Evaluating Emergent Coordination in Multi-Agent Task Allocation Through Causal Inference and Sub-Team Identification
IEEE Robotics and Automation Letters(2023)
摘要
Coordination in multi-agent systems is a vital component in teaming effectiveness. In dynamically changing situations, agent decisions depict emergent coordination strategies from following pre-defined rules to exploiting incentive-driven policies. While multi-agent reinforcement learning shapes team behaviors from experience, interpreting learned coordination strategies offers benefits in understanding complex agent dynamics and further improvement in developing adaptive strategies for evolving and unexpected situations. In this work, we develop an approach to quantitatively measure team coordination by collecting decision time series data, detecting causality between agents, and identifying statistically high coordinated sub-teams in decentralized multi-agent task allocation operations. We focus on multi-agent systems with homogeneous agents and homogeneous tasks as the strategy formation is more ambiguous and challenging than heterogeneous teams with specialized capabilities. Emergent team coordination is then analyzed using rule-based and reinforcement learning-based strategies for task allocation in operations at different demand stages (stress) levels. We also investigate correlation vs. causation and agent over- or under-estimating demand levels.
更多查看译文
关键词
Multi-Robot Systems,Reinforcement Learning,Planning,Scheduling and Coordination,Task Planning
AI 理解论文
溯源树
样例
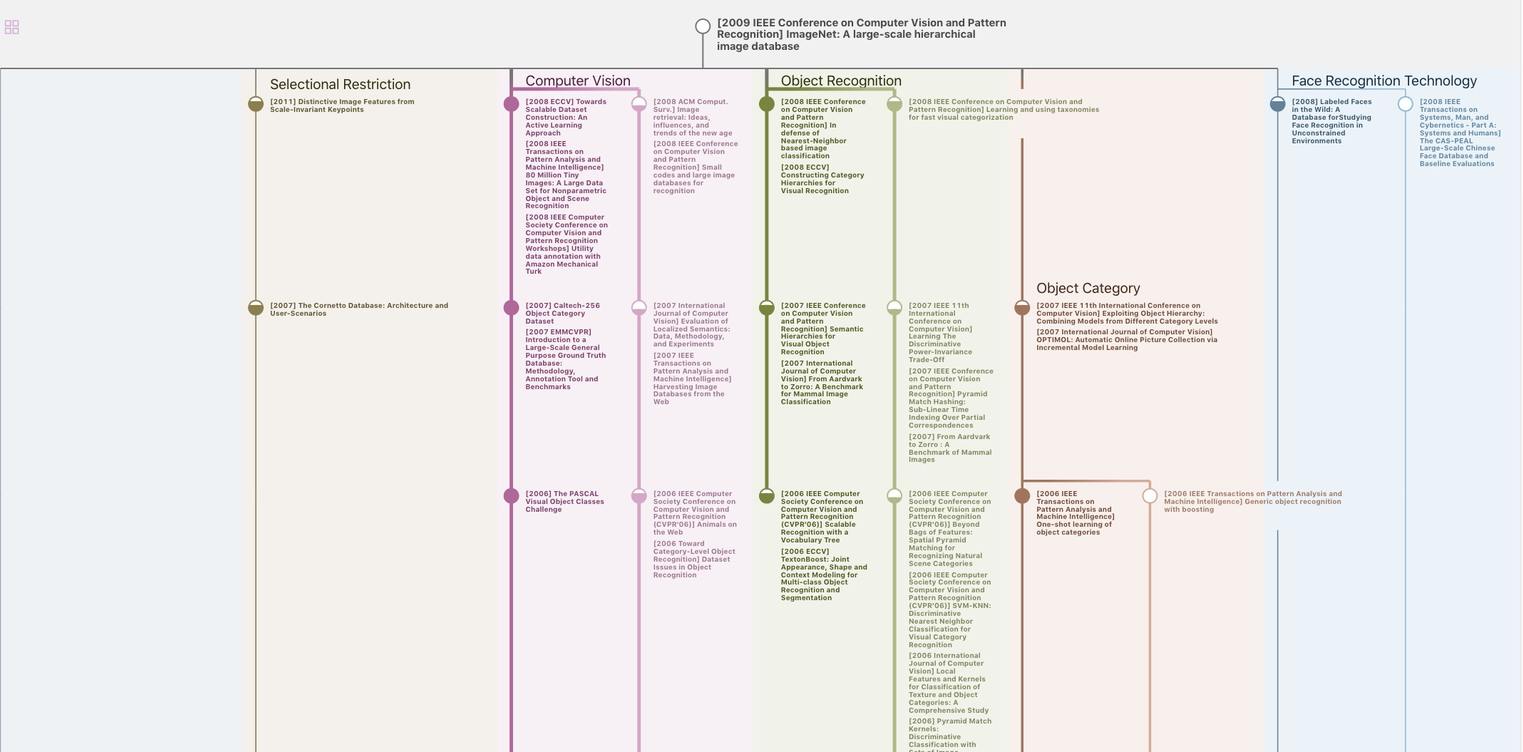
生成溯源树,研究论文发展脉络
Chat Paper
正在生成论文摘要