Encoding High-Level Control-Flow Construct Information for Process Outcome Prediction
2022 4th International Conference on Process Mining (ICPM)(2022)
摘要
Outcome-oriented predictive process monitoring aims at classifying a running process execution according to a given set of categorical outcomes, leveraging data on past process executions. Most previous studies employ Recurrent Neural Networks to encode the sequence of events, without taking the structure of the process into account. However, process executions typically involve complex control-flow constructs, like parallelism and loops. Different executions of these constructs can be recorded as different event sequences in the event log. This makes it challenging for a recurrent classifier to detect potential relations between a high-level control-flow construct and the prediction target. This is especially true in the presence of high variability in process executions and lack of data. In this paper, we propose a novel approach which encodes the control-flow construct each event belongs to. First, we exploit Local Process Model mining techniques to extract frequently occurring control-flow patterns from the event log. Then, we employ different encoding techniques to enrich an on-going process execution with information related to the extracted control-flow patterns. We tested the proposed method on nine real-life event logs. The obtained results show consistent improvements in the prediction performance.
更多查看译文
关键词
Predictive Process Monitoring,Process Outcome Prediction,Local Process Models
AI 理解论文
溯源树
样例
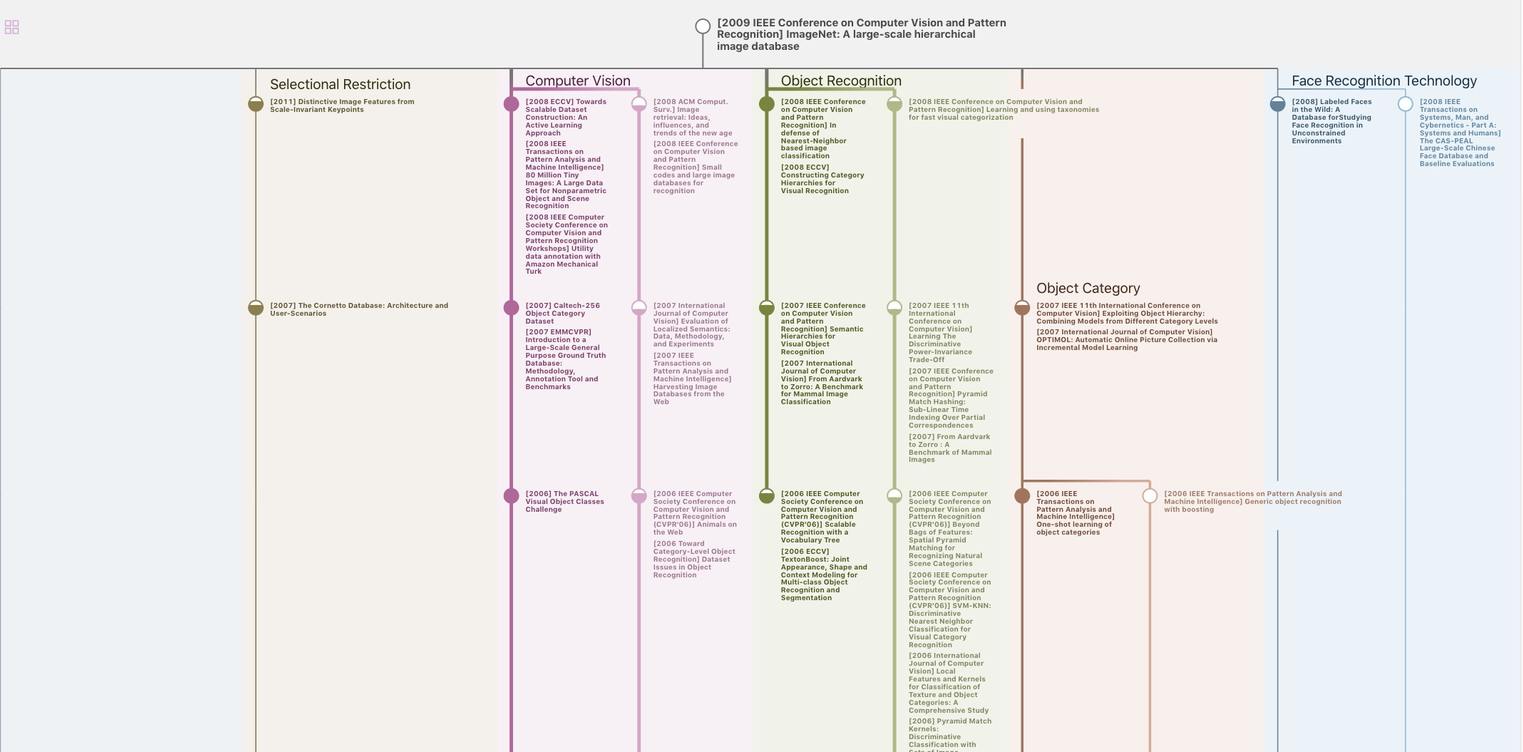
生成溯源树,研究论文发展脉络
Chat Paper
正在生成论文摘要