Contextual Tuning of Model Predictive Control for Autonomous Racing
2022 IEEE/RSJ International Conference on Intelligent Robots and Systems (IROS)(2022)
摘要
Learning-based model predictive control has been widely applied in autonomous racing to improve the closedloop behaviour of vehicles in a data-driven manner. When environmental conditions change, e.g., due to rain, often only the predictive model is adapted, but the controller parameters are kept constant. However, this can lead to suboptimal behaviour. In this paper, we address the problem of data-efficient controller tuning, adapting both the model and objective simultaneously. The key novelty of the proposed approach is that we leverage a learned dynamics model to encode the environmental condition as a so-called context. This insight allows us to employ contextual Bayesian optimization to efficiently transfer knowledge across different environmental conditions. Consequently, we require fewer data to find the optimal controller configuration for each context. The proposed framework is extensively evaluated with more than 3'000 laps driven on an experimental platform with 1:28 scale RC race cars. The results show that our approach successfully optimizes the lap time across different contexts requiring fewer data compared to other approaches based on standard Bayesian optimization.
更多查看译文
关键词
autonomous racing,closed-loop behaviour,contextual Bayesian optimization,contextual tuning,data-driven manner,data-efficient controller tuning,environmental condition,learned dynamics model,model predictive control,optimal controller configuration,predictive model,RC race cars,suboptimal behaviour
AI 理解论文
溯源树
样例
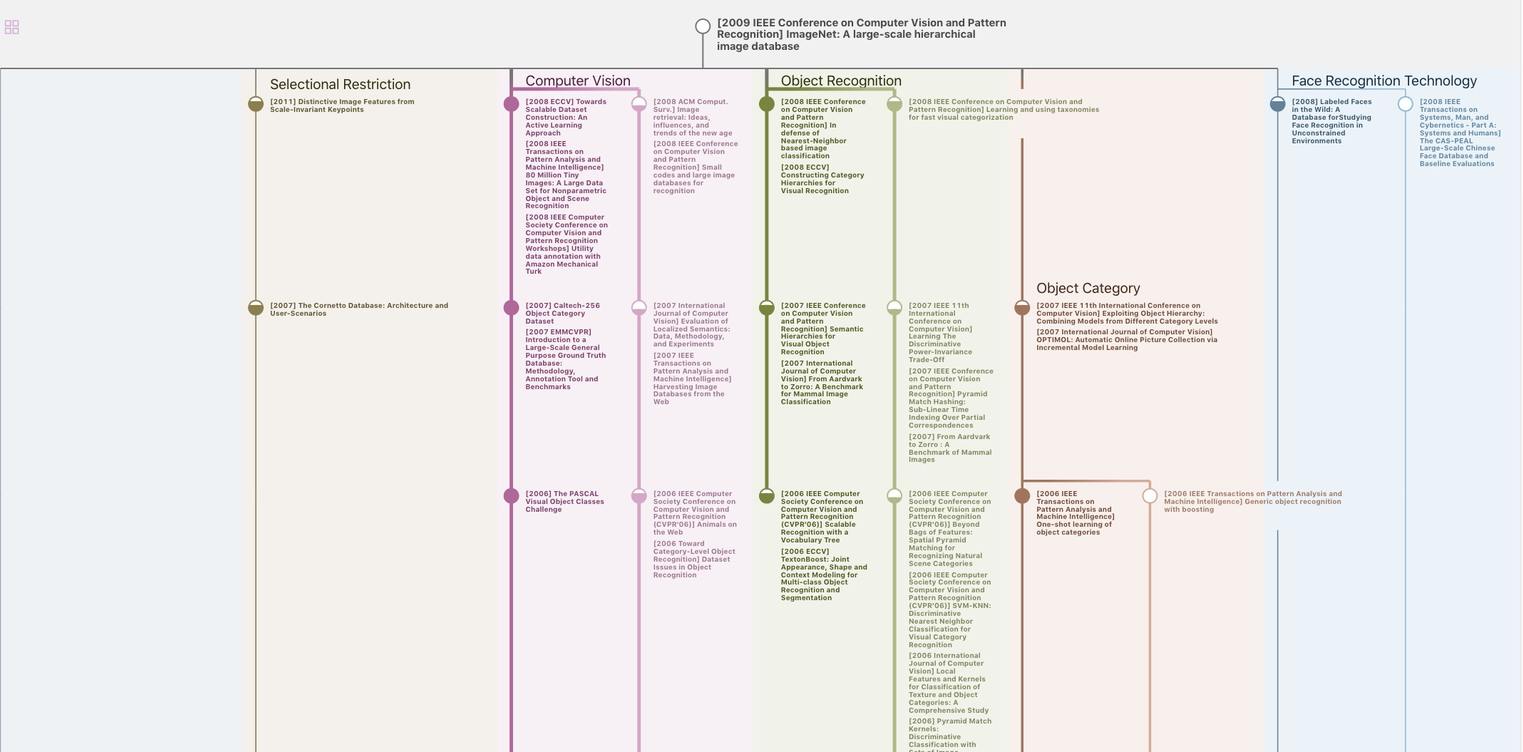
生成溯源树,研究论文发展脉络
Chat Paper
正在生成论文摘要