Surface Soil Moisture Retrieval on Qinghai-Tibetan Plateau Using Sentinel-1 Synthetic Aperture Radar Data and Machine Learning Algorithms.
Remote. Sens.(2023)
摘要
Soil moisture is a key factor in the water and heat exchange and energy transformation of the ecological systems and is of critical importance to the accurate obtainment of the soil moisture content for supervising water resources and protecting regional and global eco environments. In this study, we selected the soil moisture monitoring networks of Naqu, Maqu, and Tianjun on the Qinghai–Tibetan Plateau as the research areas, and we established a database of surface microwave scattering with the AIEM (advanced integral equation model) and the mathematical expressions for the backscattering coefficient, soil moisture, and surface roughness of the VV and VH polarizations. We used the soil moisture retrieval models of the backpropagation neural network (BPNN), support vector machine (SVM), K-nearest neighbors (KNN), and random forest (RF) empirical and machine learning algorithms for the ascending and descending orbits using Sentinel-1 and measurement data, and we also validated the accuracies of the retrieval model in the research areas. According to the results, there is a substantial logarithmic correlation among the backscattering coefficient, soil moisture, and combined roughness. Generally, we can use empirical models to estimate the soil moisture content, with an R² of 0.609, RMSE of 0.08, and MAE of 0.064 for the ascending orbit model and an R² of 0.554, RMSE of 0.086, and MAE of 0.071 for the descending orbit model. The soil moisture contents are underestimated when the volumetric water content is high. The soil moisture retrieval accuracy is improved with machine learning algorithms compared to the empirical model, and the performance of the RF algorithm is superior to those of the other machine learning algorithms. The RF algorithm also achieved satisfactory performances for the Maqu and Tianjun networks. The accuracies of the inversion models for the ascending orbit in the three soil moisture monitoring networks were better than those for the descending orbit.
更多查看译文
关键词
soil moisture,AIEM,machine learning algorithms,Sentinel-1,Qinghai-Tibetan Plateau
AI 理解论文
溯源树
样例
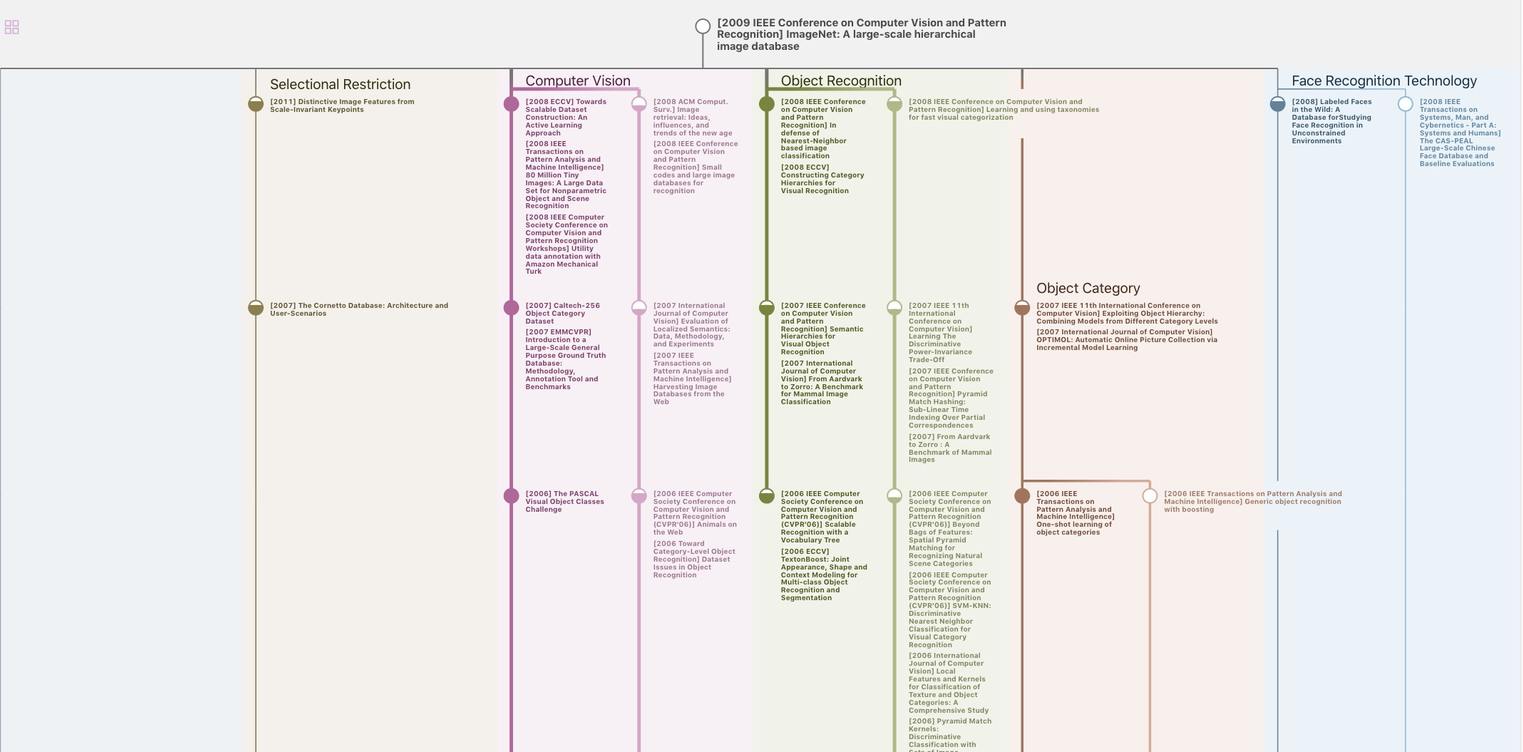
生成溯源树,研究论文发展脉络
Chat Paper
正在生成论文摘要