Head motion correction shapes functional network estimates: evidence from healthy and Parkinson’s disease cohorts
biorxiv(2022)
摘要
An open discussion in studies of intrinsic brain functional connectivity is the mitigation of head motion-related artifacts, particularly in the presence of peculiar symptomatology such as in Parkinson’s disease (PD). Previous studies show that Independent Component Analysis (ICA) denoising improves the reproducibility of functional connectivity findings by detecting sources of non-neural signals. However, there is still no consensus about which pre-processing pipeline should be applied in natural high motion populations such as PD, particularly in relation to novel functional network descriptions derived from dynamic connectivity analyses. In this study, we investigated how different pre-processing pipelines affect intrinsic brain connectivity metrics, both static and dynamic, derived from a group of young healthy controls (HC) and a group of PD participants.
A total of 20 HC and 20 PD subjects participated in this 3 T MRI study. Resting-state functional MRI images were used to test the effects of the pre-processing pipeline of static (sFC) and temporal-varying functional connectivity (dFC) estimations. Both MRI datasets were pre-processed using three different workflows differing in the motion correction approach: (i) standard motion realignment ( mc ); (ii) motion outlier detection and deweighting based on image intensity change estimations ( DVARS ) and (iii) ICA-based noise removal using reference noise features ( AROMA ). Furthermore, the PD dataset was also processed with a fourth method by applying an ICA-based denoising ( FIX ), previously trained on the HC group. sFC analysis was performed using Group ICA, by temporally concatenating different pre-processing types in pairs of different runs. Two types of dFC analyses were considered: innovation-driven co-activation patterns (iCAPs) and co-activation patterns (CAPs). CAPs allow dFC estimations that do not require the deconvolution of the hemodynamic response function and its derivative, thus potentially being less sensitive to head-motion related noise. We found that regardless of substantial head motion differences in the two groups, sFC results were consistent across denoising strategies. Conversely, dFC was extremely sensitive to denoising strategies, particularly for the PD group with the transient-based dFC analyses. Indeed, the use of the peak-based dFC framework enables the detection of time-varying networks but in a way that is highly dependent on the motion correction pipeline. In conclusion, we show that dynamic functional network representations are highly sensitive to both head motion and to fMRI denoising methods. These findings stress the importance of considering and reporting these experimental aspects to help with the reproducibility and interpretation of different studies. Future work is needed to further investigate transient-based dFC strategies that are more robust to head motion.
### Competing Interest Statement
The authors have declared no competing interest.
更多查看译文
关键词
Brain Connectivity,Neuroimaging Data Analysis,Brain Network Development,Functional Connectivity,Neuroimaging
AI 理解论文
溯源树
样例
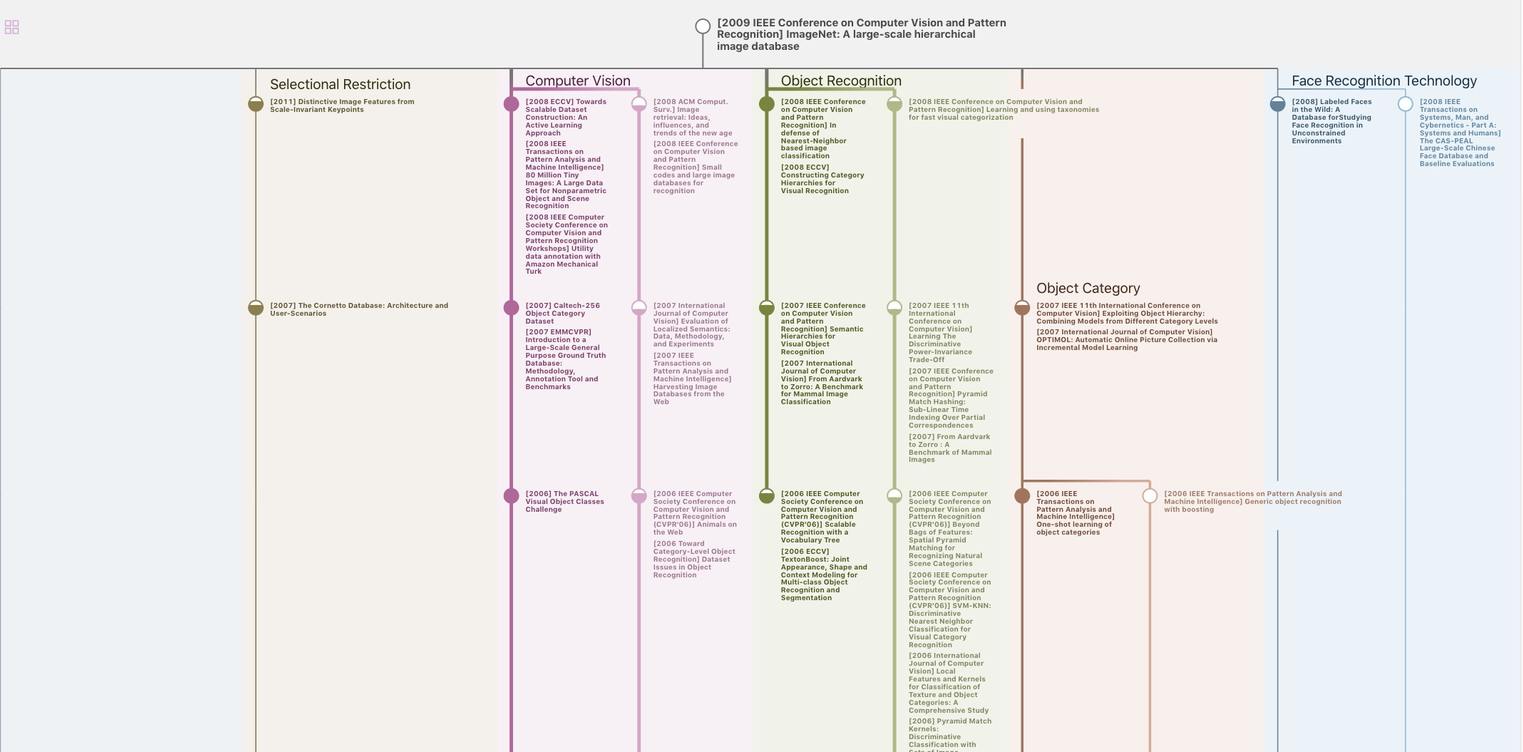
生成溯源树,研究论文发展脉络
Chat Paper
正在生成论文摘要