Transformer-Based Physiological Feature Learning for Multimodal Analysis of Self-Reported Sentiment
Multimodal Interfaces and Machine Learning for Multimodal Interaction(2022)
摘要
ABSTRACT One of the main challenges in realizing dialog systems is adapting to a user’s sentiment state in real time. Large-scale language models, such as BERT, have achieved excellent performance in sentiment estimation; however, the use of only linguistic information from user utterances in sentiment estimation still has limitations. In fact, self-reported sentiment is not necessarily expressed by user utterances. To mitigate the issue that the true sentiment state is not expressed as observable signals, psychophysiology and affective computing studies have focused on physiological signals that capture involuntary changes related to emotions. We address this problem by efficiently introducing time-series physiological signals into a state-of-the-art language model to develop an adaptive dialog system. Compared with linguistic models based on BERT representations, physiological long short-term memory (LSTM) models based on our proposed physiological signal processing method have competitive performance. Moreover, we extend our physiological signal processing method to the Transformer language model and propose the Time-series Physiological Transformer (TPTr), which captures sentiment changes based on both linguistic and physiological information. In ensemble models, our proposed methods significantly outperform the previous best result (p < 0.05).
更多查看译文
关键词
Time-Series Processing, Physiological Signals, Sentiment Analysis
AI 理解论文
溯源树
样例
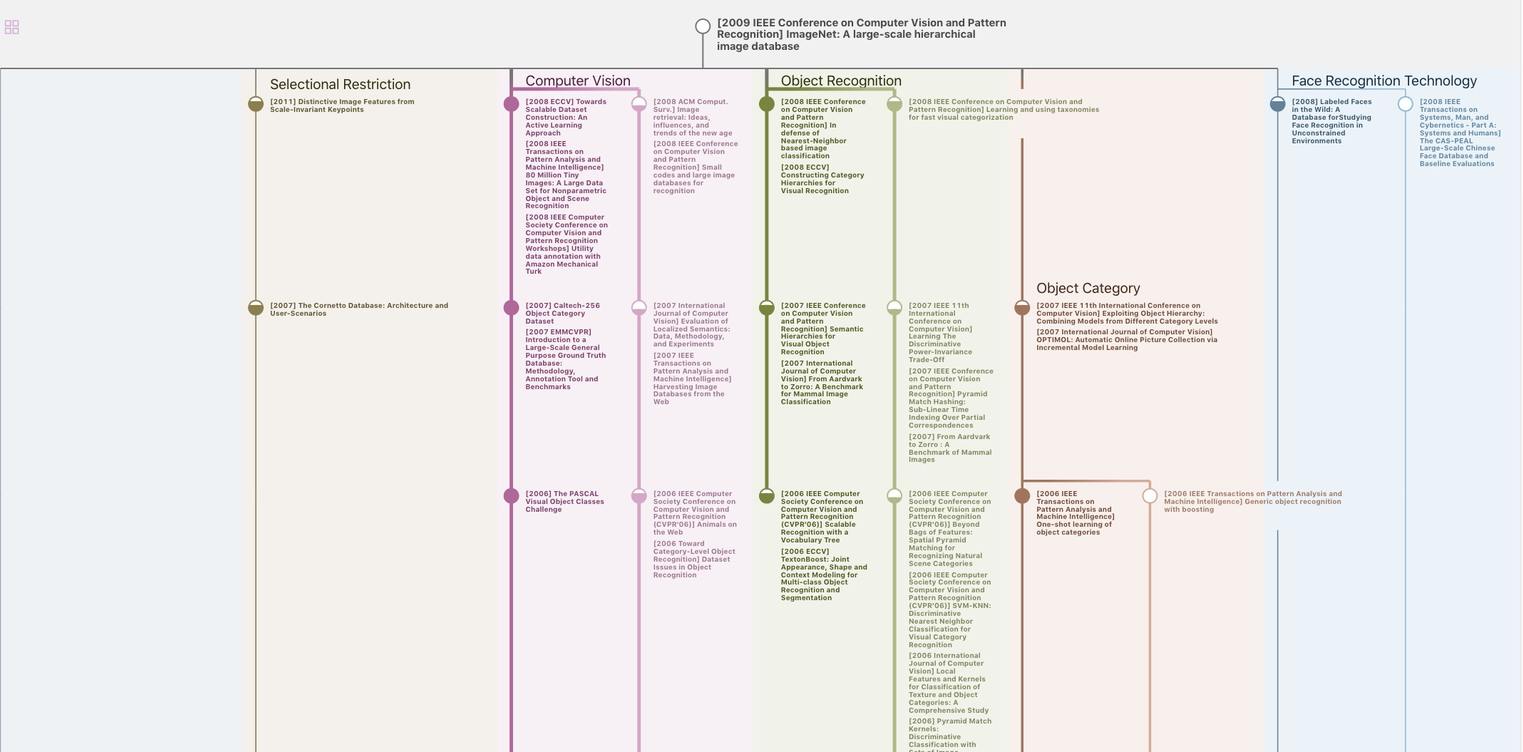
生成溯源树,研究论文发展脉络
Chat Paper
正在生成论文摘要