Operating Performance Assessment of Complex Nonlinear Industrial Process Based on Kernel Locally Linear Embedding PLS
IEEE TRANSACTIONS ON AUTOMATION SCIENCE AND ENGINEERING(2024)
摘要
The process data is strongly nonlinear due to variations in system performance caused by machine operation or condition; many nonlinear methods for operating performance assessment, which focus on capturing information about the global of each performance grade while ignoring local information, have difficulty dealing with this strong nonlinearity. Aiming at the problems of strong nonlinearity and incomplete information extraction, an operating performance assessment method based on kernel locally linear embedding partial least squares (KLLEPLS) is proposed. Firstly, the local and global information of the dataset is mined using kernel locally linear embedding (KLLE) and partial least squares (PLS). Secondly, to simplify the computation, a new method, embedding KLLE into PLS, is introduced to make the solution similar to KPLS. Then, an assessment index is introduced into online assessment; when the performance is non-optimal, the contribution rates of the variables are calculated based on the extracted local and global information to determine the possible cause variable. Finally, the practice and effectiveness of the proposed algorithm are verified by the dense medium coal preparation and the Tennessee Eastman (TE) process. Note to Practitioners-Due to the presence of noise and interference, operating performance of the process is non-optimal and the control effect is difficult to meet the actual production requirements. The motivation of this paper is to find the non- optimal operation points in time, identify the reasons, feedback to the operator, and make adjustments to maximize the comprehensive economic benefits of the enterprise. The process data is strongly nonlinear due to variations in system performance caused by machine operation or condition, and some nonlinear methods are not complete to extract feature information for this strong nonlinearity. Therefore, this paper proposes the kernel local linear embedding partial least squares (KLLEPLS) algorithm, and uses it to build a model to evaluate whether the current operation is in an optimal performance; when it is in the non-optimal performance, possible variable is identified and the process is adjusted in time to ensure that it operates under optimal conditions. This method extracts more comprehensive information compared with the traditional methods, which can provide more accurate adjustment basis for operators.
更多查看译文
关键词
Complex nonlinear industrial process,kernel locally linear embedding PLS (KLLEPLS),non-optimal cause identification,operating performance assessment
AI 理解论文
溯源树
样例
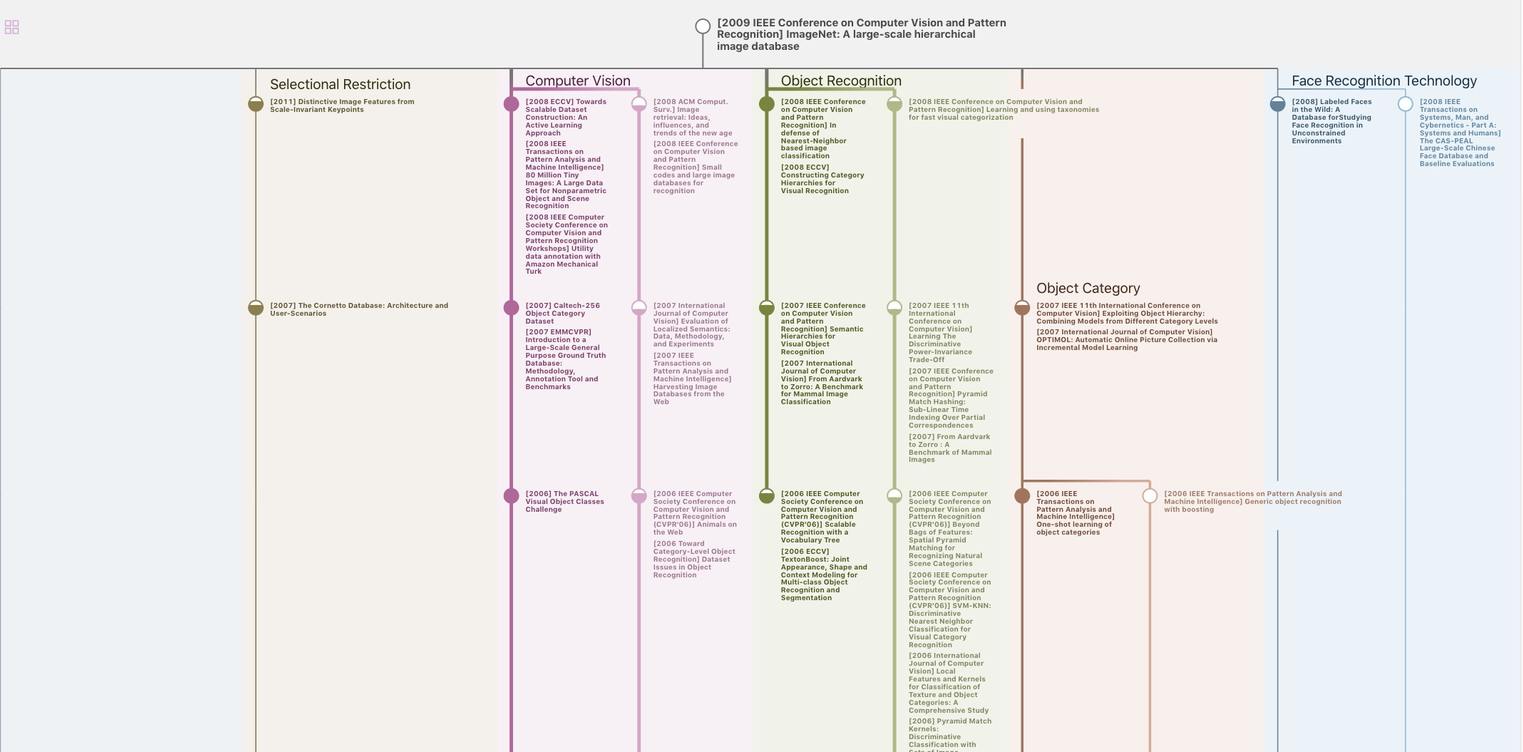
生成溯源树,研究论文发展脉络
Chat Paper
正在生成论文摘要