Interpretable and Predictive Deep Neural Network Modeling of the SARS-CoV-2 Spike Protein Sequence to Predict COVID-19 Disease Severity.
Biology(2022)
摘要
Through the COVID-19 pandemic, SARS-CoV-2 has gained and lost multiple mutations in novel or unexpected combinations. Predicting how complex mutations affect COVID-19 disease severity is critical in planning public health responses as the virus continues to evolve. This paper presents a novel computational framework to complement conventional lineage classification and applies it to predict the severe disease potential of viral genetic variation. The transformer-based neural network model architecture has additional layers that provide sample embeddings and sequence-wide attention for interpretation and visualization. First, training a model to predict SARS-CoV-2 taxonomy validates the architecture's interpretability. Second, an interpretable predictive model of disease severity is trained on spike protein sequence and patient metadata from GISAID. Confounding effects of changing patient demographics, increasing vaccination rates, and improving treatment over time are addressed by including demographics and case date as independent input to the neural network model. The resulting model can be interpreted to identify potentially significant virus mutations and proves to be a robust predctive tool. Although trained on sequence data obtained entirely before the availability of empirical data for Omicron, the model can predict the Omicron's reduced risk of severe disease, in accord with epidemiological and experimental data.
更多查看译文
关键词
COVID-19,SARS-CoV-2,bioinformatics,computational biology,coronavirus,deep learning,explainable artificial intelligence,machine learning,neural networks,viral genomics
AI 理解论文
溯源树
样例
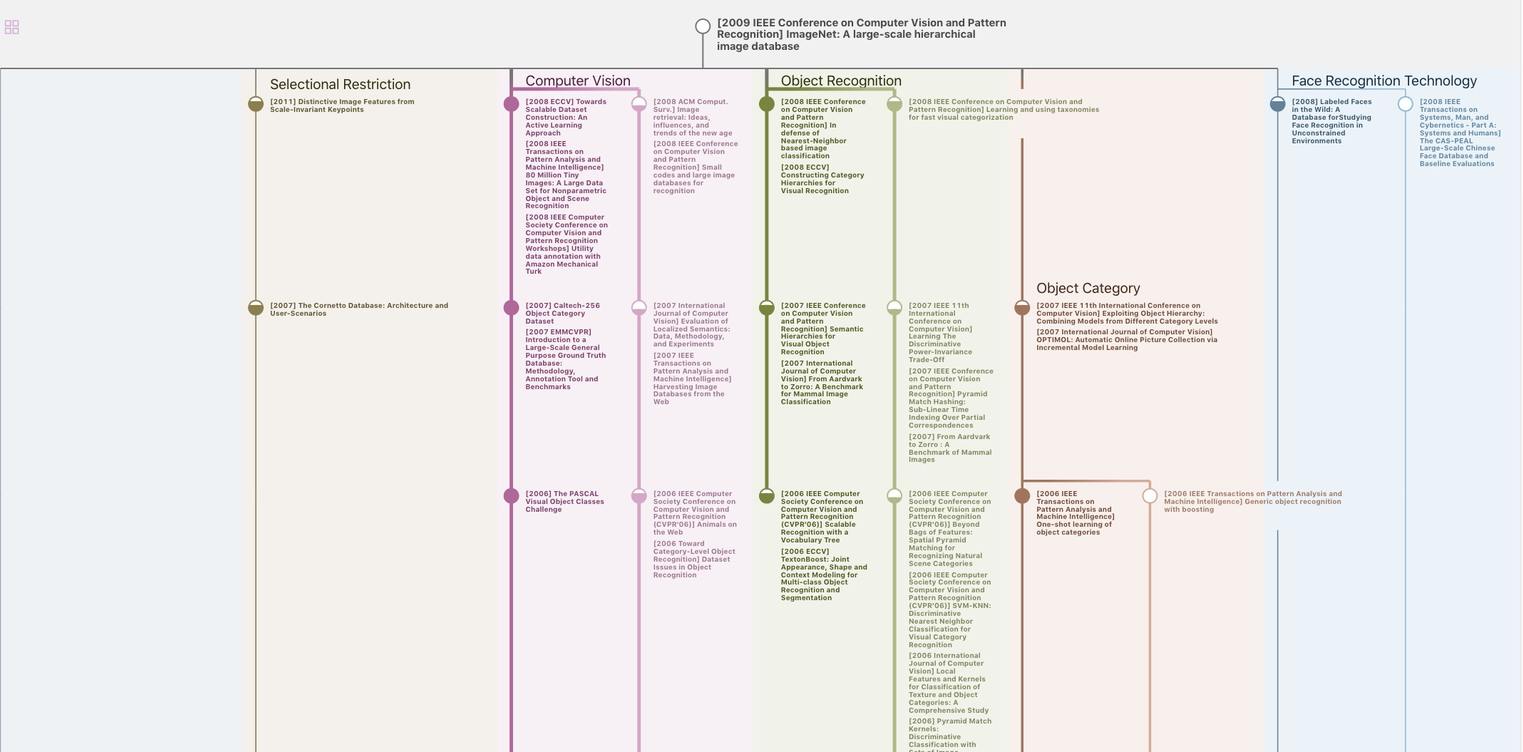
生成溯源树,研究论文发展脉络
Chat Paper
正在生成论文摘要