Gaussian Mixture with Max Expectation Guide for Stacked Architecture of Denoising Autoencoder and DRBM for Medical Chest Scans and Disease Identification
Electronics(2023)
摘要
Artificial intelligence (AI), in particular deep learning, has proven to be efficient in medical diagnosis. This paper introduces a new hybrid deep learning model for pneumonia diagnosis based on chest CT scans. At the core of the model, a Gaussian mixture is combined with the expectation-maximization algorithm (EMGMM) to extract the regions of interest (ROI), while a convolutional denoising autoencoder (DAE) and deep restricted Boltzmann machine (DRBM) are combined for the classification. In order to prevent the model from learning trivial solutions, stochastic noises were added as an input to the unsupervised learning phase. The dataset used in this work is a publicly available dataset of chest X-rays for pneumonia on the Kaggle website; it contains 5856 images with 1583 normal cases and 4273 pneumonia cases, with an imbalance ratio (IR) of 0.46. Several operations including zooming, flipping, shifting and rotation were used in the augmentation phase to balance the data distribution across the different classes, which led to enhancing the IR value to 0.028. The computational analysis of the results show that the proposed model is promising as it provides an average accuracy value of 98.63%, sensitivity value of 96.5%, and specificity value of 94.8%.
更多查看译文
关键词
deep learning,pneumonia prediction,Gaussian mixture,convolution autoencoder,Boltzmann machine
AI 理解论文
溯源树
样例
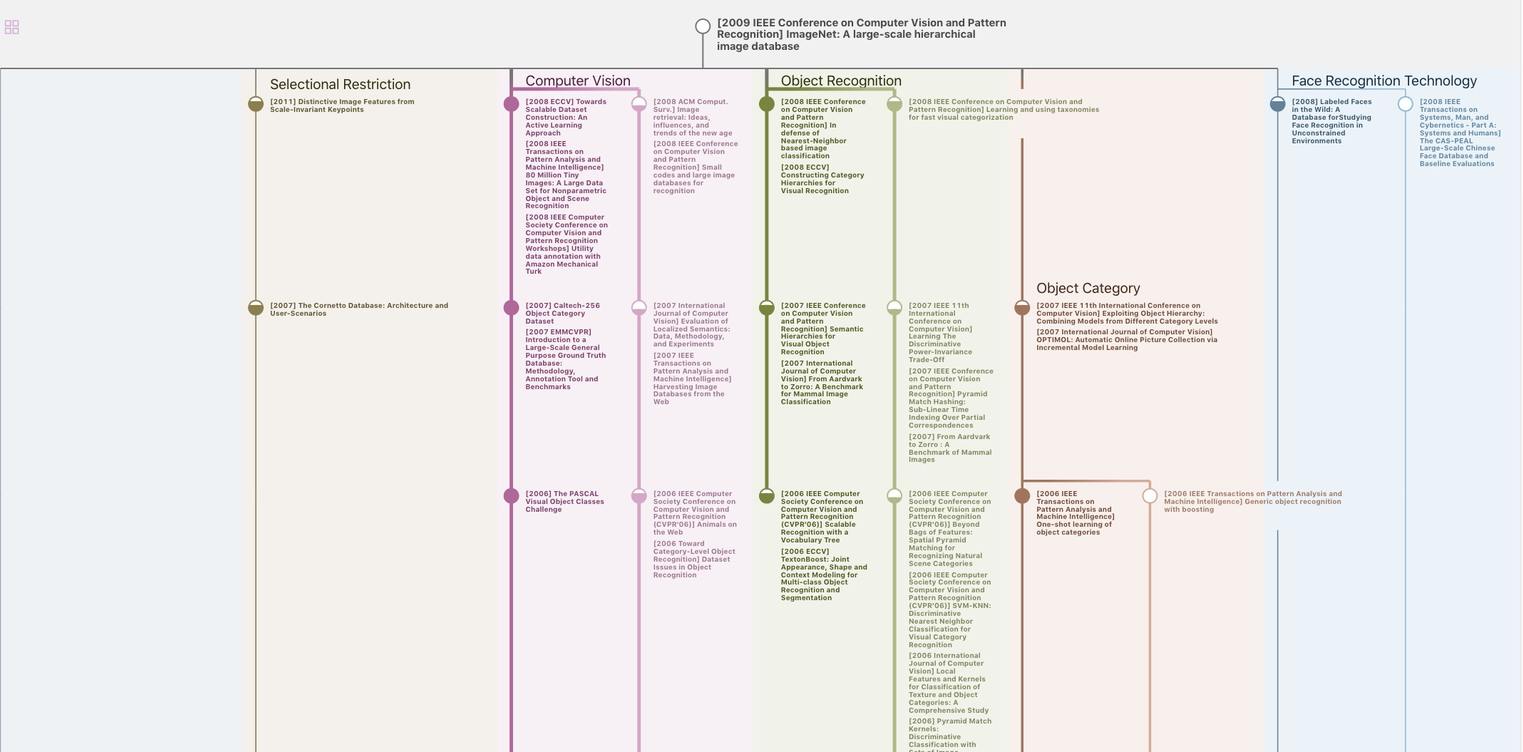
生成溯源树,研究论文发展脉络
Chat Paper
正在生成论文摘要