Improved Fine-Tuning by Better Leveraging Pre-Training Data
NeurIPS 2022(2022)
摘要
As a dominant paradigm, fine-tuning a pre-trained model on the target data is widely used in many deep learning applications, especially for small data sets. However, recent studies have empirically shown that training from scratch has the final performance that is no worse than this pre-training strategy once the number of training samples is increased in some vision tasks. In this work, we revisit this phenomenon from the perspective of generalization analysis by using excess risk bound which is popular in learning theory. The result reveals that the excess risk bound may have a weak dependency on the pre-trained model. The observation inspires us to leverage pre-training data for fine-tuning, since this data is also available for fine-tuning. The generalization result of using pre-training data shows that the excess risk bound on a target task can be improved when the appropriate pre-training data is included in fine-tuning. With the theoretical motivation, we propose a novel selection strategy to select a subset from pre-training data to help improve the generalization on the target task. Extensive experimental results for image classification tasks on 8 benchmark data sets verify the effectiveness of the proposed data selection based fine-tuning pipeline.
更多查看译文
关键词
Pre-Training,Fine-Tuning,Learning Theory,Self-Supervision
AI 理解论文
溯源树
样例
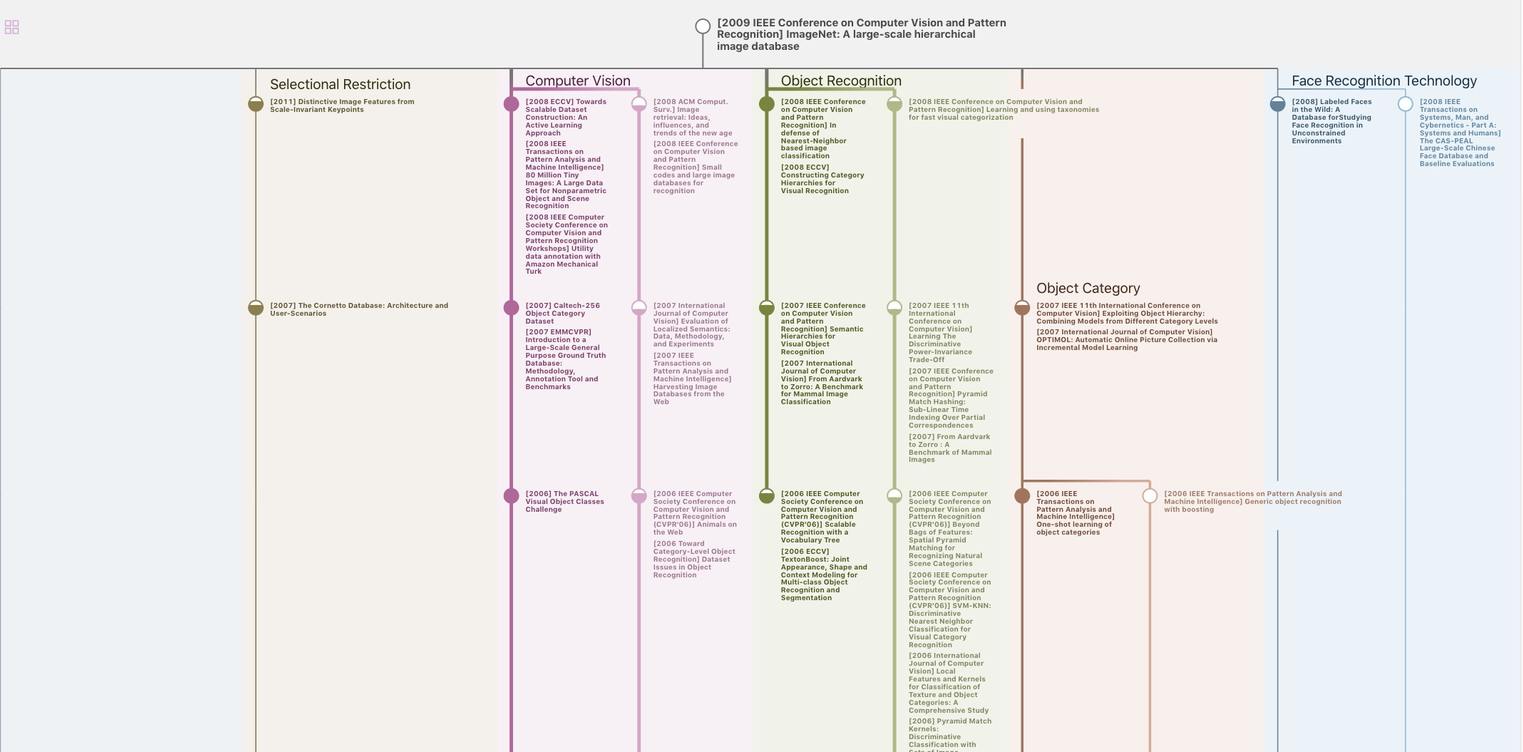
生成溯源树,研究论文发展脉络
Chat Paper
正在生成论文摘要