Short-Term Photovoltaic Power Forecasting Based on Historical Information and Deep Learning Methods
SENSORS(2022)
摘要
The accurate prediction of photovoltaic (PV) power is essential for planning power systems and constructing intelligent grids. However, this has become difficult due to the intermittency and instability of PV power data. This paper introduces a deep learning framework based on 7.5 min-ahead and 15 min-ahead approaches to predict short-term PV power. Specifically, we propose a hybrid model based on singular spectrum analysis (SSA) and bidirectional long short-term memory (BiLSTM) networks with the Bayesian optimization (BO) algorithm. To begin, the SSA decomposes the PV power series into several sub-signals. Then, the BO algorithm automatically adjusts hyperparameters for the deep neural network architecture. Following that, parallel BiLSTM networks predict the value of each component. Finally, the prediction of the sub-signals is summed to generate the final prediction results. The performance of the proposed model is investigated using two datasets collected from real-world rooftop stations in eastern China. The 7.5 min-ahead predictions generated by the proposed model can reduce up to 380.51% error, and the 15 min-ahead predictions decrease by up to 296.01% error. The experimental results demonstrate the superiority of the proposed model in comparison to other forecasting methods.
更多查看译文
关键词
Bayesian optimization algorithm,bidirectional long short-term memory,deep learning,singular spectrum analysis,short-term photovoltaic power forecasting
AI 理解论文
溯源树
样例
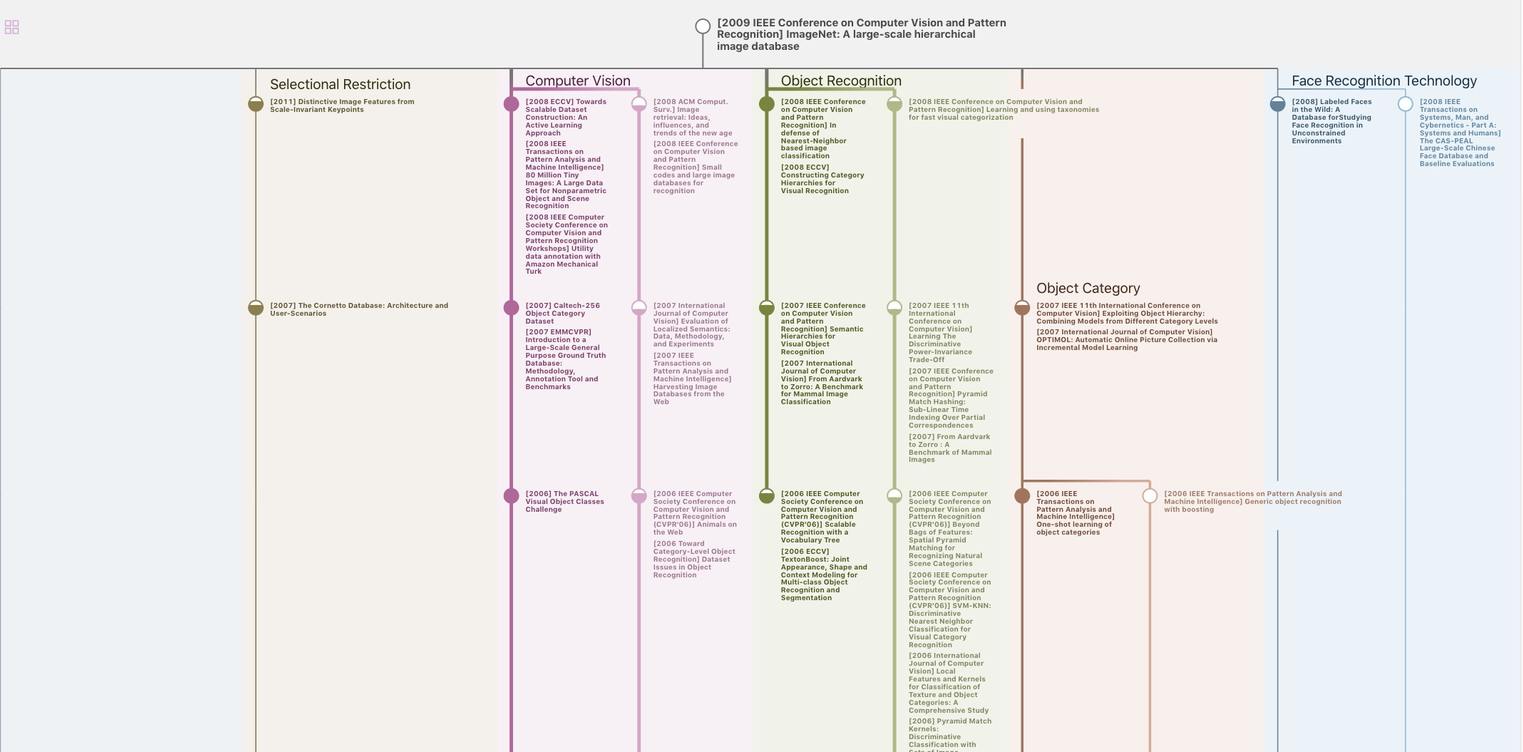
生成溯源树,研究论文发展脉络
Chat Paper
正在生成论文摘要