CGAN-Based Collaborative Intrusion Detection for UAV Networks: A Blockchain-Empowered Distributed Federated Learning Approach
IEEE Internet of Things Journal(2023)
摘要
Numerous resource-constrained Internet of Things (IoT) devices make the edge IoT consisting of unmanned aerial vehicles (UAVs) vulnerable to network intrusion. Therefore, it is critical to design an effective intrusion detection system (IDS). However, the differences in local data sets among UAVs show small samples and uneven distribution, further reducing the detection accuracy of network intrusion. This article proposes a conditional generative adversarial net (CGAN)-based collaborative intrusion detection algorithm with blockchain-empowered distributed federated learning to solve the above problems. This study introduces long short-term memory (LSTM) into the CGAN training to improve the effect of generative networks. Based on the feature extraction ability of LSTM networks, the generated data with CGAN are used as augmented data and applied in the detection and classification of intrusion data. Distributed federated learning with differential privacy ensures data security and privacy and allows collaborative training of CGAN models using multiple distributed data sets. Blockchain stores and shares the training models to ensure security when the global model’s aggregation and updating. The proposed method has good generalization ability, which can greatly improve the detection of intrusion data.
更多查看译文
关键词
Blockchain,conditional generative adversarial network (CGAN),distributed federated learning,intrusion detection system (IDS),long short-term memory (LSTM),unmanned aerial vehicle (UAV) network
AI 理解论文
溯源树
样例
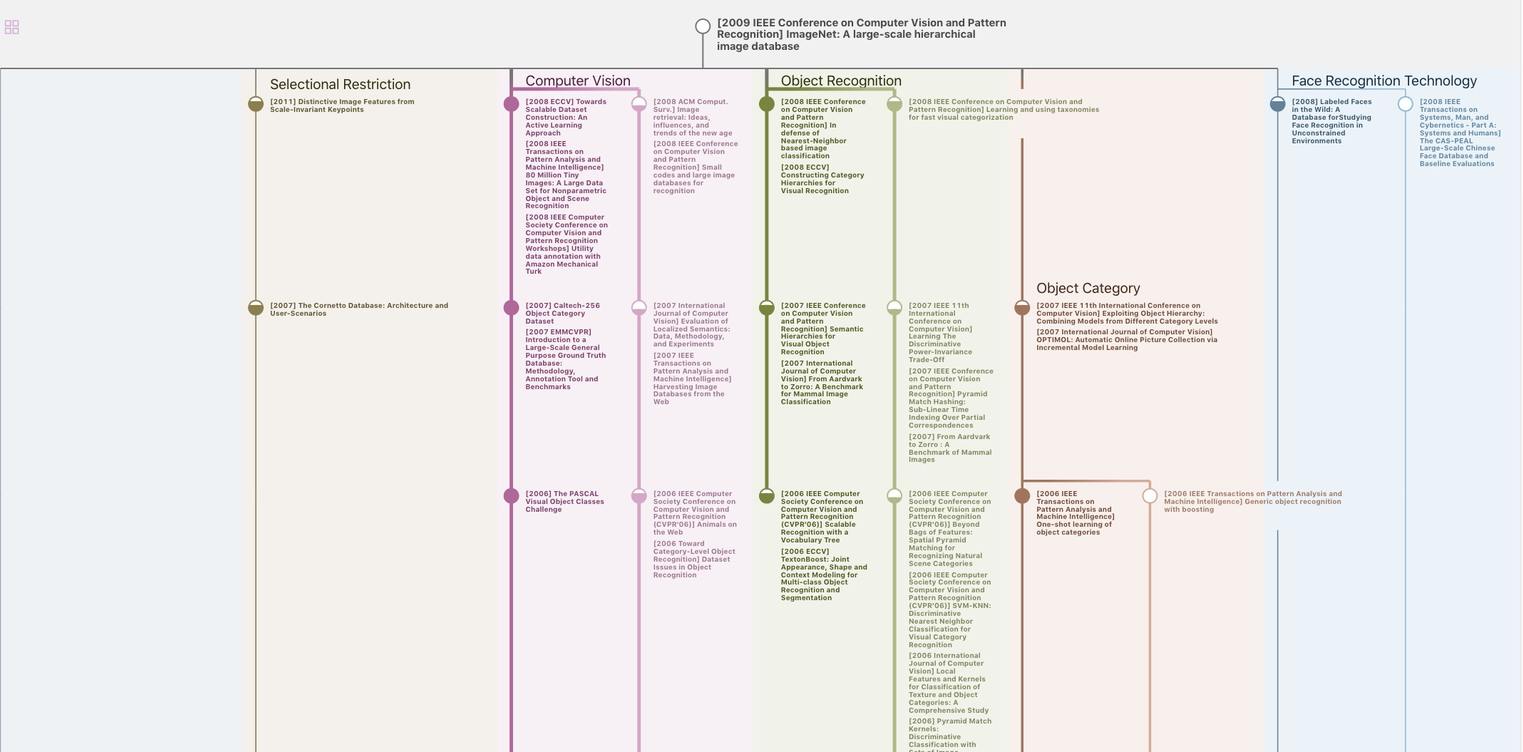
生成溯源树,研究论文发展脉络
Chat Paper
正在生成论文摘要