Enhancing Solar Energy Forecast Using Multi-Column Convolutional Neural Network and Multipoint Time Series Approach.
Remote. Sens.(2023)
摘要
The rapid expansion of solar industries presents unknown technological challenges. A dedicated and suitable energy forecast is an effective solution for the daily dispatching and production of the electricity grid. The traditional forecast technique uses weather and plant parameters as the model information. Nevertheless, these are insufficient to consider problematic weather variability and the various plant characteristics in the actual field. Considering the above facts and inspired by the excellent implementation of the multi-column convolutional neural network (MCNN) in image processing, we developed a novel approach for forecasting solar energy by transforming multipoint time series (MT) into images for the MCNN to examine. We first processed the data to convert the time series solar energy into image matrices. We observed that the MCNN showed a preeminent response under a ground-based high-resolution spatial–temporal image matrix with a 0.2826% and 0.5826% RMSE for 15 min-ahead forecast under clear (CR) and cloudy (CD) conditions, respectively. Our process was performed on the MATLAB deep learning platform and tested on CR and CD solar energy conditions. The excellent execution of the suggested technique was compared with state-of-the-art deep neural network solar forecasting techniques.
更多查看译文
关键词
convolutional neural network (CNN),multi-column convolutional neural network (MCNN) multipoint approach,solar generation forecast
AI 理解论文
溯源树
样例
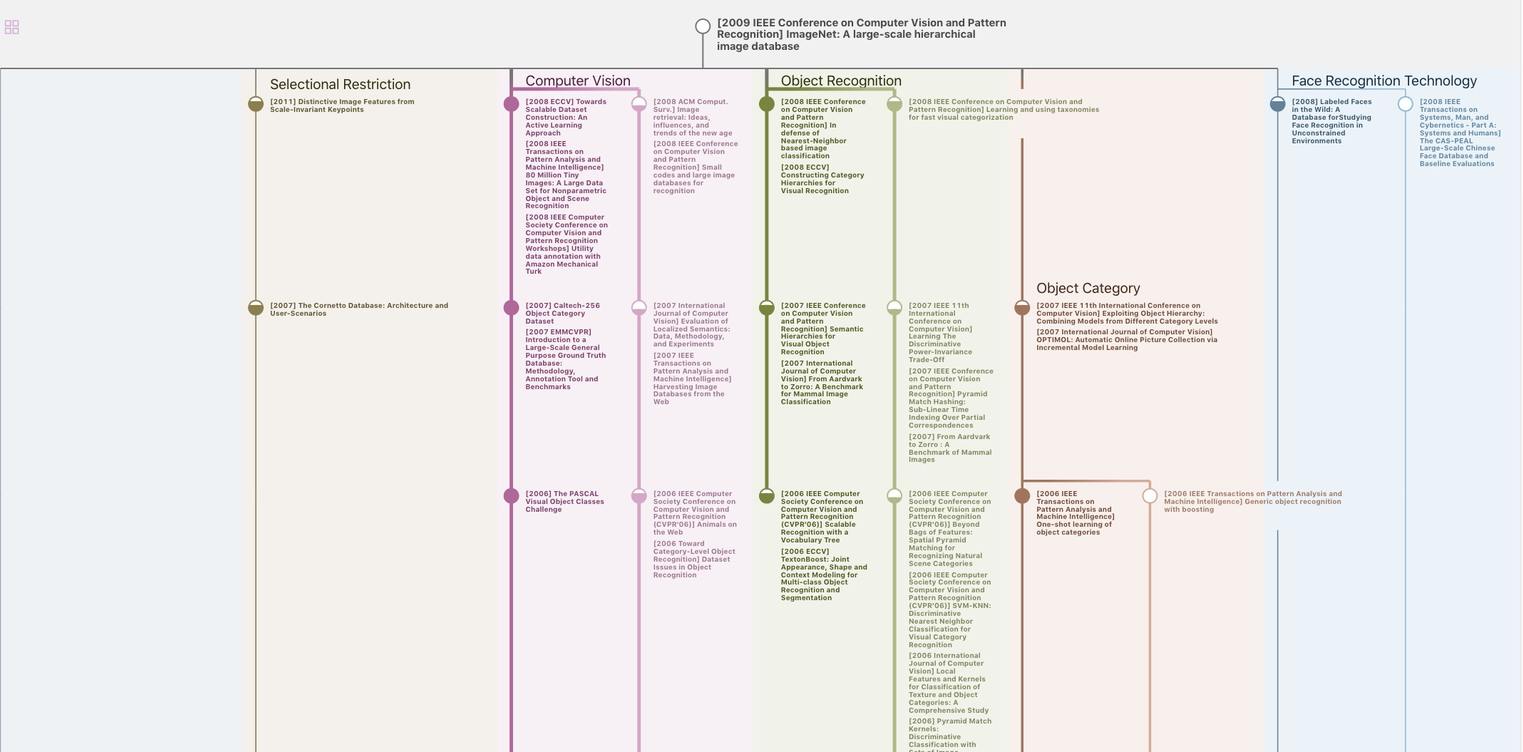
生成溯源树,研究论文发展脉络
Chat Paper
正在生成论文摘要