Bootstrapped Knowledge Graph Embedding based on Neighbor Expansion
Conference on Information and Knowledge Management(2022)
摘要
ABSTRACTMost Knowledge Graph(KG) embedding models require negative sampling to learn the representations of KG by discriminating the differences between positive and negative triples. Knowledge representation learning tasks such as link prediction are heavily influenced by the quality of negative samples. Despite many attempts, generating high-quality negative samples remains a challenge. In this paper, we propose a novel framework, Bootstrapped Knowledge graph Embedding based on Neighbor Expansion (BKENE), which learns representations of KG without using negative samples. Our model avoids using augmentation methods that can alter the semantic information when creating the two semantically similar views of KG. In particular, we generate an alternative view of KG by aggregating the information of the expanded neighbor of each node with multi-hop relation. Experimental results show that our BKENE outperforms the state-of-the-art methods for link prediction tasks.
更多查看译文
关键词
knowledge graph,neighbor expansion
AI 理解论文
溯源树
样例
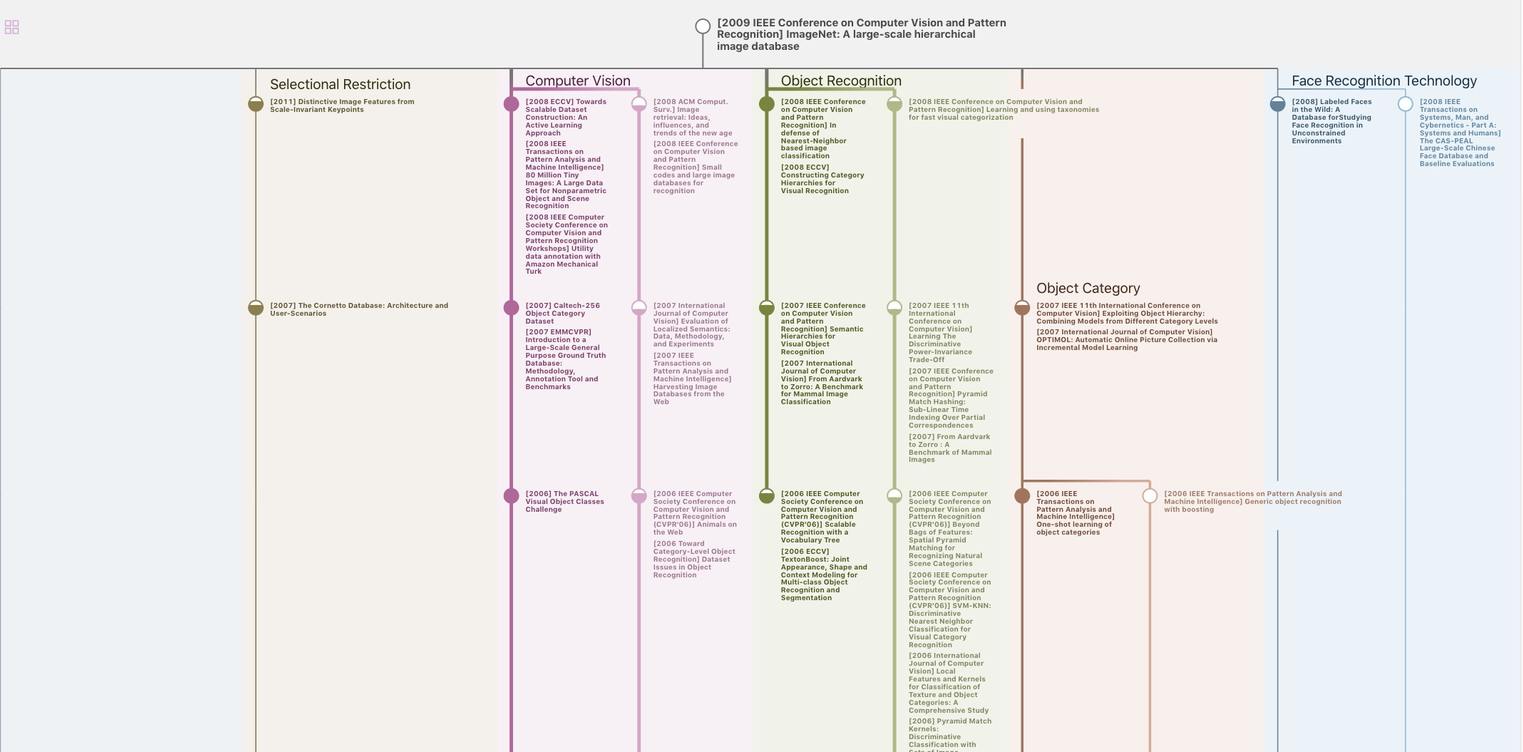
生成溯源树,研究论文发展脉络
Chat Paper
正在生成论文摘要