SESR: Self-Ensembling Sim-to-Real Instance Segmentation for Auto-Store Bin Picking
2022 IEEE/RSJ International Conference on Intelligent Robots and Systems (IROS)(2022)
摘要
Instance segmentation is an important task for supporting robotic grasping in auto-store scenarios. Accurate segmentation usually relies on the quantity and quality of available annotated training data. However, it requires tremendous cost to obtain these labels. In this work, without requiring any human annotations on real data, our proposed self-ensembling sim-to-real network, namely SESR, is able to generate precise instance masks for a wide variety of supermarket goods. We design our SESR with a teacher model and a student model trained with a self-ensembling strategy. We adopt different levels of consistency to bridge the sim-to-real gap and boost the model generalization ability. Also, we compile an auto-store bin-picking dataset covering various goods. Extensive experiments on both unseen scenarios and unseen objects validate the effectiveness and superiority of our method over others, and the robot arm demonstrations further show that our segmentation results can support real-time auto-store bin picking.
更多查看译文
关键词
auto-store bin-picking dataset,human annotations,instance segmentation,model generalization ability,real-time auto-store bin picking,robot arm demonstration,robotic grasping,self-ensembling sim-to-,self-ensembling strategy,SESR,sim-to-real gap,sim-to-real network,student model,supermarket goods,teacher model
AI 理解论文
溯源树
样例
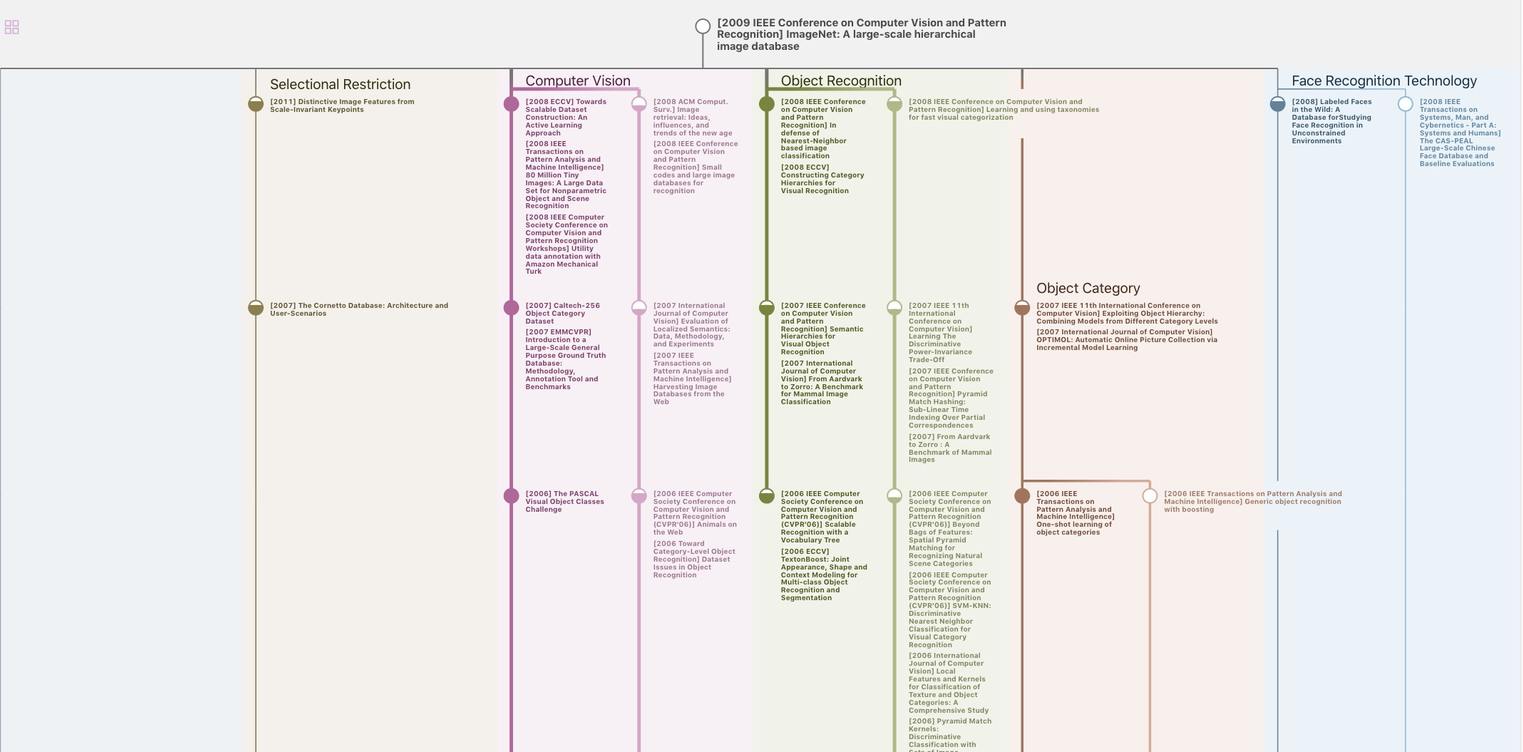
生成溯源树,研究论文发展脉络
Chat Paper
正在生成论文摘要