Query-Aware Sequential Recommendation
Conference on Information and Knowledge Management(2022)
摘要
ABSTRACTSequential recommenders aim to capture users' dynamic interests from their historical action sequences, but remain challenging due to data sparsity issues, as well as the noisy and complex relationships among items in a sequence. Several approaches have sought to alleviate these issues using side-information, such as item content (e.g., images), action types (e.g., click, purchase). While useful, we argue one of the main contextual signals is largely ignored-namely users' queries. When users browse and consume products (e.g., music, movies), their sequential interactions are usually a combination of queries, clicks (etc.). Most interaction datasets discard queries, and corresponding methods simply model sequential behaviors over items and thus ignore this critical context of user interactions. In this work, we argue that user queries should be an important contextual cue for sequential recommendation. First, we propose a new query-aware sequential recommendation setting, i.e. incorpo- rating explicit user queries to model users' intent. Next, we propose a model, namely Query-SeqRec, to (1) incorporate query information into user behavior sequences; and (2) improve model generalization ability using query-item co-occurrence information. Last, we demonstrate the effectiveness of incorporating query features in sequential recommendation on three datasets.1
更多查看译文
关键词
personalization, sequential recommendation
AI 理解论文
溯源树
样例
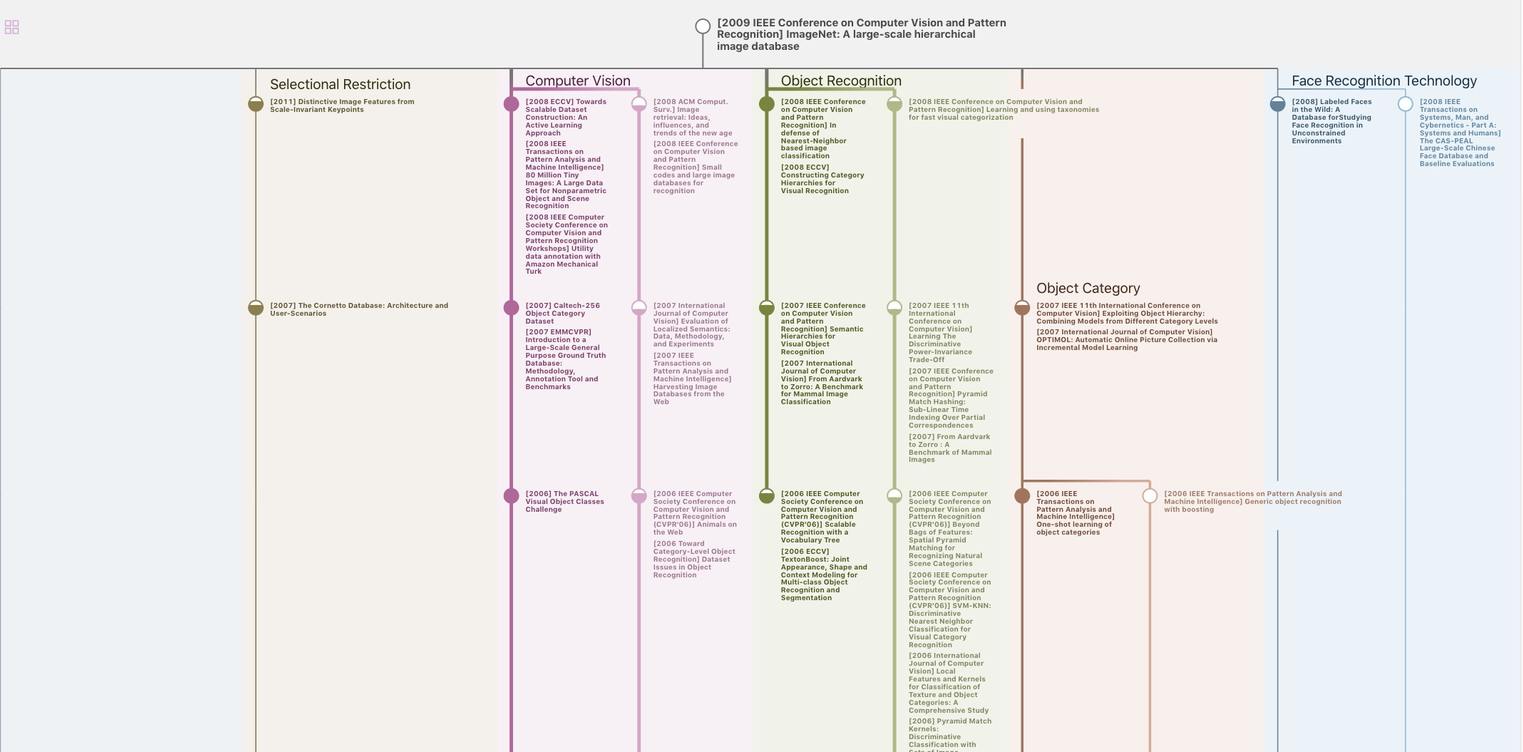
生成溯源树,研究论文发展脉络
Chat Paper
正在生成论文摘要