CloudNet: A Deep Learning Approach for Mitigating Occlusions in Landsat-8 Imagery Using Data Coalescence.
2022 IEEE 18TH INTERNATIONAL CONFERENCE ON E-SCIENCE (ESCIENCE 2022)(2022)
摘要
Multi-spectral satellite images that remotely sense the Earth's surface at regular intervals are often contaminated due to occlusion by clouds. Remote sensing imagery captured via satellites, drones, and aircraft has successfully influenced a wide range of fields such as monitoring vegetation health, tracking droughts, and weather forecasting, among others. Researchers studying the Earth's surface are often hindered while gathering reliable observations due to contaminated reflectance values that are sensitive to thin, thick, and cirrus clouds, as well as their shadows. In this study, we propose a deep learning network architecture, CloudNet, to alleviate cloud-occluded remote sensing imagery captured by Landsat-8 satellite for both visible and non-visible spectral bands. We propose a deep neural network model trained on a distributed storage cluster that leverages historical trends within Landsat-8 imagery while complementing this analysis with high-resolution Sentinel-2 imagery. Our empirical benchmarks profile the efficiency of the CloudNet model with a range of cloud-occluded pixels in the input image. We further compare our CloudNet's performance with state-of-the-art deep learning approaches such as SpAGAN and Resnet. We propose a novel method, dynamic hierarchical transfer learning, to reduce computational resource requirements while training the model to achieve the desired accuracy. Our model regenerates features of cloudy images with a high PSNR accuracy of 34.28 dB.
更多查看译文
关键词
remote sensing,deep learning,cloud removal,convolution network
AI 理解论文
溯源树
样例
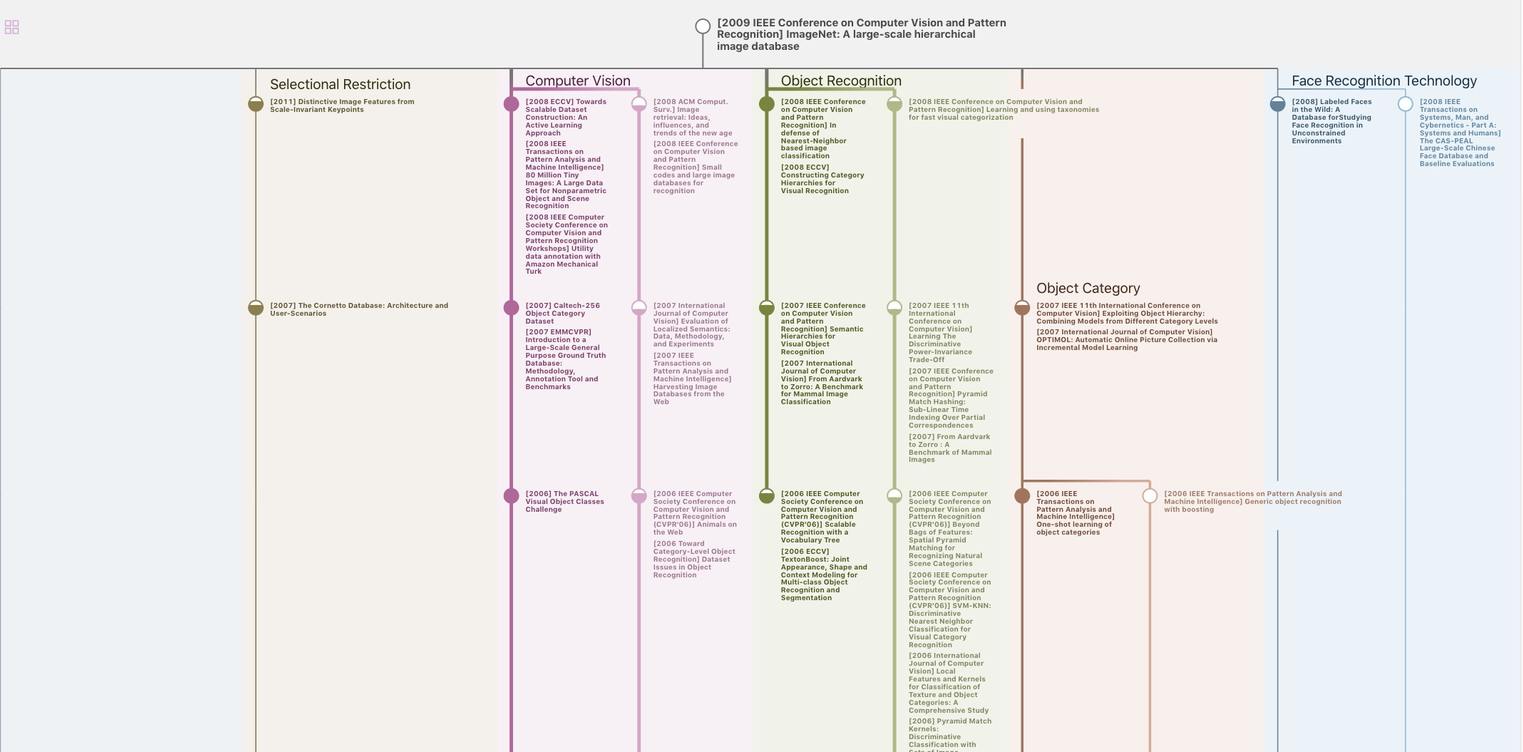
生成溯源树,研究论文发展脉络
Chat Paper
正在生成论文摘要