Joint Embedding of 2D and 3D Networks for Medical Image Anomaly Detection
arxiv(2022)
摘要
Obtaining ground truth data in medical imaging has difficulties due to the fact that it requires a lot of annotating time from the experts in the field. Also, when trained with supervised learning, it detects only the cases included in the labels. In real practice, we want to also open to other possibilities than the named cases while examining the medical images. As a solution, the need for anomaly detection that can detect and localize abnormalities by learning the normal characteristics using only normal images is emerging. With medical image data, we can design either 2D or 3D networks of self-supervised learning for anomaly detection task. Although 3D networks, which learns 3D structures of the human body, show good performance in 3D medical image anomaly detection, they cannot be stacked in deeper layers due to memory problems. While 2D networks have advantage in feature detection, they lack 3D context information. In this paper, we develop a method for combining the strength of the 3D network and the strength of the 2D network through joint embedding. We also propose the pretask of self-supervised learning to make it possible for the networks to learn efficiently. Through the experiments, we show that the proposed method achieves better performance in both classification and segmentation tasks compared to the SoTA method.
更多查看译文
关键词
3d networks,medical image anomaly
AI 理解论文
溯源树
样例
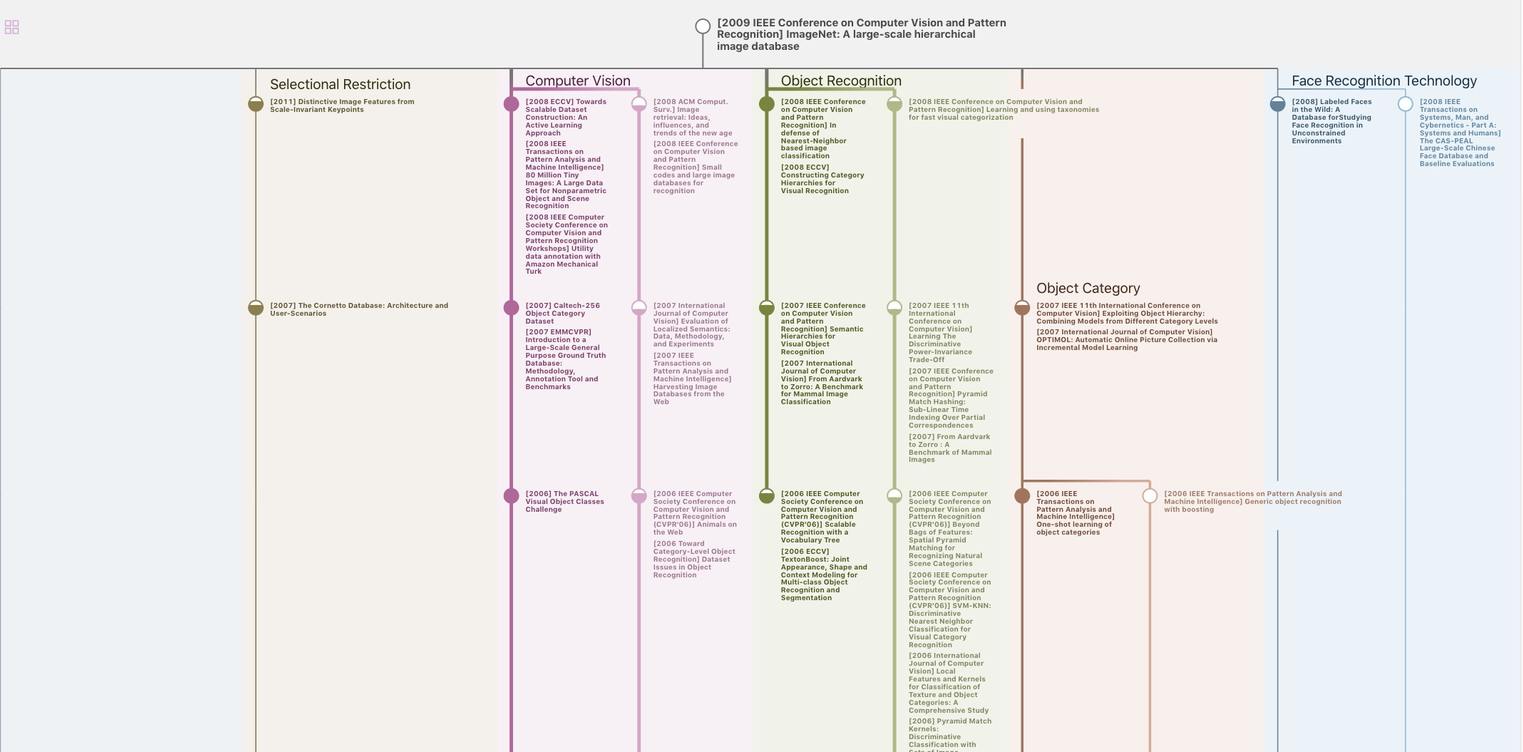
生成溯源树,研究论文发展脉络
Chat Paper
正在生成论文摘要