Learning Team Decisions
arxiv(2022)
摘要
In this paper, we treat linear quadratic team decision problems, where a team of agents minimizes a convex quadratic cost function over $T$ time steps subject to possibly distinct linear measurements of the state of nature. We assume that the state of nature is a Gaussian random variable and that the agents do not know the cost function nor the linear functions mapping the state of nature to their measurements. We present a gradient-descent based algorithm with an expected regret of $O(\log(T))$ for full information gradient feedback and $O(\sqrt(T))$ for bandit feedback. In the case of bandit feedback, the expected regret has an additional multiplicative term $O(d)$ where $d$ reflects the number of learned parameters.
更多查看译文
关键词
learning,team
AI 理解论文
溯源树
样例
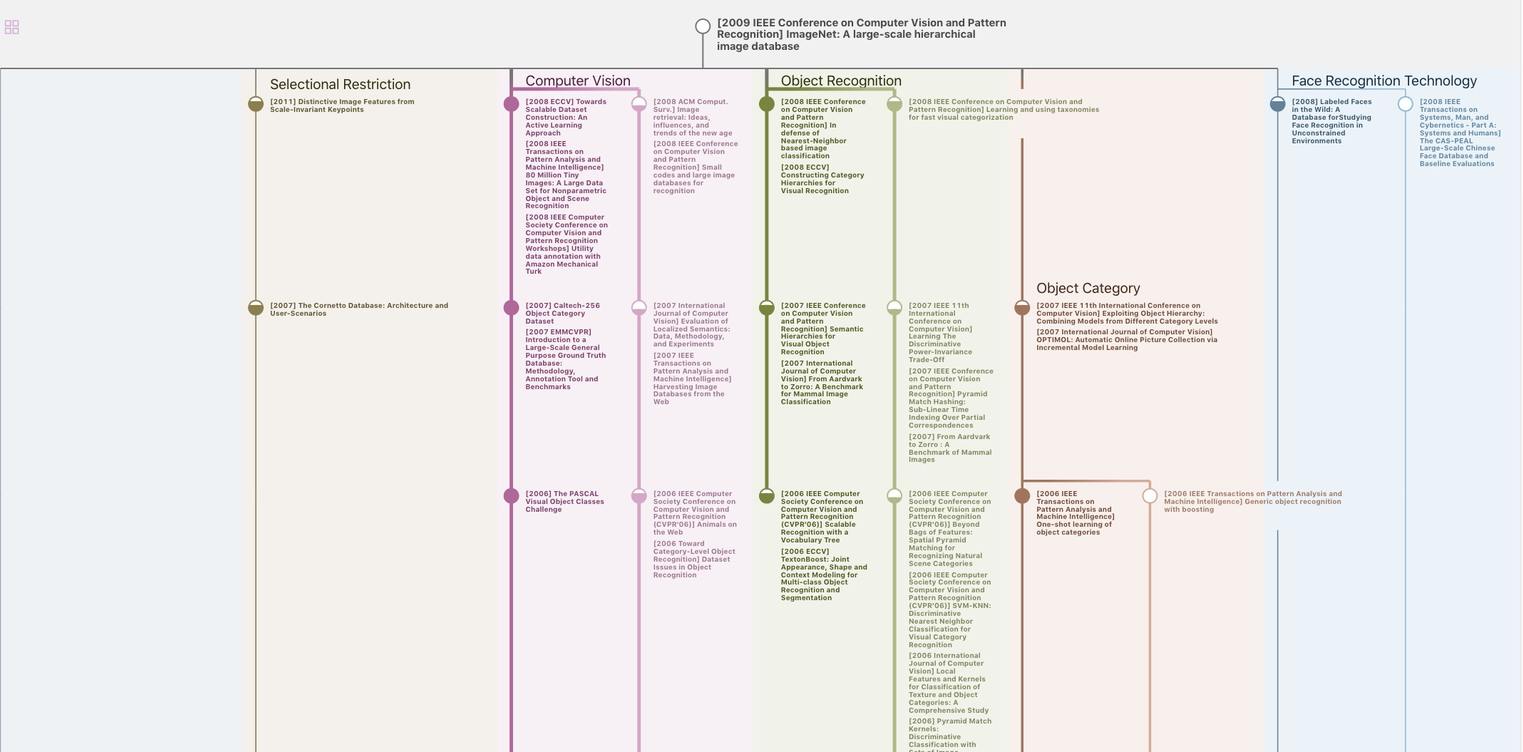
生成溯源树,研究论文发展脉络
Chat Paper
正在生成论文摘要