Real-time and Accurate Detection of Citrus in Complex Scenes Based on HPL-YOLOv4.
Computers and electronics in agriculture(2023)
摘要
This paper improves the citrus-fruit detection accuracy for picking robot in complex scenes and ensures real-time picking operations by proposing a novel detection method called high-precision and lightweight YOLOv4 (HPL-YOLOv4). Specifically, the lightweight feature extraction network GhostNet is applied as the backbone to enhance the citrus feature extraction. Then, a depthwise separable convolution and the Mish activation function are introduced into the Neck network to reduce the model's parameters. Furthermore, an efficient channel attention mechanism (ECA) is employed to enhance the multi-scale feature weights obtained by the backbone network, further improving the model's performance. Finally, the soft DIoU non-maximum suppression (DIo-U_NMS) algorithm optimizes the bounding boxes screening. The experimental evaluation highlights that for a 416 x 416 pixels image, the detection time of HPL-YOLOv4 is 18.2 ms, and the target detection speed is 55 FPS. Compared with YOLOv4, the average precision (AP) value of HPL-YOLOv4 is 98.21 %, and the precision rate is 93.45 %, increased by 9.41 % and 2.26 %, respectively. Moreover, the model size of HPL-YOLOv4 is reduced from 250.7 MB to 43.5 MB. Overall, HPL-YOLOv4 can accurately and quickly detect citrus fruits in complex growth environments, theoretically supporting the development of citrus-picking robots.
更多查看译文
关键词
Citrus Detection,YOLOv4,GhostNet,Picking Robot
AI 理解论文
溯源树
样例
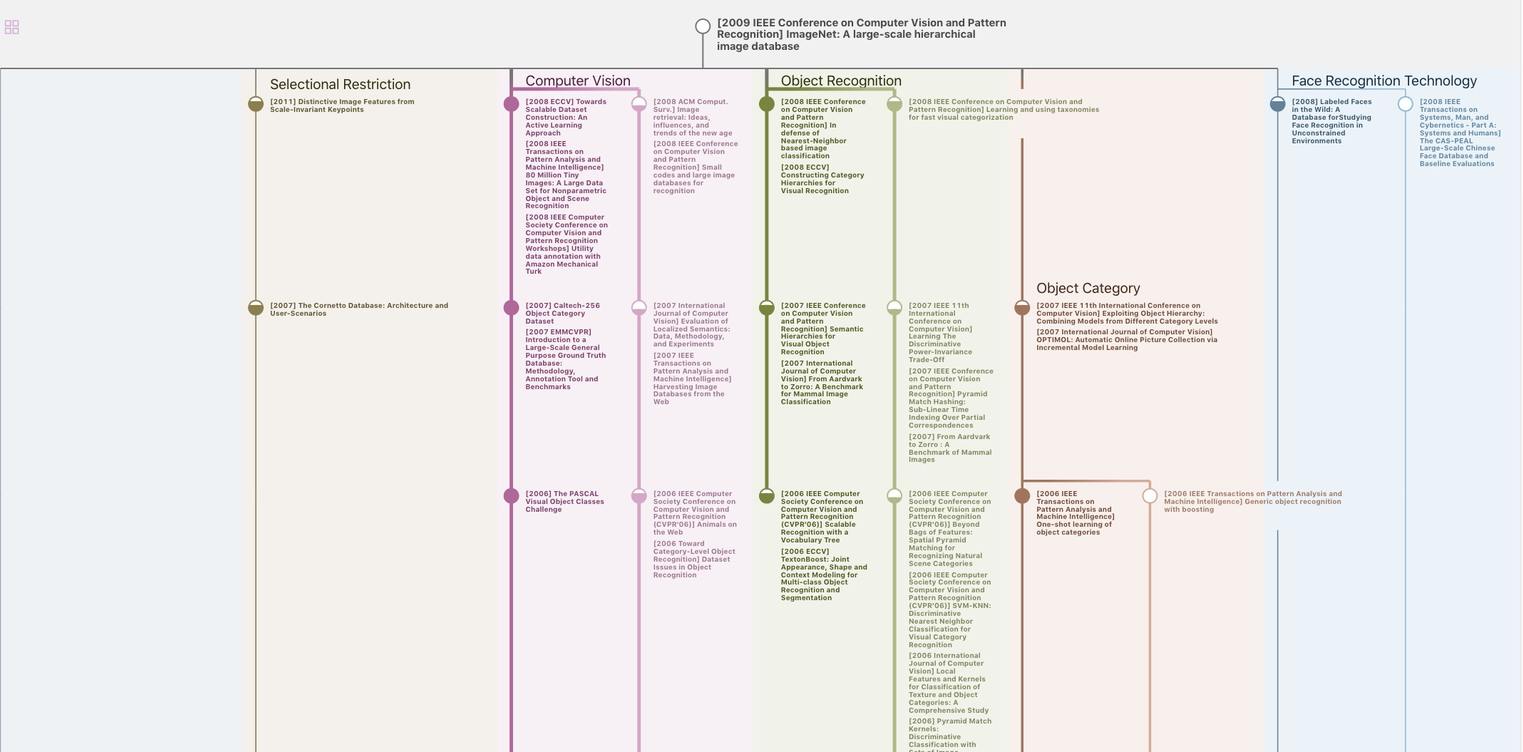
生成溯源树,研究论文发展脉络
Chat Paper
正在生成论文摘要