Numerical Feature Representation with Hybrid N-ary Encoding
Conference on Information and Knowledge Management(2022)
摘要
ABSTRACTNumerical features (e.g., statistical features) are widely used in recommender systems and online advertising. Existing approaches for numerical feature representation in industry are primarily based on discretization. However, hard-discretization based methods (e.g., Equal Distance Discretization) are deficient in continuity while soft-discretization based methods (e.g., AutoDis) lack discriminability. To emphasize both continuity and discriminability for numerical features, we propose an end-to-end representation learning framework named NaryDis. Specifically, NaryDis first leverages hybrid n-ary encoding as an automatic discretization module to generate hybrid-grained discretization results (multiple encoded sequences). Each position of the encoded sequence is assigned with a positional embedding and an intra-ary attention network is leveraged to aggregate the positional embeddings for obtaining ary-wise representations. Then an inter-ary attention is adopted to assemble these representations, which are further constrained by a self-supervised regularization module. Comprehensive experiments on two public datasets are conducted to show the superiority and compatibility of NaryDis. Besides, we deeply investigate the properties of continuity and discriminability. Moreover, we further verify the effectiveness of NaryDis on a large-scale industrial advertisement dataset.
更多查看译文
关键词
representation,feature,hybrid
AI 理解论文
溯源树
样例
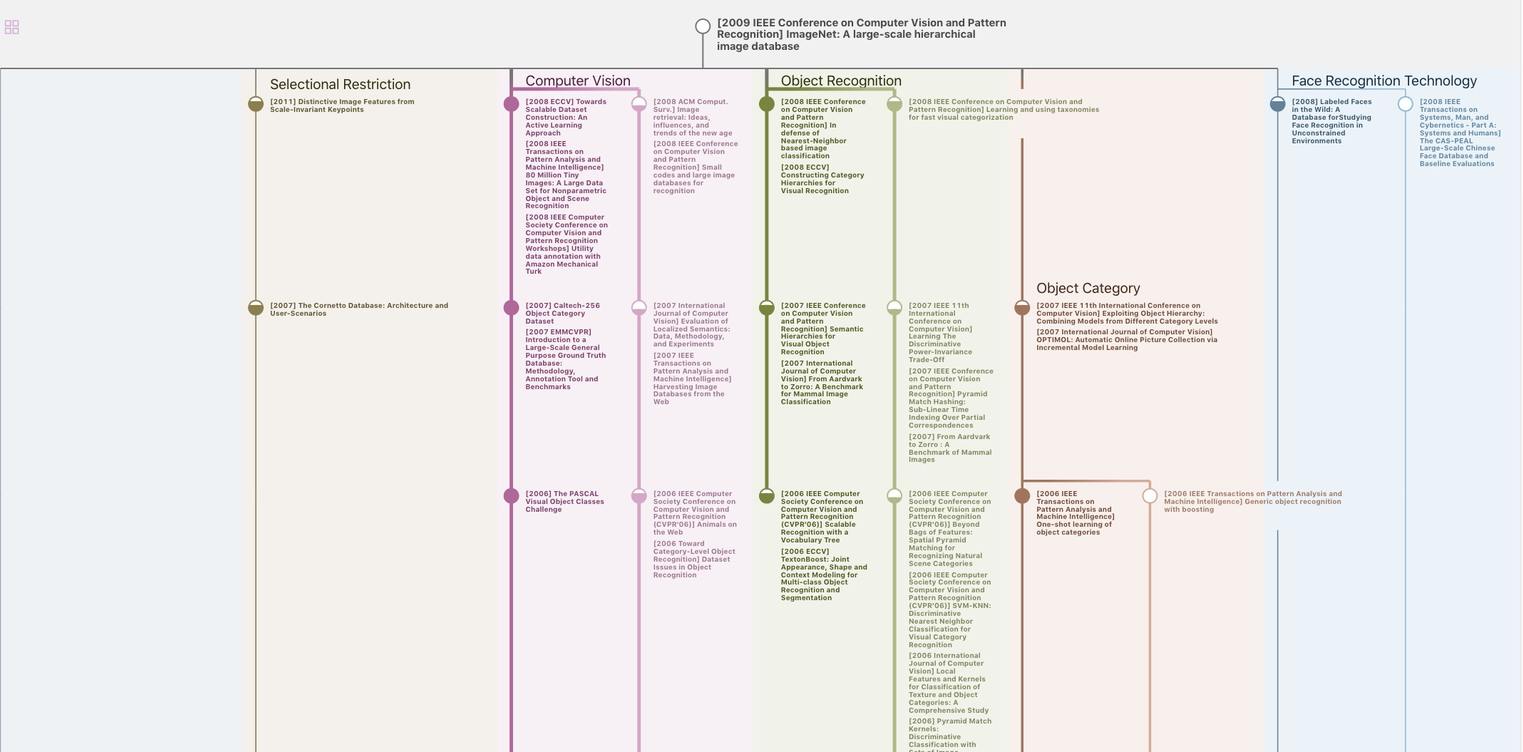
生成溯源树,研究论文发展脉络
Chat Paper
正在生成论文摘要