Modeling multi-regional temporal correlation with gated recurrent unit and multiple linear regression for urban traffic flow prediction
Knowledge-Based Systems(2023)
摘要
Urban traffic flow prediction has received much attention in the past few years, especially after the availability of huge traffic data. In addition, the efficacy of some existing traffic flow techniques heavily depends on some influential external factors (like weather, geographical information, point-of-interests (POIs), or road information). However, exploitable data containing such auxiliary information are extremely limited. One primary reason is that some rising cities lack the resources necessary for collecting such data. Therefore, it is difficult to pick an effective method that can accurately predict the traffic state when the data is limited. Due to that, this paper proposes a framework for achieving accurate predictions based on four data characteristics and the temporal correlation of neighbors without using additional influential factors. Specifically, we first propose a novel Multi-Region Correlation (MRC) method to make regions cooperate and share their traffic flow and patterns. Then, a deep recurrent network is used to capture the neighbors’ temporal correlation by integrating their traffic history that flow among regions and their neighbors. When analyzing the traffic data history, we model four data characteristics (seasonality, trend, residual, and cyclic). And lastly, we employ a Multiple Linear Regression Unit (MLRU) to predict the future traffic directly from the neighbors’ traffic. We conducted extensive experiments on two real-world datasets collected from two major cities in China, Chengdu and Xi’an. The results demonstrate that the proposed model can achieve superior performance compared to many existing models in terms of accuracy and efficiency.
更多查看译文
关键词
Data fusion,Temporal correlation,Traffic flow prediction,Time series,Multiple linear regression
AI 理解论文
溯源树
样例
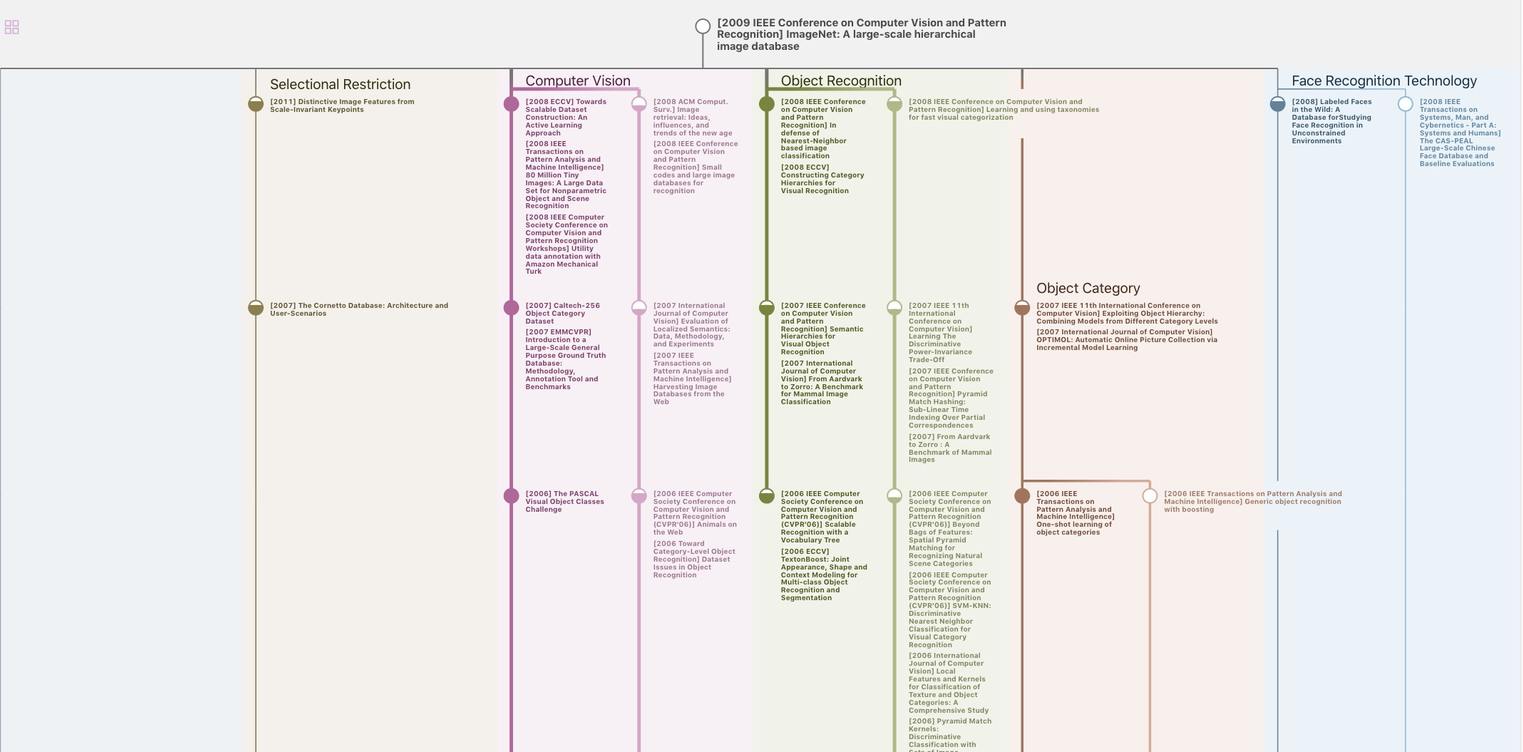
生成溯源树,研究论文发展脉络
Chat Paper
正在生成论文摘要