Gamma-ray Track Reconstruction Using Graph Neural Networks
Nuclear instruments and methods in physics research Section A, Accelerators, spectrometers, detectors and associated equipment/Nuclear instruments & methods in physics research Section A, Accelerators, spectrometers, detectors and associated equipment(2023)
摘要
Since the advent of the new generation of germanium detector arrays for low-energy nuclear physics experiments utilizing gamma-ray tracking, the challenges associated with track-reconstruction methods have been extensively studied. In the present work an approach based on recent developments in machine learning was used to address the problem. Here, a graph neural network was constructed and trained on data simulated in Geant4 in order to attempt track reconstruction of gamma rays below 1 MeV in a spherical shell geometry of pure germanium. Using a thick-shell geometry, and simulated data without energy-and position uncertainties the network achieved a reconstruction rate above 80% for complete tracks, and a combined peak-to-total value of 85% for energy spectra with four discrete peaks. For data with added noise, i.e. finite resolution in interaction-point position and energy, the corresponding peak-to-total ratio dropped to 74%. The track reconstruction was stable across multiplicities 1-10 but showed an increased error frequency in the energy range between 50 keV and 250 keV. To specifically study the complication of gamma tracks lost by out -scattering from the detector volume, a thin-shell (9 cm thickness) geometry was used together with a modified version of the GNN framework. By letting the GNN code identify and discriminate the out-scatter events, an improvement of the P/T value from 66% to 75% was found for the packed, noisy data. For the sake of comparison the new GNN model with existing gamma-ray tracking methods, a separate instance of the network was trained on slightly higher energies (up to 1.5 MeV) and multiplicities (up to 15) to evaluate 1.332 MeV photon cascade data in terms of P/T and photo-peak efficiency. The results for this GNN data set, with P/T values at 85% for single tracks and 74% for multiplicity 15, show clear promise when compared to the existing tracking methods.
更多查看译文
关键词
Gamma-ray spectroscopy,Gamma-ray tracking,Machine learning,GNN
AI 理解论文
溯源树
样例
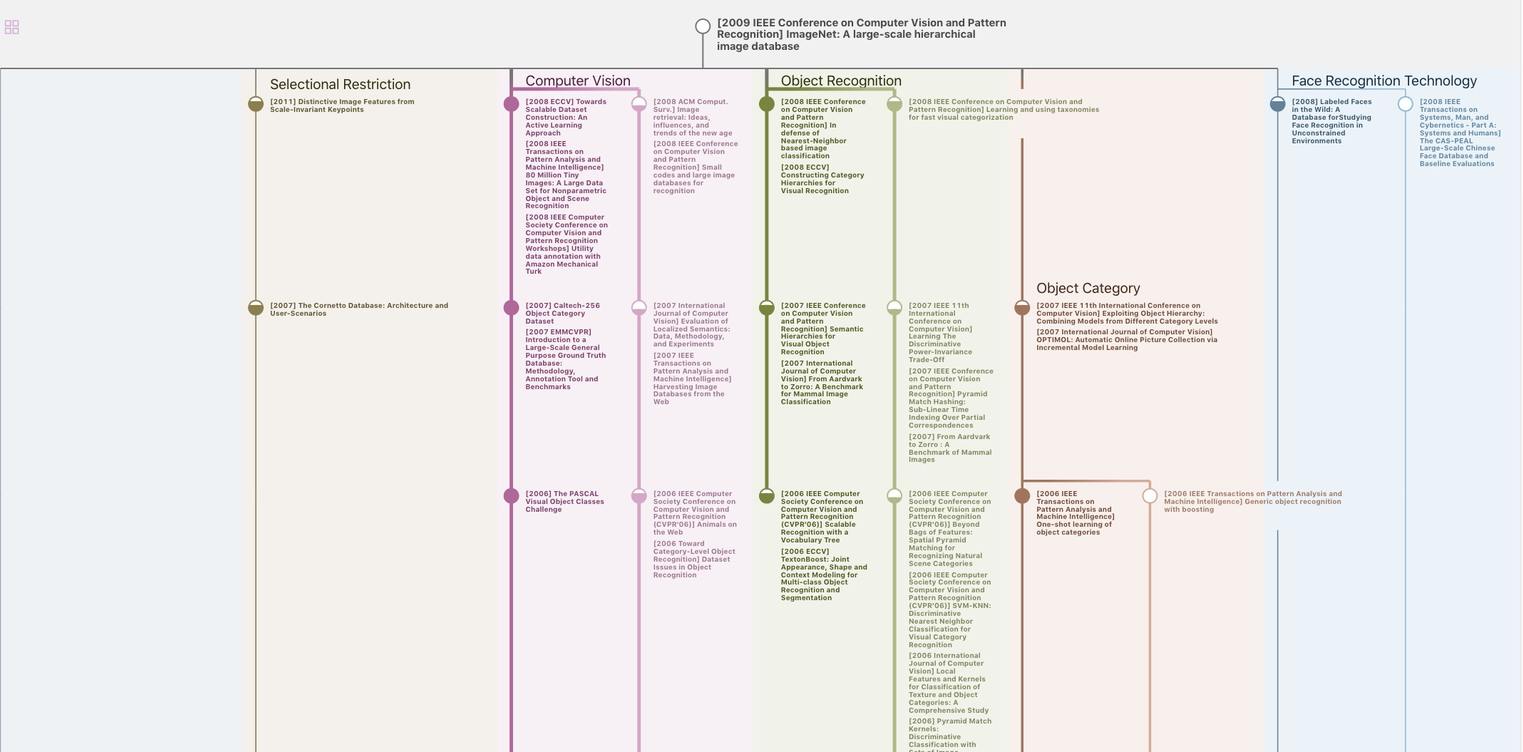
生成溯源树,研究论文发展脉络
Chat Paper
正在生成论文摘要