Conditional Patch-Based Domain Randomization: Improving Texture Domain Randomization Using Natural Image Patches
2022 IEEE/RSJ International Conference on Intelligent Robots and Systems (IROS)(2022)
摘要
Using Domain Randomized synthetic data for training deep learning systems is a promising approach for addressing the data and the labeling requirements for supervised techniques to bridge the gap between simulation and the real world. We propose a novel approach for generating and applying class-specific Domain Randomization textures by using randomly cropped image patches from real-world data. In evaluation against the current Domain Randomization texture application techniques, our approach outperforms the highest performing technique by 4.94 AP and 6.71 AP when solving object detection and semantic segmentation tasks on the YCB-M [1] real-world robotics dataset. Our approach is a fast and inexpensive way of generating Domain Randomized textures while avoiding the need to handcraft texture distributions currently being used.
更多查看译文
关键词
texture domain randomization,natural image patches,domain randomization,patch-based
AI 理解论文
溯源树
样例
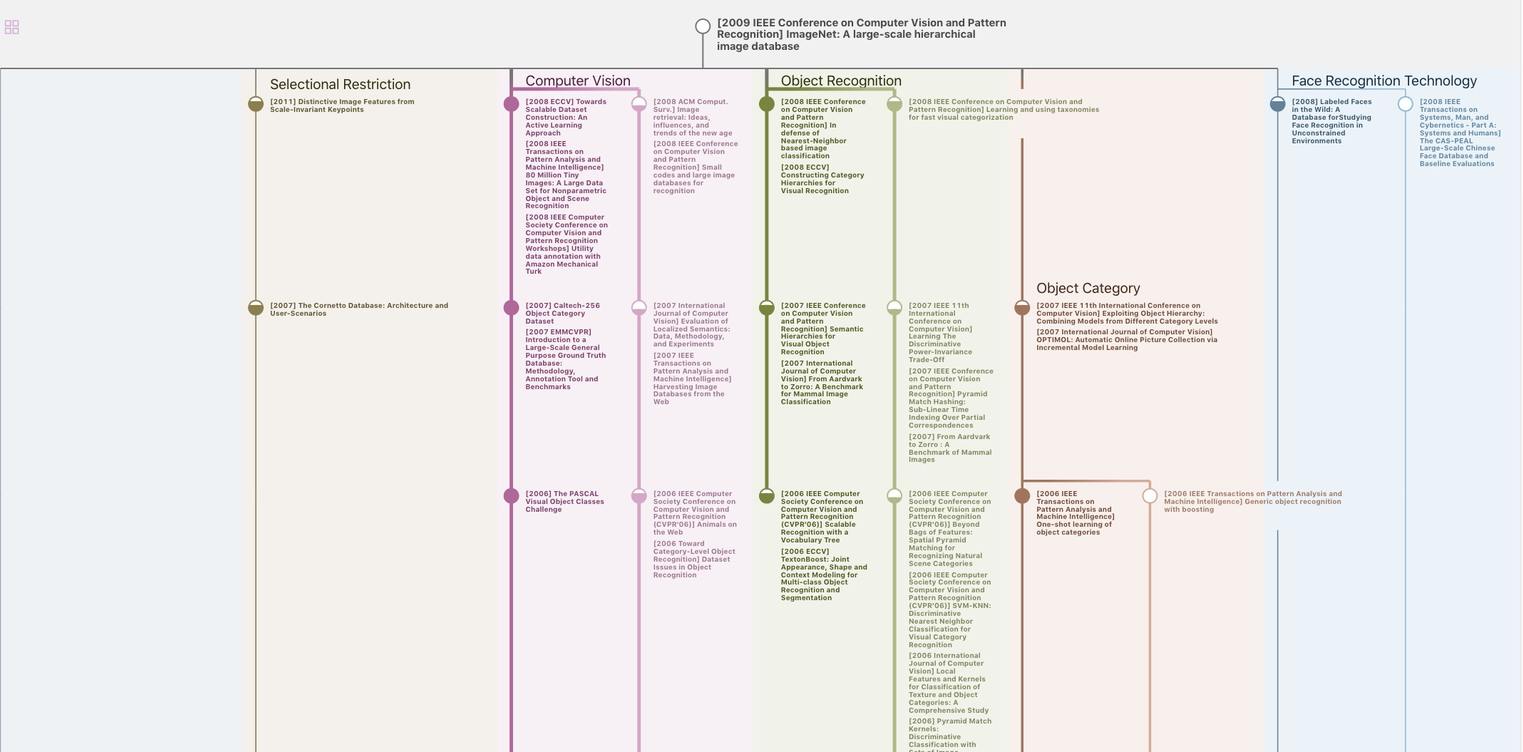
生成溯源树,研究论文发展脉络
Chat Paper
正在生成论文摘要