Fixed-Distance Hamiltonian Monte Carlo
NeurIPS 2022(2022)
摘要
We propose a variation of the Hamiltonian Monte Carlo sampling (HMC) where the equations of motion are simulated for a fixed traversed distance rather than the conventional fixed simulation time. This new mechanism tends to generate proposals that have higher target probability values. The momentum distribution that is naturally joint with our Fixed-Distance HMC (FDHMC), and keeps the proposal acceptance probability close to 1, is not Gaussian and generates momentums that have a higher expected magnitude. This translates into a reduced correlation between the successive MCMC states and according to our experimental results, leads to an improvement in terms of the effective sample size per gradient when compared to the baseline HMC and No-U-Turn (NUTS) samplers.
更多查看译文
关键词
Markov Chain Monte Carlo,Sampling,Hamiltonian Monte Carlo,Reversible Jump,RJMCMC,No-U-Turn sampler
AI 理解论文
溯源树
样例
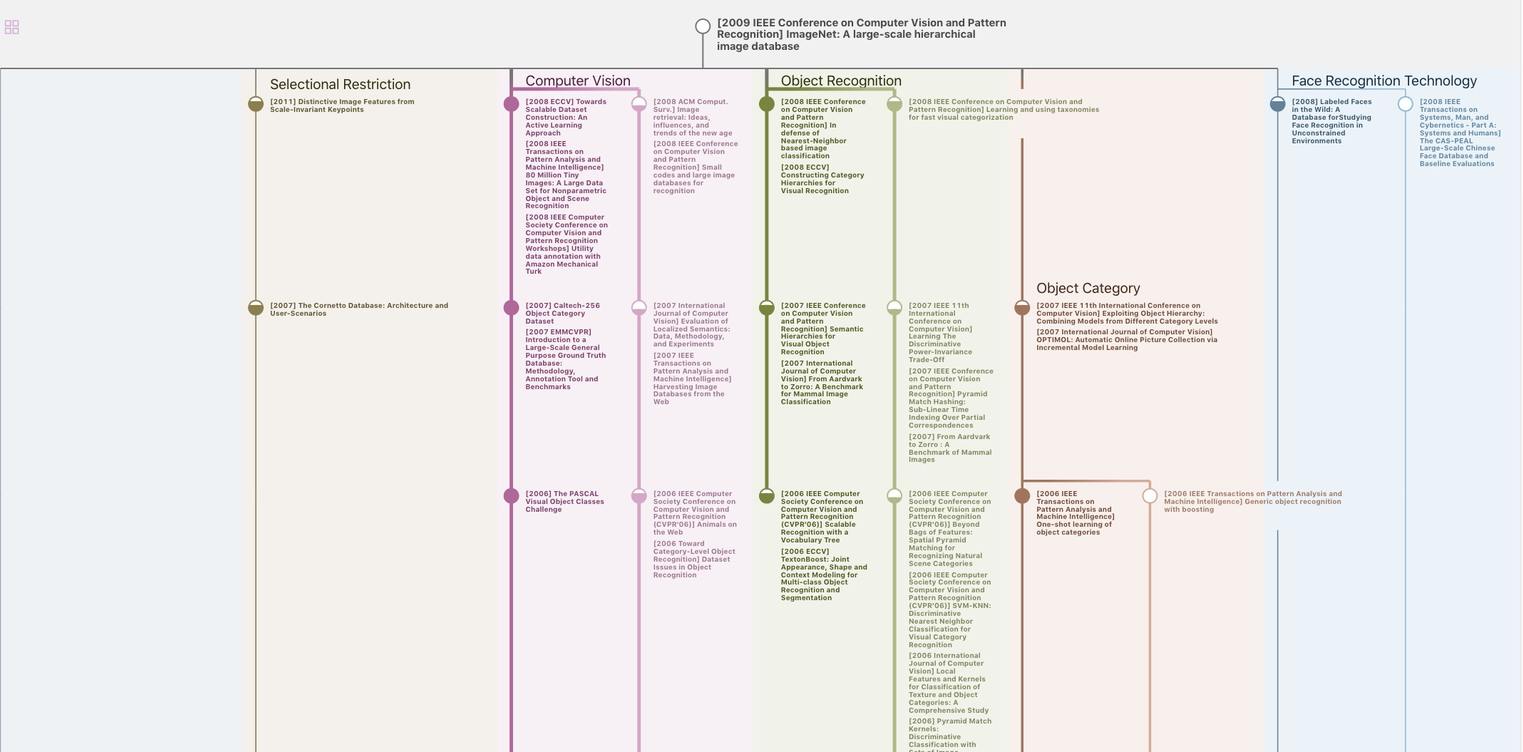
生成溯源树,研究论文发展脉络
Chat Paper
正在生成论文摘要