Data Oversampling with Structure Preserving Variational Learning
Conference on Information and Knowledge Management(2022)
摘要
ABSTRACTTraditional oversampling methods are well explored for binary and multi-class imbalanced datasets. In most cases, the data space is adapted for oversampling the imbalanced classes. It leads to various issues like poor modelling of the structure of the data, resulting in data overlapping between minority and majority classes that lead to poor classification performance of minority class(es). To overcome these limitations, we propose a novel data oversampling architecture called Structure Preserving Variational Learning (SPVL). This technique captures an uncorrelated distribution among classes in the latent space using an encoder-decoder framework. Hence, minority samples are generated in the latent space, preserving the structure of the data distribution. The improved latent space distribution (oversampled training data) is evaluated by training an MLP classifier and testing with unseen test dataset. The proposed SPVL method is applied to various benchmark datasets with i) binary and multi-class imbalance data, ii) high-dimensional data and, iii) large or small-scale data. Extensive experimental results demonstrated that the proposed SPVL technique outperforms the state-of-the-art counterparts.
更多查看译文
关键词
learning,data,structure
AI 理解论文
溯源树
样例
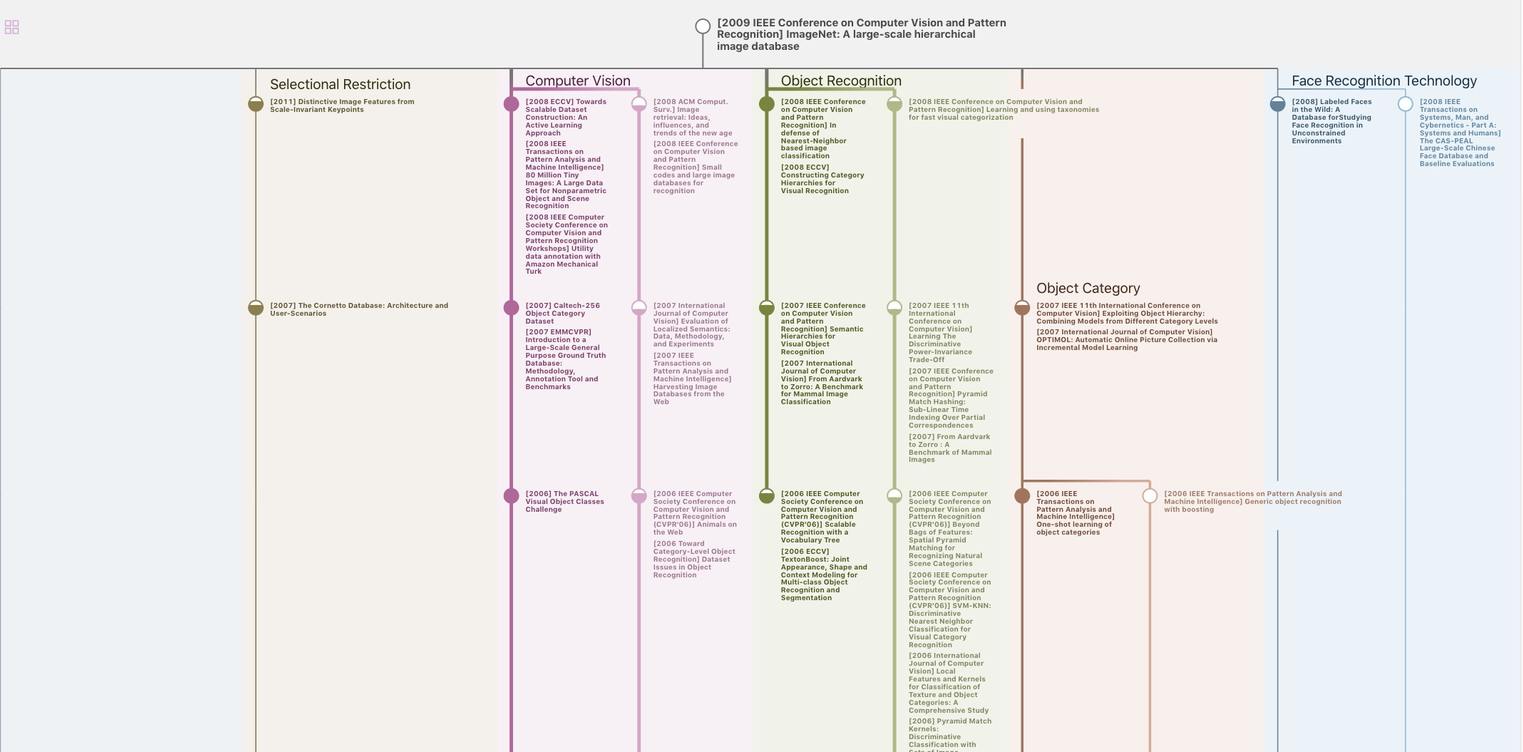
生成溯源树,研究论文发展脉络
Chat Paper
正在生成论文摘要