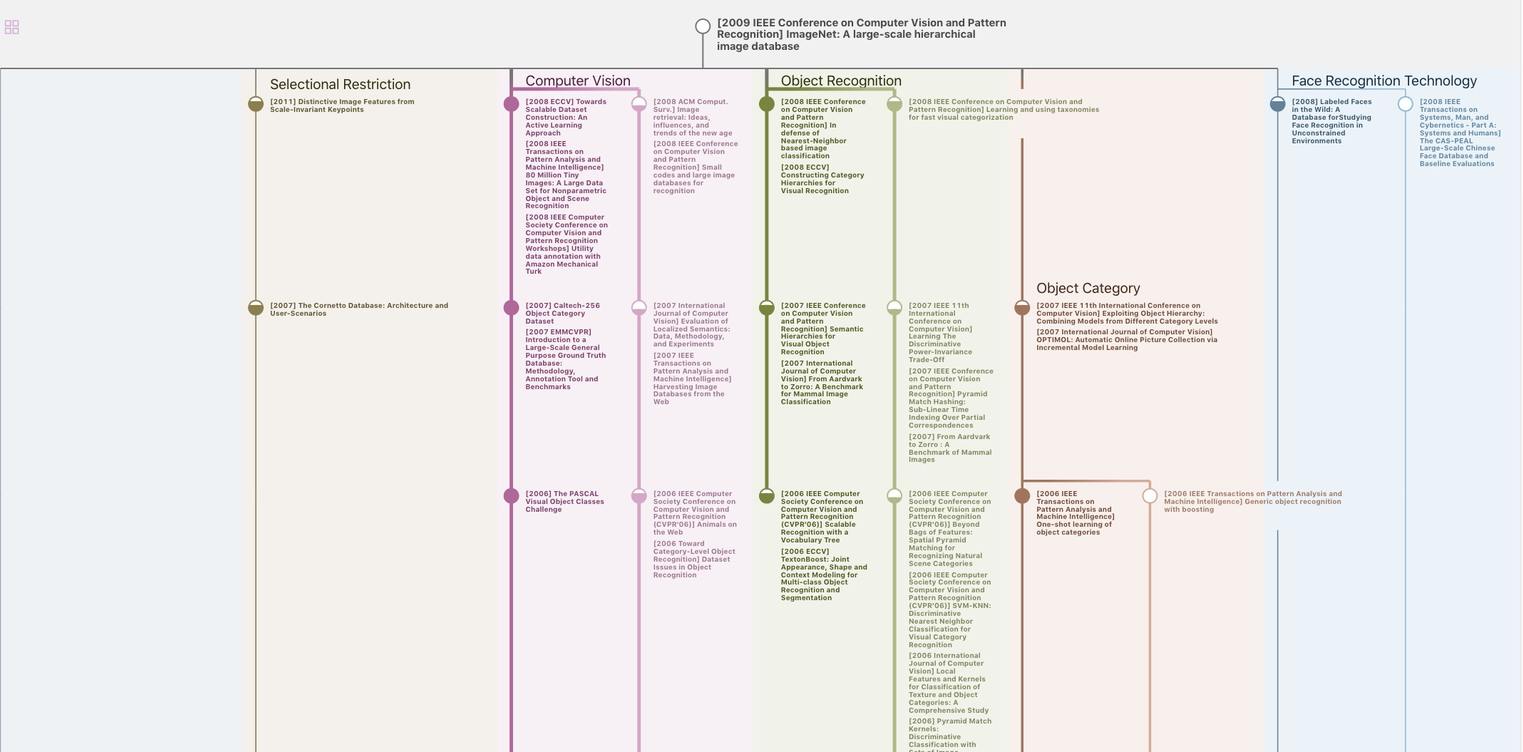
生成溯源树,研究论文发展脉络
Chat Paper
正在生成论文摘要