Robust computation of optimal transport by $\beta$-potential regularization
arxiv(2022)
摘要
Optimal transport (OT) has become a widely used tool in the machine learning field to measure the discrepancy between probability distributions. For instance, OT is a popular loss function that quantifies the discrepancy between an empirical distribution and a parametric model. Recently, an entropic penalty term and the celebrated Sinkhorn algorithm have been commonly used to approximate the original OT in a computationally efficient way. However, since the Sinkhorn algorithm runs a projection associated with the Kullback-Leibler divergence, it is often vulnerable to outliers. To overcome this problem, we propose regularizing OT with the \beta-potential term associated with the so-called $\beta$-divergence, which was developed in robust statistics. Our theoretical analysis reveals that the $\beta$-potential can prevent the mass from being transported to outliers. We experimentally demonstrate that the transport matrix computed with our algorithm helps estimate a probability distribution robustly even in the presence of outliers. In addition, our proposed method can successfully detect outliers from a contaminated dataset
更多查看译文
关键词
optimal transport,robust computation
AI 理解论文
溯源树
样例
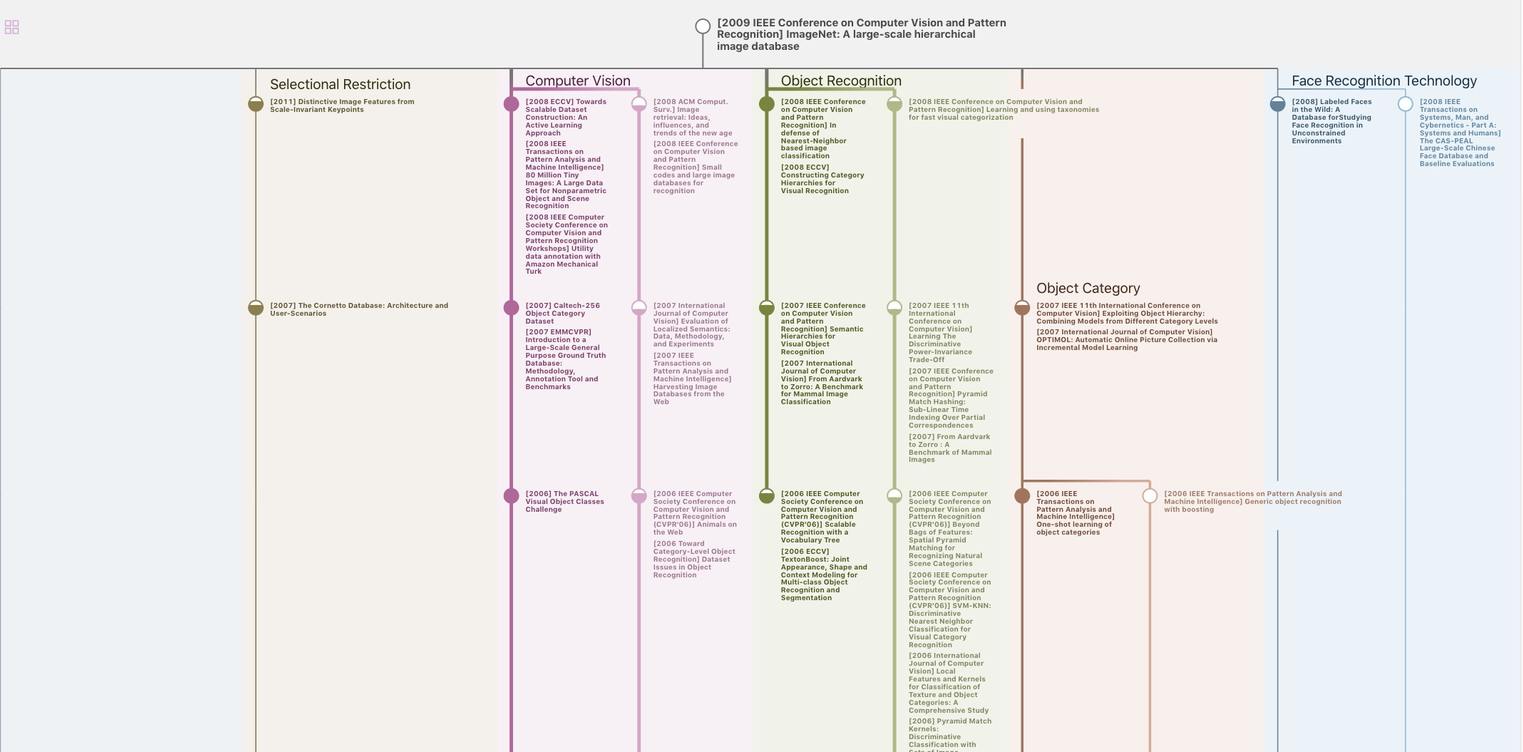
生成溯源树,研究论文发展脉络
Chat Paper
正在生成论文摘要