Feature generation and multi-sequence fusion based deep convolutional network for breast tumor diagnosis with missing MR sequences
Biomedical Signal Processing and Control(2023)
摘要
In breast tumor diagnostic tasks, joint analysis of multiple sequences of MRI can improve the accuracy of diagnosis. More and more studies focus on the correlation between sequences and try applying multiple MRI sequences to computer-aided diagnosis. The deep neural network plays a vital role in this process because of its good feature extraction ability. However, the inevitable presence of missing or unavailable sequences in clinical can lead to degraded performance or even failure of multi-input networks. The key problem solved in this paper is to ensure the diagnostic accuracy of the network despite the presence of missing input sequences. A new breast tumor diagnosis deep learning method, ESF-NET, is proposed. First, ESF-NET combines the attention mechanism for adversarial learning to obtain the potential mapping and relationship between sequences and completes the generation of features for the missing sequences. Then the extended sequence fusion module with different fusion strategies is designed, which makes the final fusion results benefit from the feature enhancement resulting from all fusion strategies. These strategies can be adaptively weighted. We apply EST-NET to a dataset of MRI images of 98 women at high risk of breast cancer, including 33 benign and 65 malignant lesions. Each patient data contains several two-dimensional slices with different axial planes, the dataset consists of 2245 slices. In the presence of sequence missing, ESF-NET achieves a diagnostic accuracy of 85.61% at the slice level and 89.66% at the patient level, an improvement of 8.39% compared to using only a single sequence.
更多查看译文
关键词
Multi-sequence MRI,Feature fusion,Breast tumor diagnosis,Feature generation,Deep learning
AI 理解论文
溯源树
样例
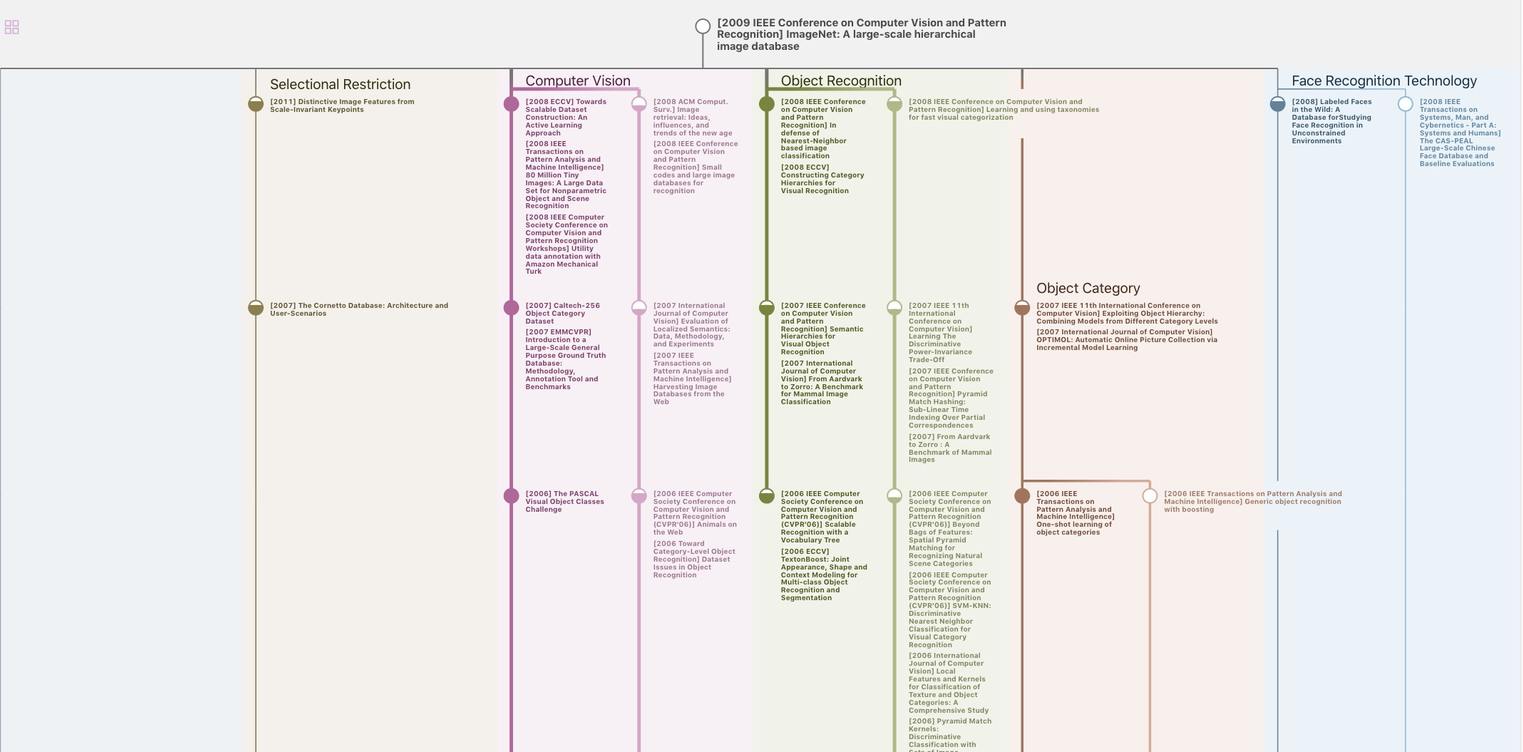
生成溯源树,研究论文发展脉络
Chat Paper
正在生成论文摘要