SWAG-Net: SemanticWord-Aware Graph Network for Temporal Video Grounding
Conference on Information and Knowledge Management(2022)
摘要
In this paper, to effectively capture non-sequential dependencies among semantic words for temporal video grounding, we propose a novel framework called Semantic Word-Aware Graph Network (SWAG-Net), which adopts graph-guided semantic word embedding in an end-to-end manner. Specifically, we define semantic word features as node features of semantic word-aware graphs and word-to-word correlations as three edge types (i.e., intrinsic, extrinsic, and relative edges) for diverse graph structures. We then apply Semantic Word-aware Graph Convolutional Networks (SWGCNs) to the graphs for semantic word embedding. For modality fusion and context modeling, the embedded features and video segment features are merged into bi-modal features, and the bimodal features are aggregated by incorporating local and global contextual information. Leveraging the aggregated features, the proposed method effectively finds a temporal boundary semantically corresponding to a sentence query in an untrimmed video. We verify that our SWAG-Net outperforms state-of-the-art methods on Charades-STA and ActivityNet Captions datasets.
更多查看译文
关键词
temporal video grounding, multimodal fusion, graph neural network, attention mechanism
AI 理解论文
溯源树
样例
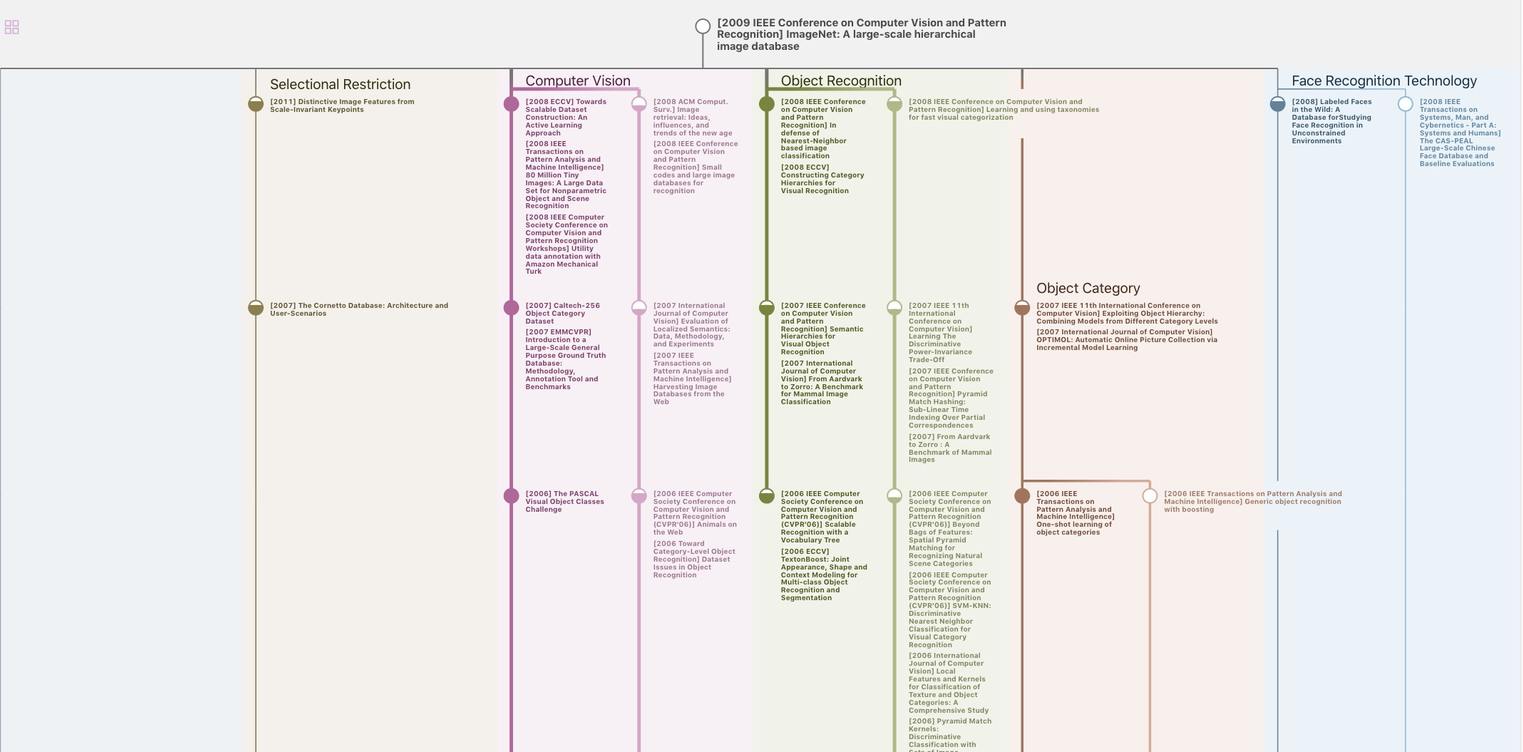
生成溯源树,研究论文发展脉络
Chat Paper
正在生成论文摘要