Inference of genetic networks using random forests: Performance improvement using a new variable importance measure
Chem-Bio Informatics Journal(2022)
摘要
Among the various methods so far proposed for genetic network inference, this study focuses on the random-forest-based methods. Confidence values are assigned to all of the candidate regulations when taking the random-forest-based approach. To our knowledge, all of the random-forest-based methods make the assignments using the standard variable importance measure defined in tree-based machine learning techniques. Therefore, the sum of the confidence values of the candidate regulations of a certain gene from the other genes, that are computed from a single random forest, is always restricted to a value of almost 1. We think that this feature is inconvenient for the genetic network inference that requires to compare the confidence values computed from multiple random forests. In this study we therefore propose an alternative measure, what we call ?the random-input variable importance measure," and design a new inference method that uses the proposed measure in place of the standard measure in the existing random-forest-based inference method. We show, through numerical experiments, that the use of the random-input variable importance measure improves the performance of the existing random-forest-based inference method by as much as 45.5% with respect to the area under the recall-precision curve (AURPC).
更多查看译文
关键词
random forestsperformance,genetic networks
AI 理解论文
溯源树
样例
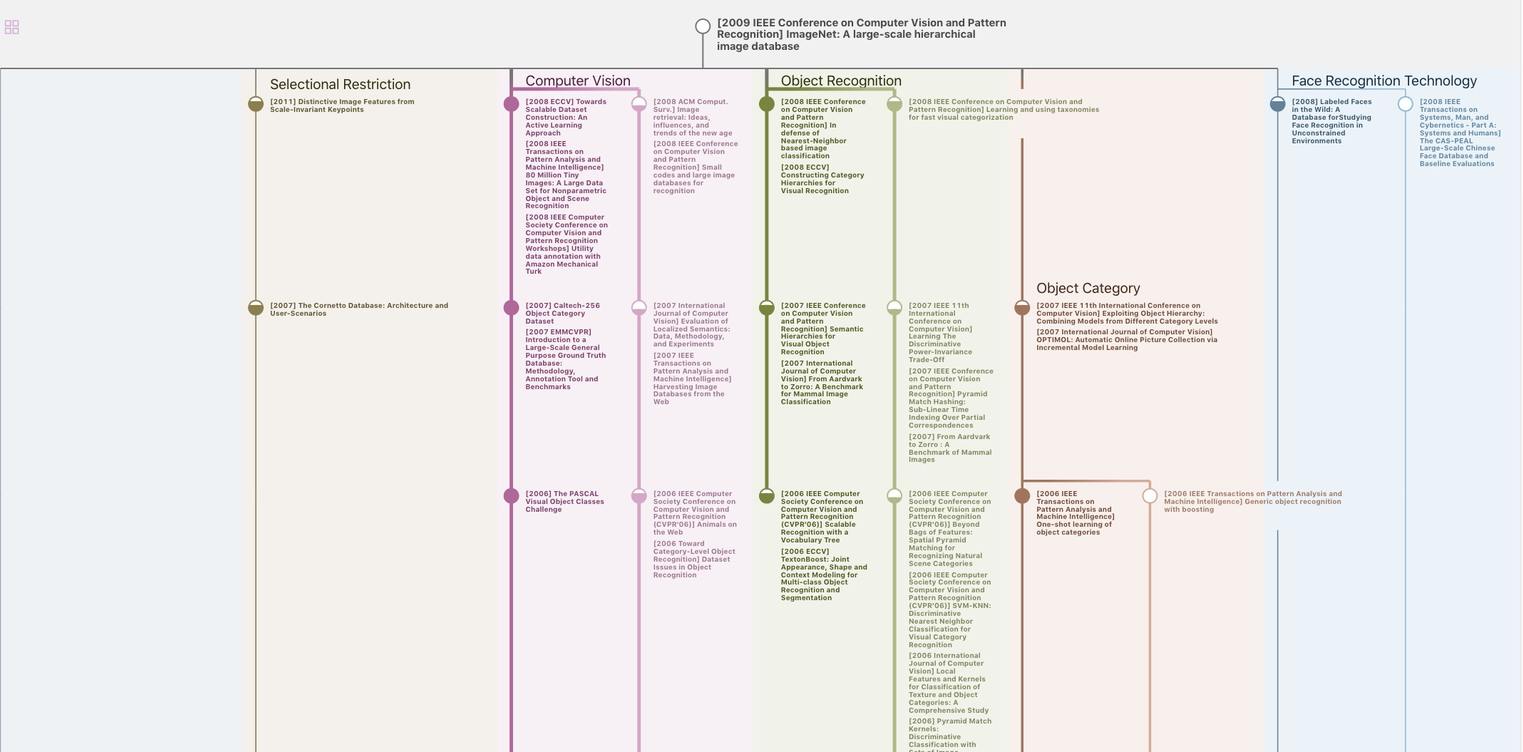
生成溯源树,研究论文发展脉络
Chat Paper
正在生成论文摘要