Deep-Learning-Based Step Detection and Step Length Estimation With a Handheld IMU
IEEE Sensors Journal(2022)
摘要
A popular approach to track the position of a user is to use an inertial measurement unit (IMU), which allows tracking a user with a pedestrian dead reckoning (PDR) system by estimating the length and heading of each step a user takes. Furthermore, a user’s steps together with their length can be used to detect anomalies in the gait due to, e.g., a gait disorder. To determine the length and/or heading of a step, we first need an algorithm that estimates the boundaries of a step, i.e., the start and end, in the IMU signals. In this article, we propose a deep-learning-based step detection algorithm that detects the start and end of a step using only data from a handheld accelerometer. In this algorithm, also, the relationship between the start and end of a step is used to improve performance. To evaluate the performance of the detector, in contrast to most previous works that only look at the total number of detected steps, we determine if the start and end of each step are detected at the correct instant. This resulted in a step detector with an f-score of 99.2% and 99.0% for, respectively, detecting the start and end of a step. After detecting the start and end of the steps, we use a long short-term memory (LSTM)/convolutional neural network (CNN)-based step length estimator, which resulted in a mean absolute error (mae) on the step length of 3.21 cm. Finally, we also determine the combined performance of the step detector and step length estimator, i.e., where the proposed step detector is used to extract the accelerometer fragments of a step instead of assuming that the boundaries are known. This resulted in an mae of 6.56 cm using the deep-learning-based step length estimator.
更多查看译文
关键词
Deep learning,inertial measurement unit (IMU),pedestrian dead reckoning (PDR),step detection,step length
AI 理解论文
溯源树
样例
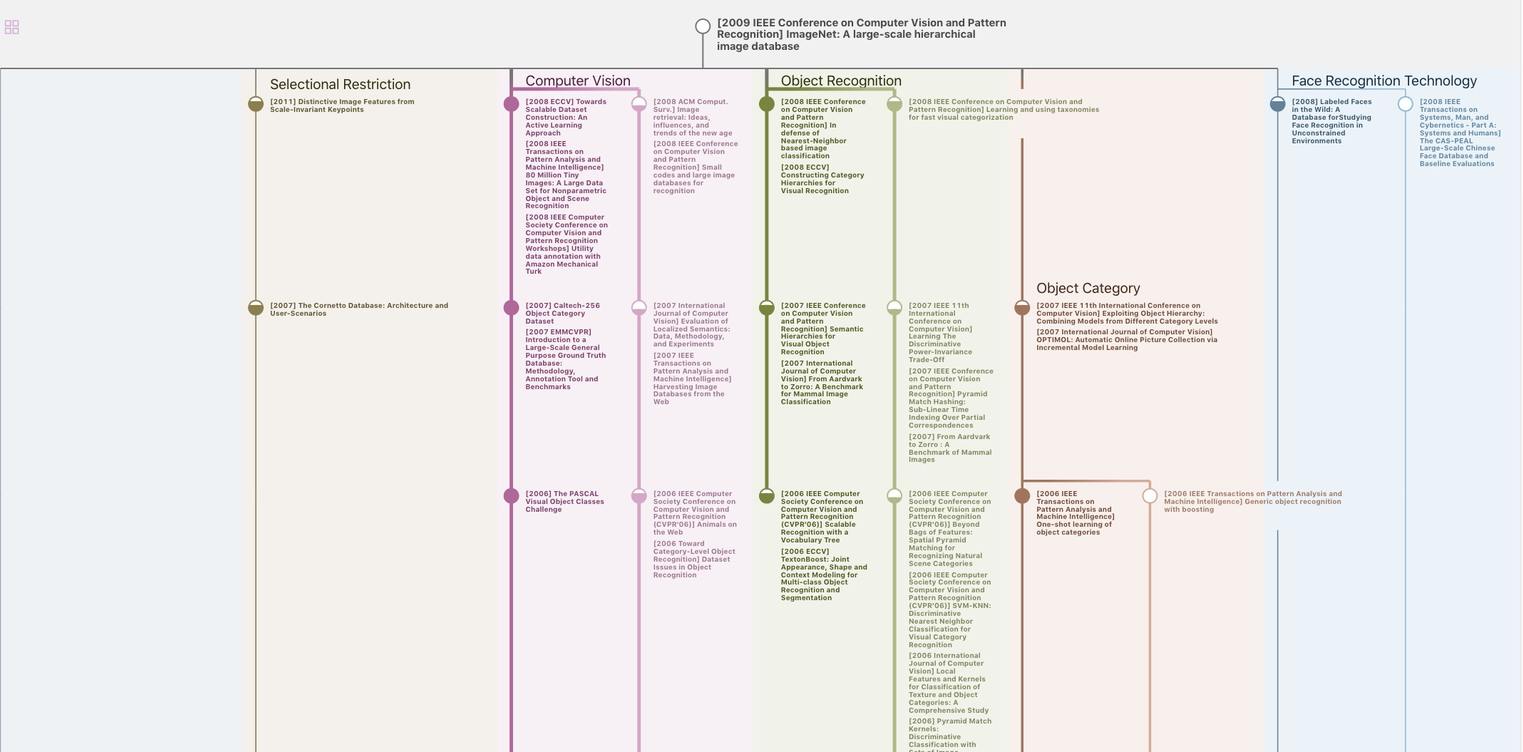
生成溯源树,研究论文发展脉络
Chat Paper
正在生成论文摘要