Automated machine learning-based radiomics analysis versus deep learning-based classification for thyroid nodule on ultrasound images: a multi-center study
2022 IEEE 22nd International Conference on Bioinformatics and Bioengineering (BIBE)(2022)
摘要
Often, the characteristics of thyroid nodules need to be determined by fine needle aspiration (FNA) biopsy. The increasing applications of machine learning and deep learning algorithms provide alternative noninvasive methods to study thyroid nodules on ultrasound images. Many studies examined the feasibility of convolutional neural networks or radiomics feature extraction to analyze the characteristics of thyroid nodules. In this study, we built an automated radiomics analysis system by combining thyroid segmentation via U-Net and radiomics feature extraction. Our proposed machine learning-based automated radiomics analysis was compared to a deep learning-based convolutional neural network method in a two-center thyroid nodule classification task. It is shown that the automated radiomics analysis can accurately segment thyroid nodules to facilitate clinical diagnosis by achieving dice scores of 0.77 and 0.74 on internal and external sets respectively. In addition, the proposed automated radiomics analysis approach can improve sensitivity, negative predictive value (NPV) and positive predictive value (PPV) by 41.2%, 3.5% and 7.5% respectively, while reducing the false negative rate by 41.1%.
更多查看译文
关键词
radiomics,thyroid nodule,deep learning,ultrasound,radimagenet
AI 理解论文
溯源树
样例
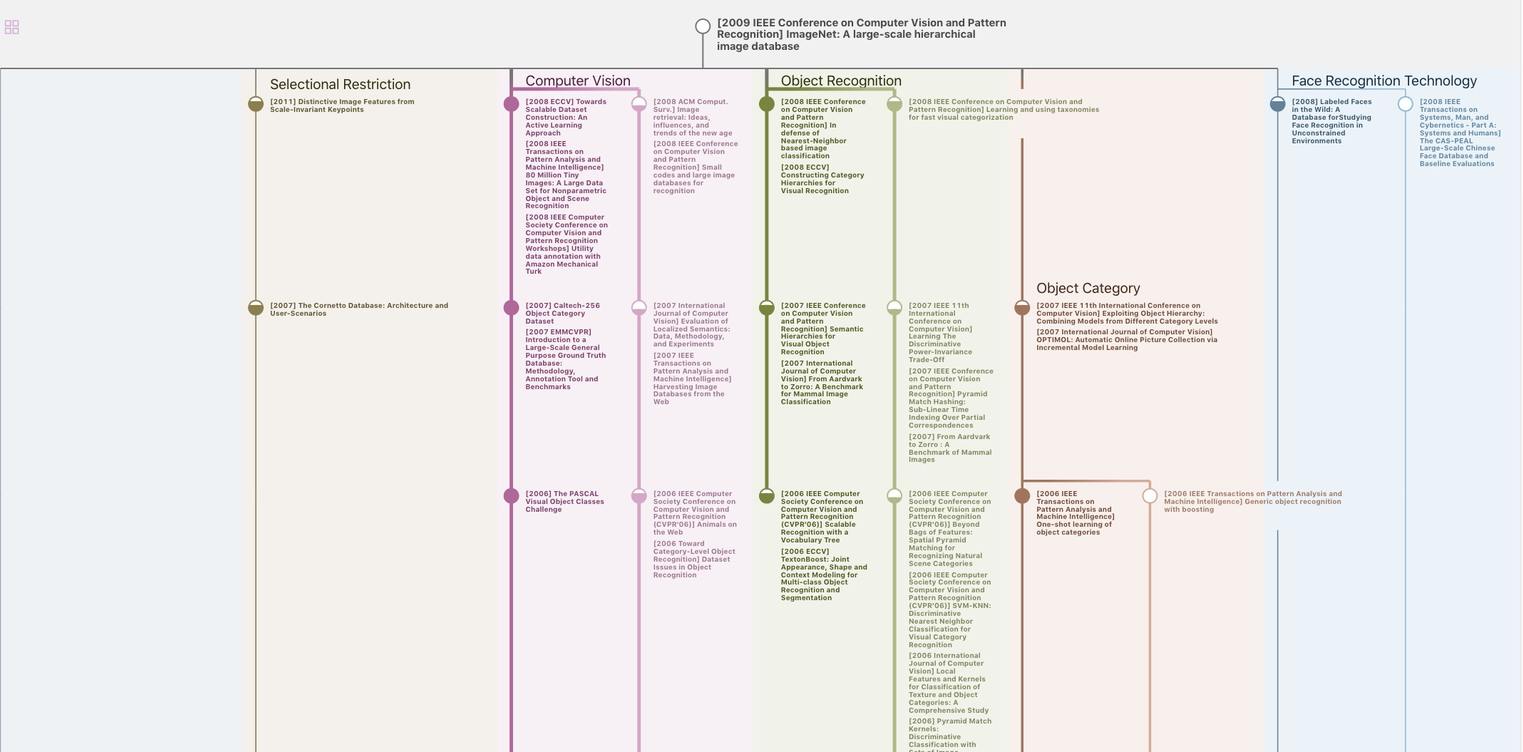
生成溯源树,研究论文发展脉络
Chat Paper
正在生成论文摘要