C-ToMExO: Learning Cancer Progression Dynamics from Clonal Composition of Tumors
biorxiv(2022)
摘要
Cancer is an evolutionary process involving the accumulation of somatic mutations in the genome. The tumor's evolution is known to be highly influenced by specific somatic mutations in so-called cancer driver genes. Cancer progression models are computational tools used to infer the interactions among cancer driver genes by analyzing the pattern of absence/presence of mutations in different tumors of a cohort. In an abundance of subclonal mutations, discarding the heterogeneity of tumors and investigating the interrelations among the driver genes solely based on tumor-level data can result in misleading interpretations. In this paper, we introduce a computational approach to infer cancer progression models from the clone-level data gathered from a cohort of tumors. Our method leverages the rich clone-level data to identify the patterns of interactions among cancer driver genes and produce significantly more robust and reliable cancer progression models. Using a novel efficient Markov Chain Monte Carlo inference algorithm, our method provides outstanding scalability to the rapidly increasing size of available datasets. Using an extensive set of synthetic data experiments, we demonstrate the performance of our inference method in recovering the generative progression models. Finally, we present our analysis of two sub-types of lung cancer using biological multi-regional bulk data.
### Competing Interest Statement
The authors have declared no competing interest.
更多查看译文
关键词
learning cancer progression dynamics,clonal composition,c-tomexo
AI 理解论文
溯源树
样例
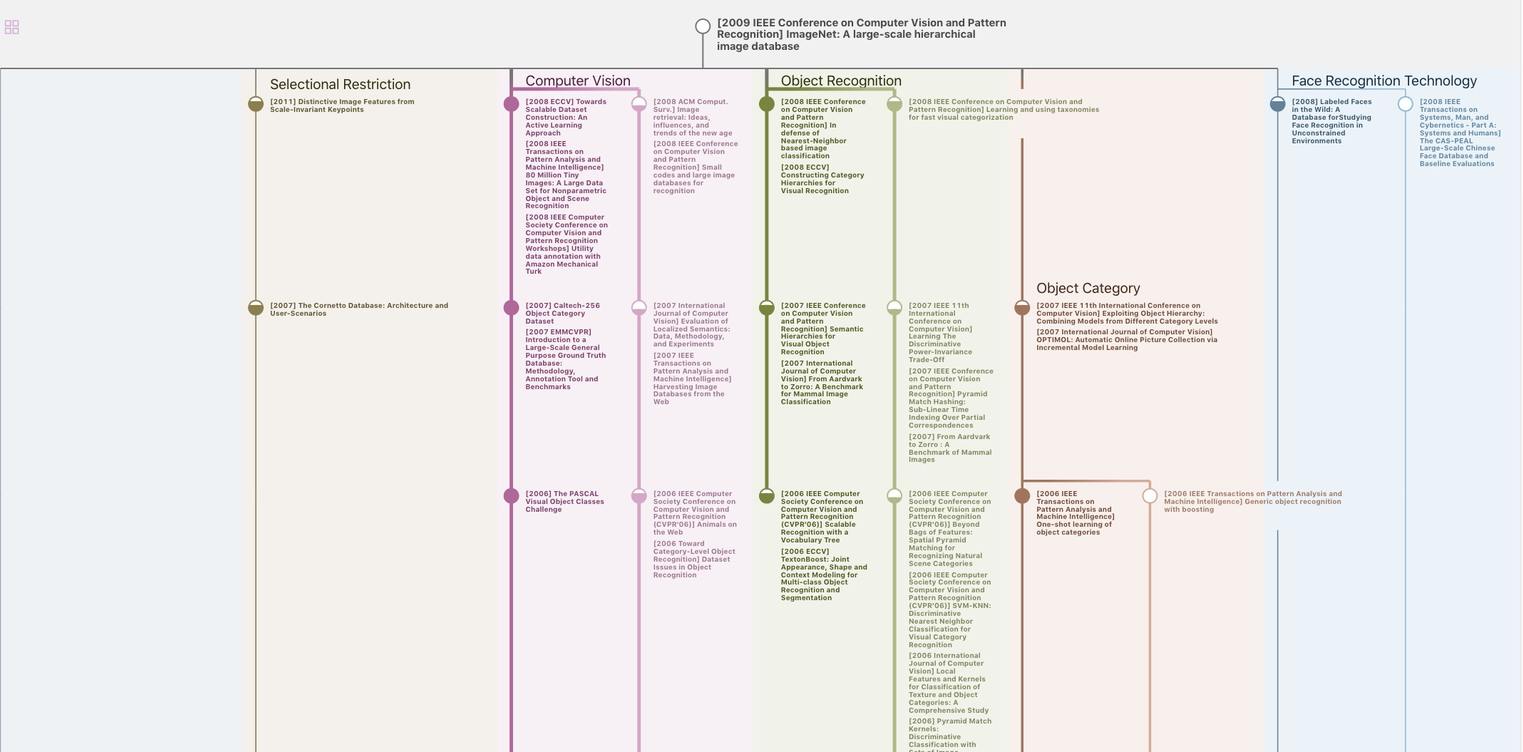
生成溯源树,研究论文发展脉络
Chat Paper
正在生成论文摘要