Scalable Primal Decomposition Schemes for Large-Scale Infrastructure Networks
arxiv(2022)
摘要
The real-time operation of large-scale infrastructure networks requires scalable optimization capabilities. Decomposition schemes can help achieve scalability; classical decomposition approaches such as the alternating direction method of multipliers (ADMM) and distributed Newtons schemes, however, often either suffer from slow convergence or might require high degrees of communication. In this work, we present new primal decomposition schemes for solving large-scale, strongly convex QPs. These approaches have global convergence guarantees and require limited communication. We benchmark their performance against the off-the-shelf interior-point method Ipopt and against ADMM on infrastructure networks that contain up to 300,000 decision variables and constraints. Overall, we find that the proposed approaches solve problems as fast as Ipopt but with reduced communication. Moreover, we find that the proposed schemes achieve higher accuracy than ADMM approaches.
更多查看译文
关键词
scalable primal decomposition schemes,infrastructure,networks,large-scale
AI 理解论文
溯源树
样例
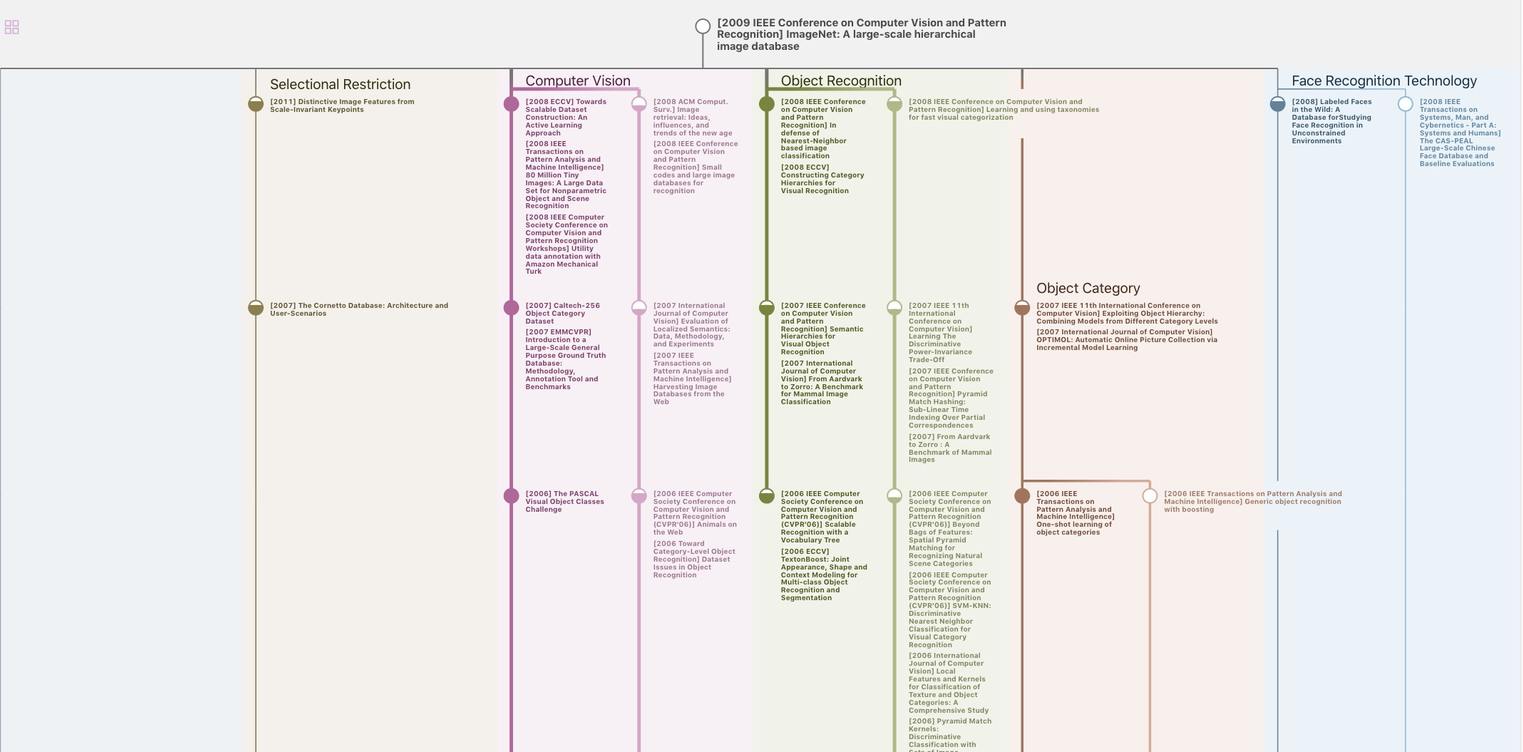
生成溯源树,研究论文发展脉络
Chat Paper
正在生成论文摘要