Improving 3D Markerless Pose Estimation of Animals in the Wild using Low-Cost Cameras
2022 IEEE/RSJ International Conference on Intelligent Robots and Systems (IROS)(2022)
摘要
Tracking the 3D motion of agile animals in the wild will enable new insight into the design of robotic controllers. However, in-field 3D pose estimation of high-speed wildlife such as cheetahs is still a challenge [1]. In this work, we aim to solve two of these challenges: unnatural pose estimates during highly occluded sequences and synchronization error between multi-view data. We expand on our previous Full Trajectory Estimation (FTE) method with two significant additions: Pairwise FTE (PW-FTE) and Shutter-delay FTE (SD-FTE). The PW-FTE expands on image-dependent pairwise terms, produced by a convolutional neural network (CNN), to infer occluded 2D keypoints, while SD-FTE uses shutter delay estimation to correct the synchronization error. Lastly, we combine both methods into PW-SD-FTE and perform a quantitative and qualitative analysis on a subset of AcinoSet, the video dataset of rapid and agile motions of cheetahs. We found that SD-FTE has significant benefits in tracking the position of the cheetah in the world frame, while PW-FTE provided a more robust 3D pose estimate during events of high occlusion. The PW-SDFTE was found to retain both advantages, resulting in an improved baseline for AcinoSet. Code and data can be found at https://github.com/African-Robotics-Unit/AcinoSet/tree/pw_sd_fte.
更多查看译文
关键词
3d markerless pose estimation,animals,low-cost low-cost,wild
AI 理解论文
溯源树
样例
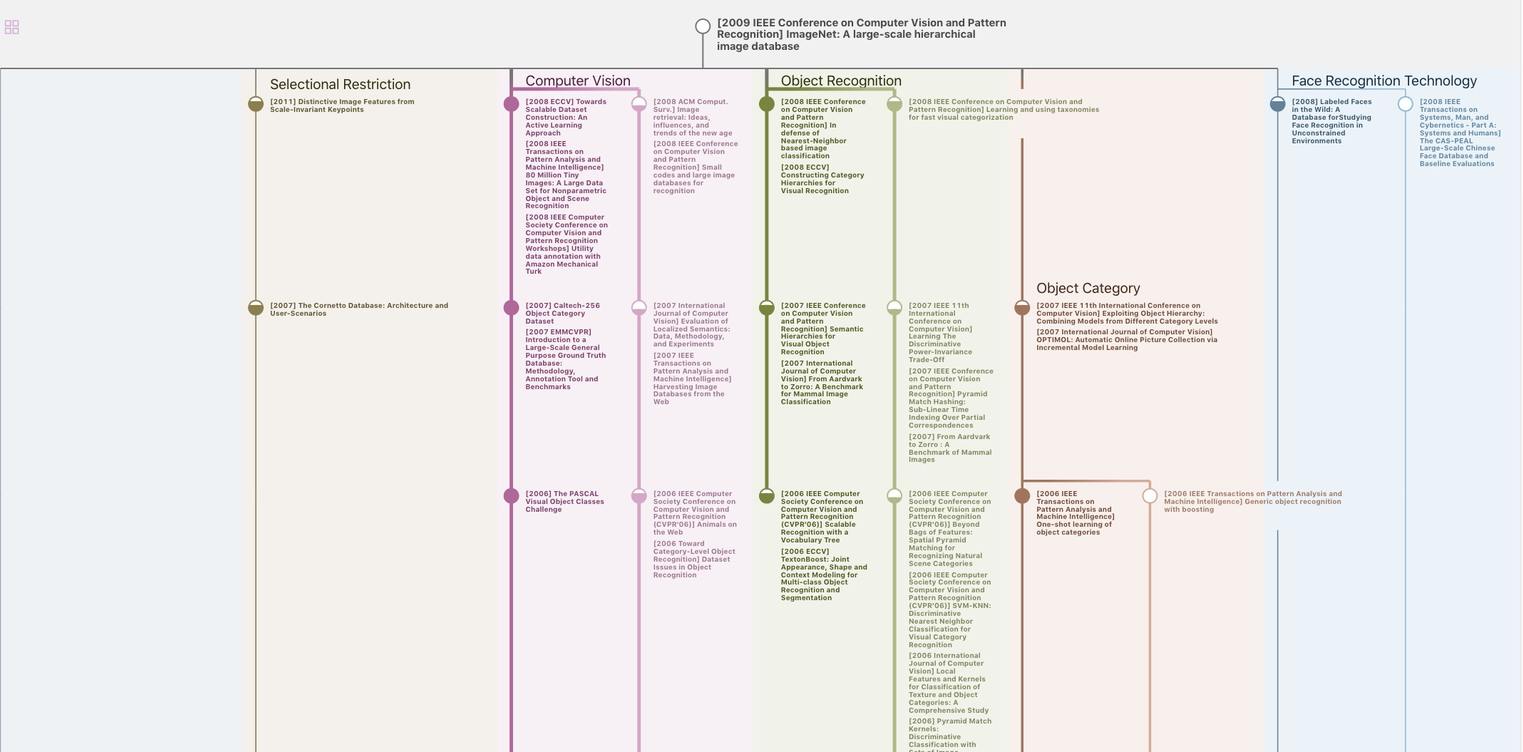
生成溯源树,研究论文发展脉络
Chat Paper
正在生成论文摘要