Sparse Fourier Backpropagation in Cryo-EM Reconstruction
NeurIPS 2022(2022)
摘要
Electron cryo-microscopy (cryo-EM) is a powerful method for investigating the structures of protein molecules, with important implications for understanding the molecular processes of life and drug development. In this technique, many noisy, two-dimensional projection images of protein molecules in unknown poses are combined into one or more three-dimensional reconstructions. The presence of multiple structural states in the data represents a major bottleneck in existing processing pipelines, often requiring expert user supervision. Variational auto-encoders (VAEs) have recently been proposed as an attractive means for learning the data manifold of data sets with a large number of different states. These methods are based on a coordinate-based approach, similar to Neural Radiance Fields (NeRF), to make volumetric reconstructions from 2D image data in Fourier-space. Although NeRF is a powerful method for real-space reconstruction, many of the benefits of the method do not transfer to Fourier-space, e.g. inductive bias for spatial locality. We present an approach where the VAE reconstruction is expressed on a volumetric grid, and demonstrate how this model can be trained efficiently through a novel backpropagation method that exploits the sparsity of the projection operation in Fourier-space. We achieve improved results on a simulated data set and at least equivalent results on an experimental data set when compared to the coordinate-based approach, while also substantially lowering computational cost. Our approach is computationally more efficient, especially in inference, enabling interactive analysis of the latent space by the user.
更多查看译文
关键词
cryo-EM,NeRF,Fourier space reconstruction,VAE,generative modeling
AI 理解论文
溯源树
样例
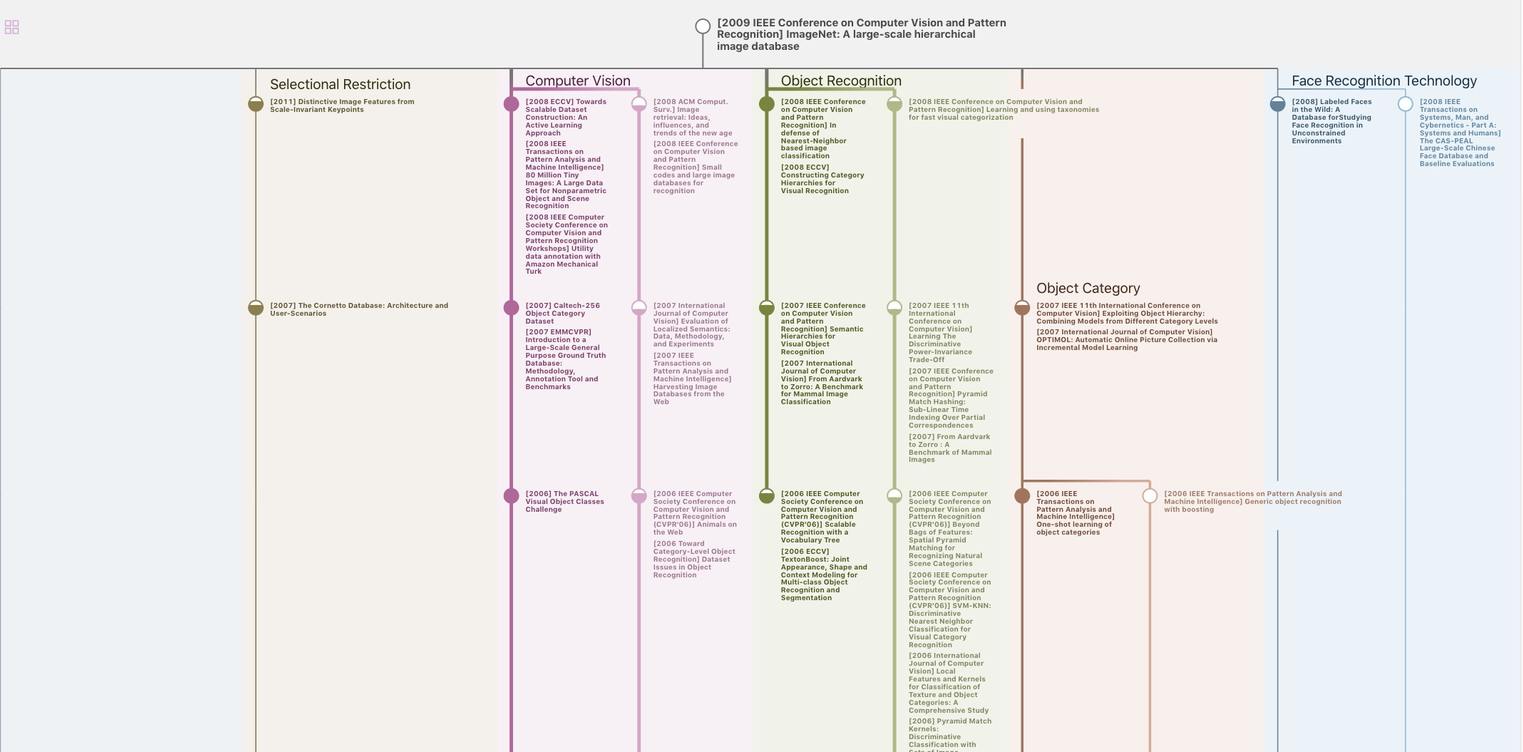
生成溯源树,研究论文发展脉络
Chat Paper
正在生成论文摘要