Aligning accumulative representations for sign language recognition
Machine Vision and Applications(2022)
摘要
Accumulative representations provide a method for representing variable-length videos with constant length features. In this study, we present aligned temporal accumulative features (ATAF), a skeleton heatmap-based feature for efficient representation and modeling of isolated sign language videos. Inspired by the movement-hold model in sign linguistics, we extract keyframes, align them using temporal transformer networks (TTNs) and extract descriptors using convolutional neural networks (CNNs). In the proposed approach, the use of aligned keyframes increases the recognition power of accumulative features as linguistically significant parts of signs are represented uniquely. Since we detect keyframes using hand movement, there can be differences from signer to signer. To overcome this challenge, ATAF has been implemented with both alignment of sampled frames and keyframe alignment approaches, using both finger speed differences and hand joint heatmaps to perform end-to-end alignment during classification. Results demonstrate that the proposed method achieves state-of-the-art recognition performance on the public BosphorusSign22k (BSign22k) dataset in combination with 3D-CNNs.
更多查看译文
关键词
Sign Language Recognition,Skeleton-Based Representation,Temporal Alignment
AI 理解论文
溯源树
样例
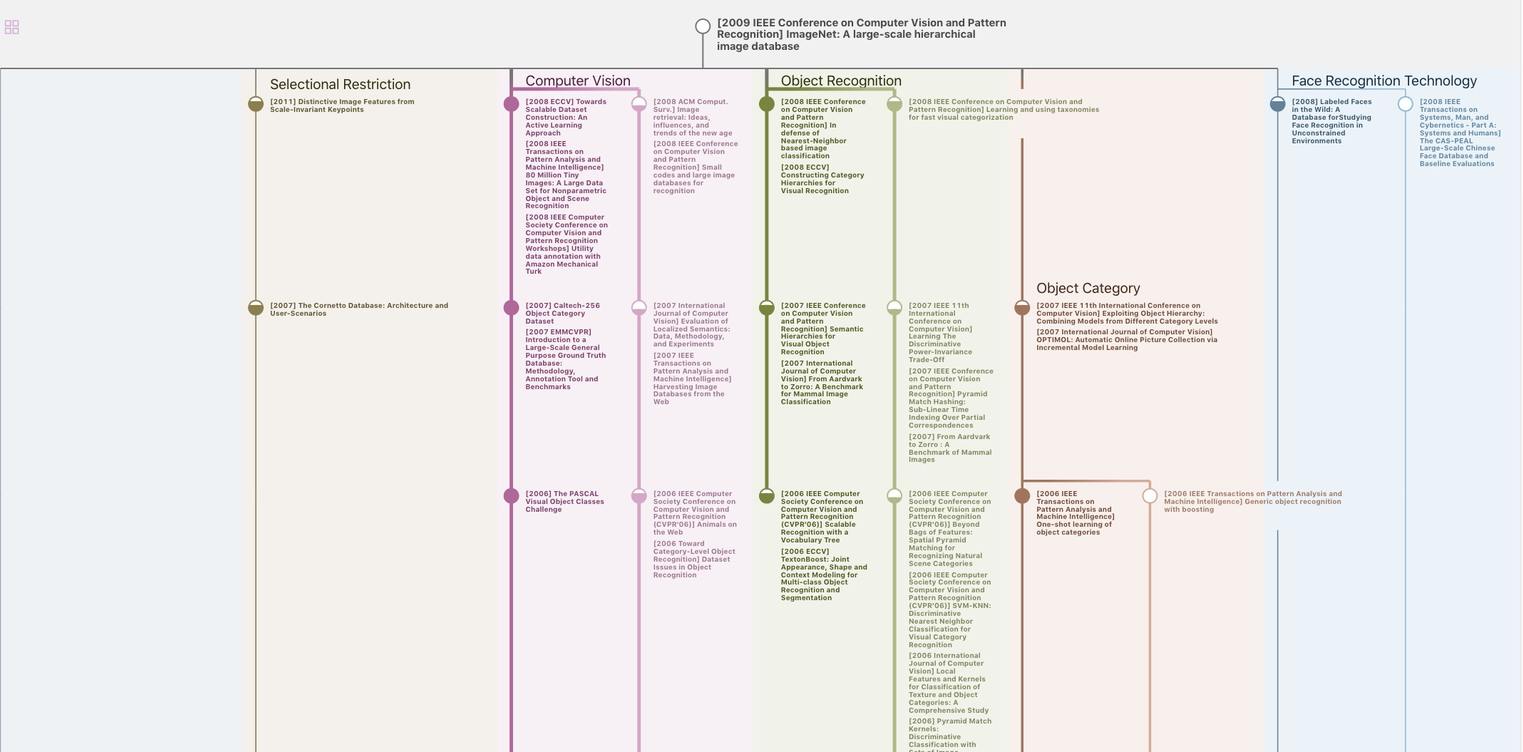
生成溯源树,研究论文发展脉络
Chat Paper
正在生成论文摘要