Predicting Invasive Coronary Perfusion Pressure Using Noninvasive Electrocardiogram and Photoplethysmography Based on Machine Learning for Cardiopulmonary Resuscitation
2022 15th International Congress on Image and Signal Processing, BioMedical Engineering and Informatics (CISP-BMEI)(2022)
摘要
Cardiac arrest is a leading reason for death. High-quality cardiopulmonary resuscitation (CPR) is considered to be an effective treatment for cardiac arrest. A big challenge is how to improve the quality of CPR based on the real-time physiological status. It would be of interest to find a way to predict the invasive coronary perfusion pressure (CPP) based on noninvasive physiological parameters. This study aimed to establish a novel approach to predict the invasive CPP with the noninvasive electrocardiogram (ECG) and photoplethysmography (PPG), based on signal processing and machine learning techniques. Three data sets were examined for the CPP prediction, including (a) ECG only, (b) PPG only, and (c) a combination of both ECG and PPG. Four machine learning algorithms were applied for the CPP prediction, including support vector regression, K nearest Neighbor, gradient boosted regression tree, and random forest. Results showed that the random forest model with a combined dataset of both ECG and PPG could achieve optimal prediction for the CPP. In this case, the mean absolute error was 4.176 mmHg, the root mean square error was 6.722 mmHg, and the adjusted determination coefficient of the model was 0.755. Strong correlations between the predicted and real CPP values were found, which confirmed our hypothesis that the invasive CPP could be predicted by non-invasive physiological indicators, such as the ECG and PPG parameters. This study sheds light on the role of machine learning in CPP prediction and may facilitate to develop high-quality CPR treatment for particularly cardiac arrest out of the hospital.
更多查看译文
关键词
Cardiac arrest,cardiopulmonary resuscitation,machine learning,coronary perfusion pressure,prediction model
AI 理解论文
溯源树
样例
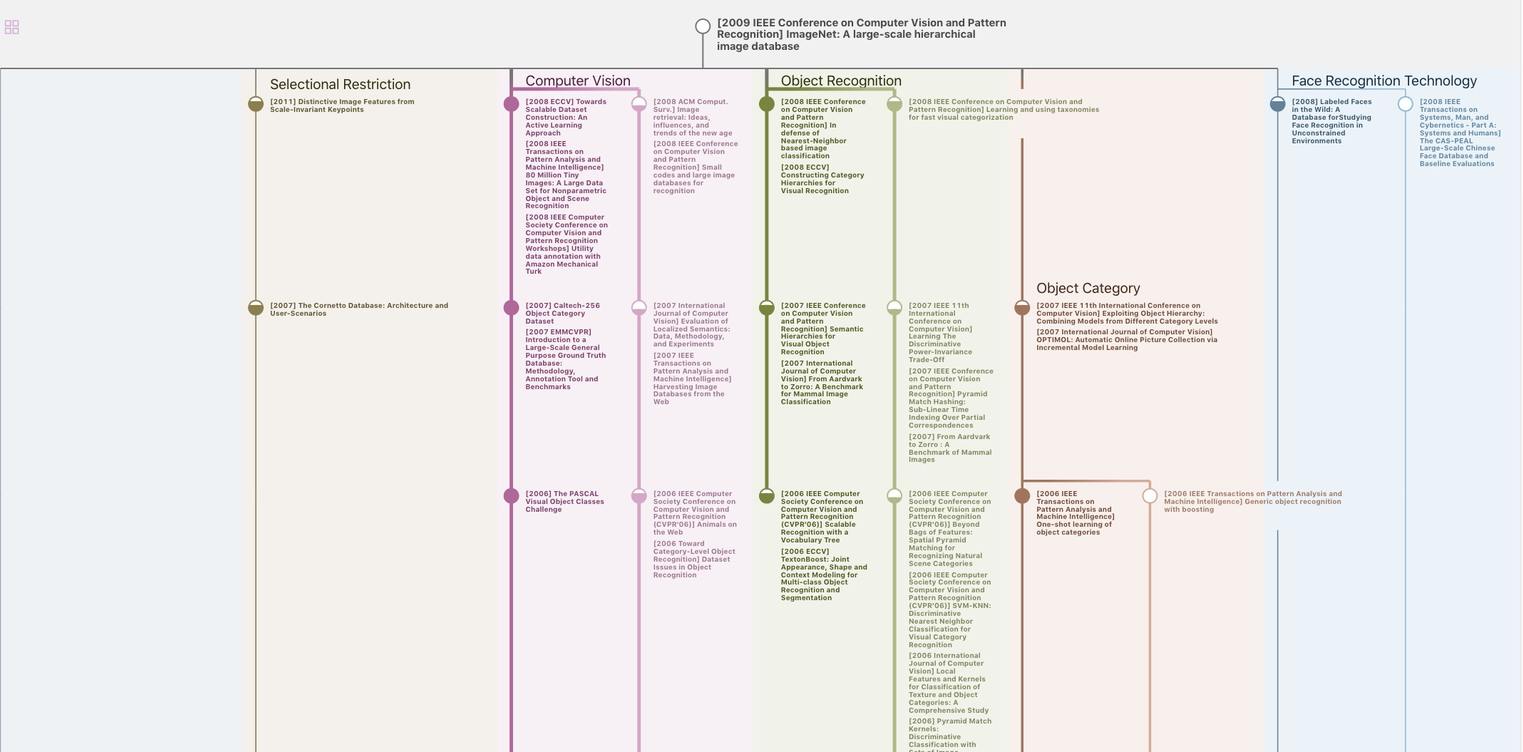
生成溯源树,研究论文发展脉络
Chat Paper
正在生成论文摘要