Sliding Cross Entropy for Self-Knowledge Distillation
Conference on Information and Knowledge Management(2022)
摘要
ABSTRACTKnowledge distillation (KD) is a powerful technique for improving the performance of a small model by leveraging the knowledge of a larger model. Despite its remarkable performance boost, KD has a drawback with the substantial computational cost of pre-training larger models in advance. Recently, a method called self-knowledge distillation has emerged to improve the model's performance without any supervision. In this paper, we present a novel plug-in approach called Sliding Cross Entropy (SCE) method, which can be combined with existing self-knowledge distillation to significantly improve the performance. Specifically, to minimize the difference between the output of the model and the soft target obtained by self-distillation, we split each softmax representation by a certain window size, and reduce the distance between sliced parts. Through this approach, the model evenly considers all the inter-class relationships of a soft target during optimization. The extensive experiments show that our approach is effective in various tasks, including classification, object detection, and semantic segmentation. We also demonstrate SCE consistently outperforms existing baseline methods.
更多查看译文
关键词
sliding cross entropy,self-knowledge
AI 理解论文
溯源树
样例
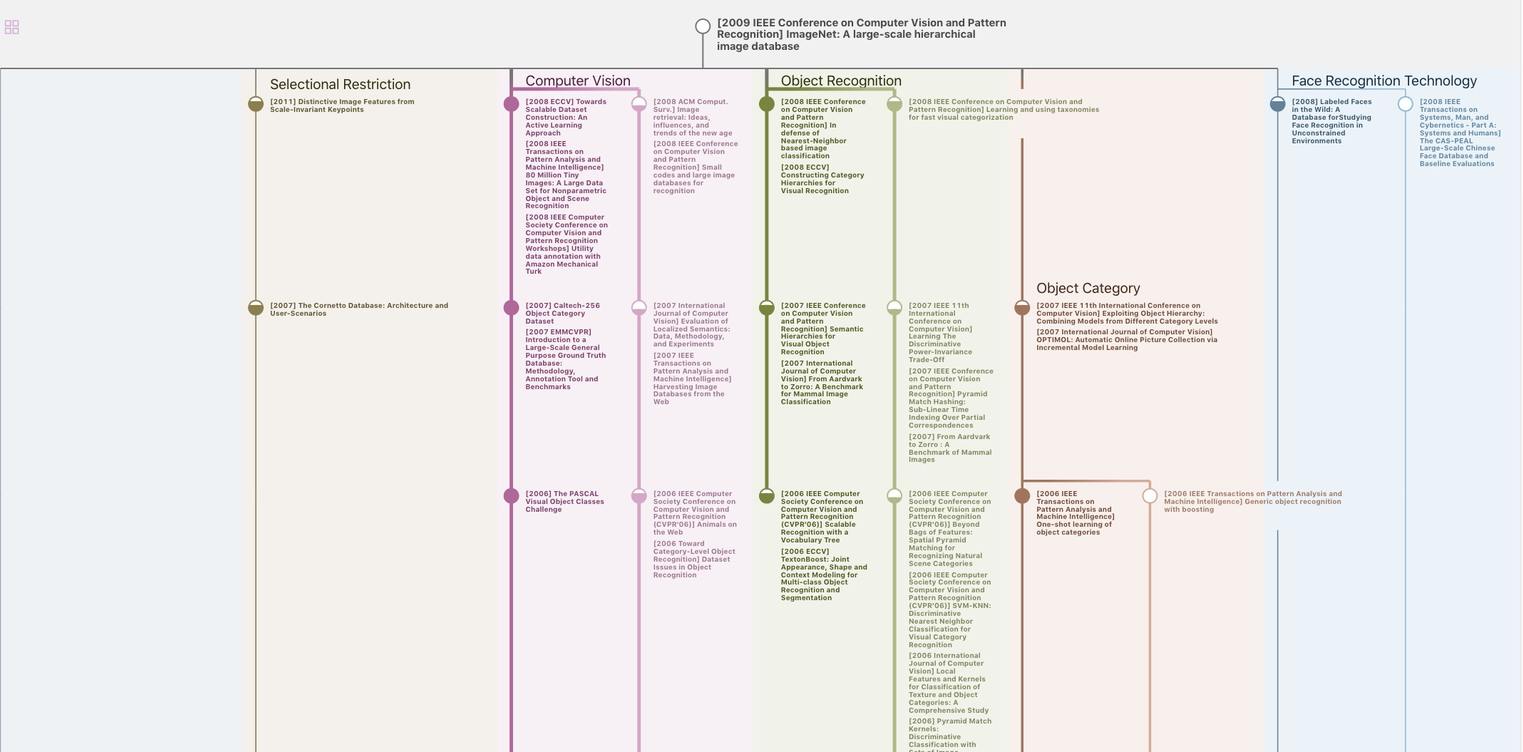
生成溯源树,研究论文发展脉络
Chat Paper
正在生成论文摘要