Learned Depth Estimation of 3D Imaging Radar for Indoor Mapping.
2022 IEEE/RSJ International Conference on Intelligent Robots and Systems (IROS)(2022)
摘要
3D imaging radar offers robust perception capability through visually demanding environments due to the unique penetrative and reflective properties of millimeter waves (mmWave). Current approaches for 3D perception with imaging radar require knowledge of environment geometry, accumulation of data from multiple frames for perception, or access to between-frame motion. Imaging radar presents an additional difficulty due to the complexity of its data representation. To address these issues, and make imaging radar easier to use for downstream robotics tasks, we propose a learning-based method that regresses radar measurements into cylindrical depth maps using LiDAR supervision. Due to the limitation of the regression formulation, directions where the radar beam could not reach will still generate a valid depth. To address this issue, our method additionally learns a 3D filter to remove those pixels. Experiments show that our system generates visually accurate depth estimation. Furthermore, we confirm the overall ability to generalize in the indoor scene using the estimated depth for probabilistic occupancy mapping with ground truth trajectory. The code and model will be released 1 1 https://github.com/rpl-cmu/learned-depth-imaging-radar.
更多查看译文
关键词
3D filter,3D imaging radar,3D perception,cylindrical depth maps,depth estimation,LiDAR supervision,millimeter waves,radar beam,radar measurements,regression formulation
AI 理解论文
溯源树
样例
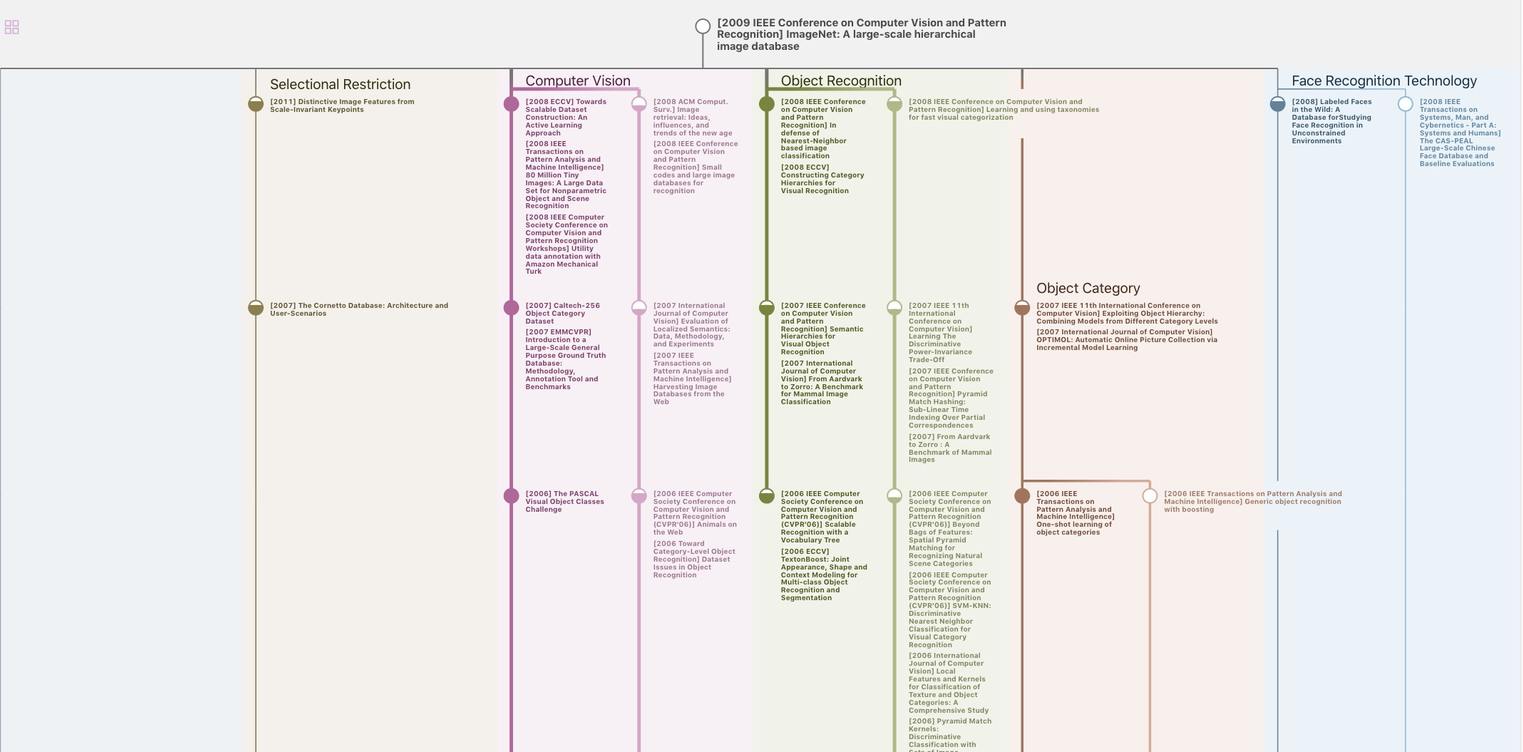
生成溯源树,研究论文发展脉络
Chat Paper
正在生成论文摘要