Dynamic Online Trajectory Planning for a UAV-Enabled Data Collection System
IEEE Transactions on Vehicular Technology(2022)
摘要
Due to the maneuverability and flexibility of unmanned aerial vehicles (UAVs), the UAV-enabled data collection systems for wireless sensor networks (WSN) have received widespread attention. However, the state-of-the-art UAV trajectory designs mainly focus on static environments, which are not applicable in the practical scenarios considered in this work, e.g., mobile nodes, decommissioning of existing nodes, and new emergency nodes. This article proposes a two-level deep reinforcement learning (DRL) framework to solve this challenge. The first-level deep neural network (DNN) is applied to model the dynamic changing environment. In the second level, we employ a deep Q-learning network to plan a trajectory online according to the environment features from the first level DNN. Besides, online trajectory planning is performed by a low-power UAV edge computing platform. To enable online planning on the power-constraint UAV edge-computing platform, all networks adopt a lightweight low-complexity optimization design. According to simulation results, the proposed system achieves higher data acquisition success rates when compared to existing state-of-the-art methods. We also perform field tests on the proposed UAV edge computing platform, which also demonstrates high data acquisition performance.
更多查看译文
关键词
Unmanned aerial vehicle (UAV),online trajectory planning,deep reinforcement learning (DRL)
AI 理解论文
溯源树
样例
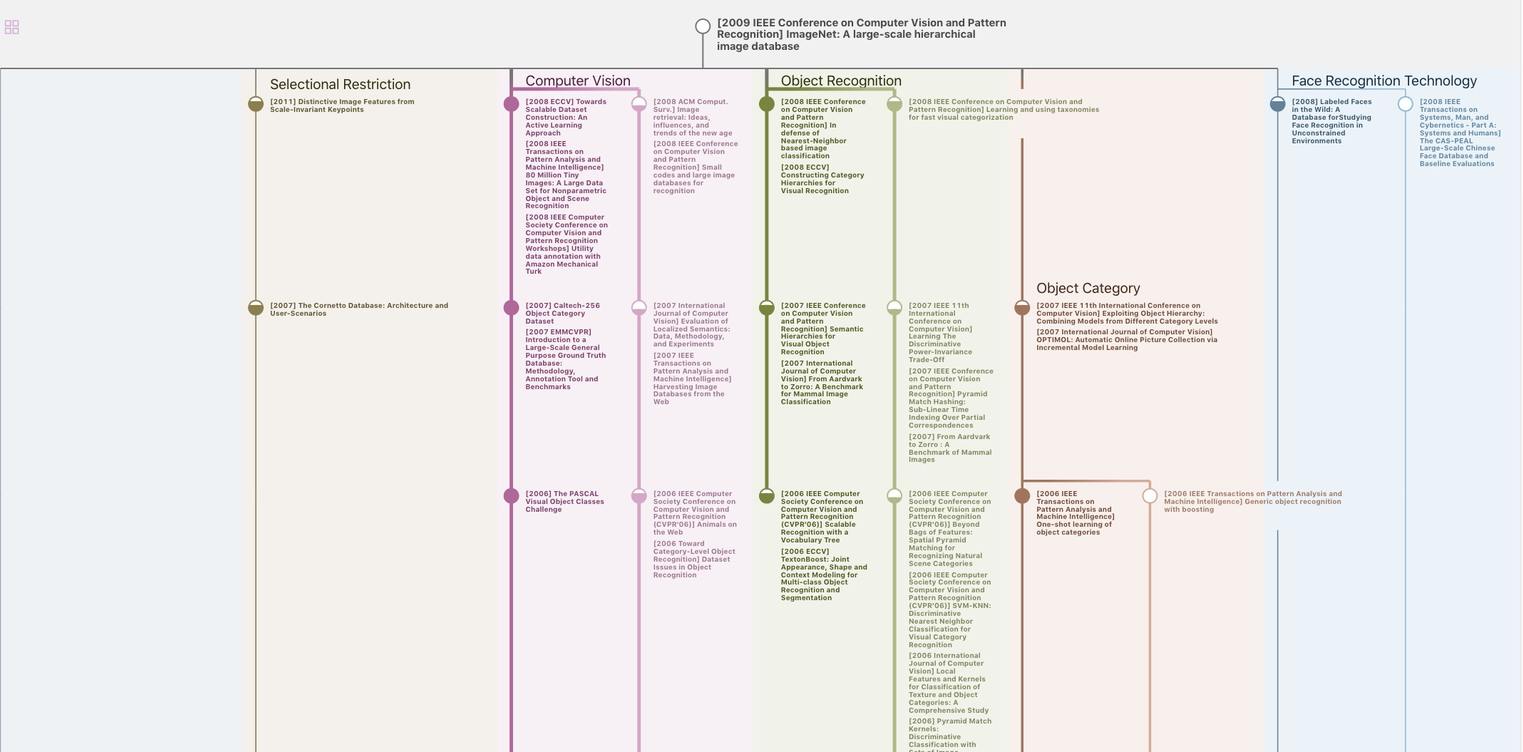
生成溯源树,研究论文发展脉络
Chat Paper
正在生成论文摘要