GEDI: GEnerative and DIscriminative Training for Self-Supervised Learning
arxiv(2023)
摘要
Self-supervised learning is a popular and powerful method for utilizing large amounts of unlabeled data, for which a wide variety of training objectives have been proposed in the literature. In this study, we perform a Bayesian analysis of state-of-the-art self-supervised learning objectives and propose a unified formulation based on likelihood learning. Our analysis suggests a simple method for integrating self-supervised learning with generative models, allowing for the joint training of these two seemingly distinct approaches. We refer to this combined framework as GEDI, which stands for GEnerative and DIscriminative training. Additionally, we demonstrate an instantiation of the GEDI framework by integrating an energy-based model with a cluster-based self-supervised learning model. Through experiments on synthetic and real-world data, including SVHN, CIFAR10, and CIFAR100, we show that GEDI outperforms existing self-supervised learning strategies in terms of clustering performance by a wide margin. We also demonstrate that GEDI can be integrated into a neural-symbolic framework to address tasks in the small data regime, where it can use logical constraints to further improve clustering and classification performance.
更多查看译文
关键词
discriminative training,learning,generative,self-supervised
AI 理解论文
溯源树
样例
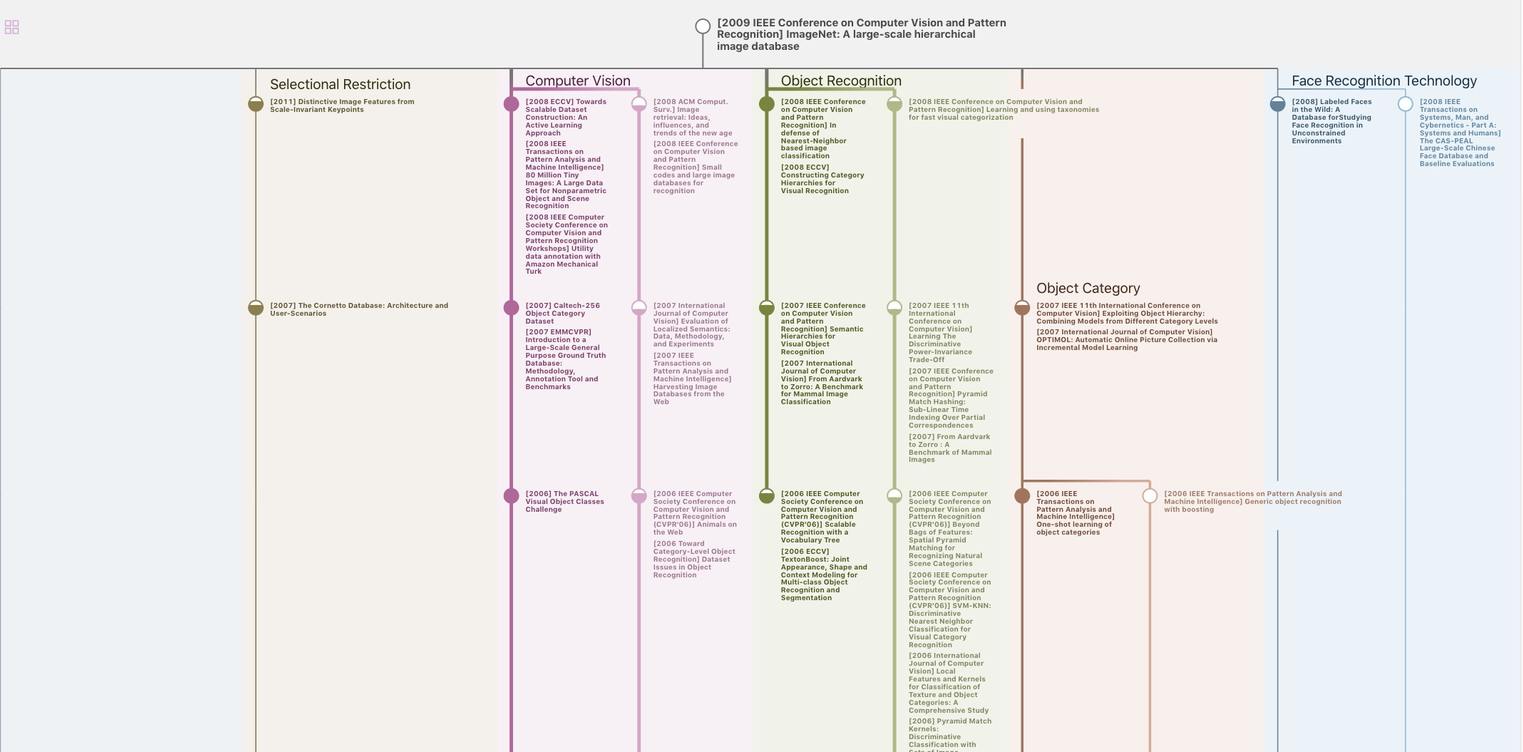
生成溯源树,研究论文发展脉络
Chat Paper
正在生成论文摘要